Improved Neural Radiance Fields Using Pseudo-depth and Fusion
CoRR(2023)
摘要
Since the advent of Neural Radiance Fields, novel view synthesis has received tremendous attention. The existing approach for the generalization of radiance field reconstruction primarily constructs an encoding volume from nearby source images as additional inputs. However, these approaches cannot efficiently encode the geometric information of real scenes with various scale objects/structures. In this work, we propose constructing multi-scale encoding volumes and providing multi-scale geometry information to NeRF models. To make the constructed volumes as close as possible to the surfaces of objects in the scene and the rendered depth more accurate, we propose to perform depth prediction and radiance field reconstruction simultaneously. The predicted depth map will be used to supervise the rendered depth, narrow the depth range, and guide points sampling. Finally, the geometric information contained in point volume features may be inaccurate due to occlusion, lighting, etc. To this end, we propose enhancing the point volume feature from depth-guided neighbor feature fusion. Experiments demonstrate the superior performance of our method in both novel view synthesis and dense geometry modeling without per-scene optimization.
更多查看译文
关键词
improved neural radiance fields,fusion,pseudo-depth
AI 理解论文
溯源树
样例
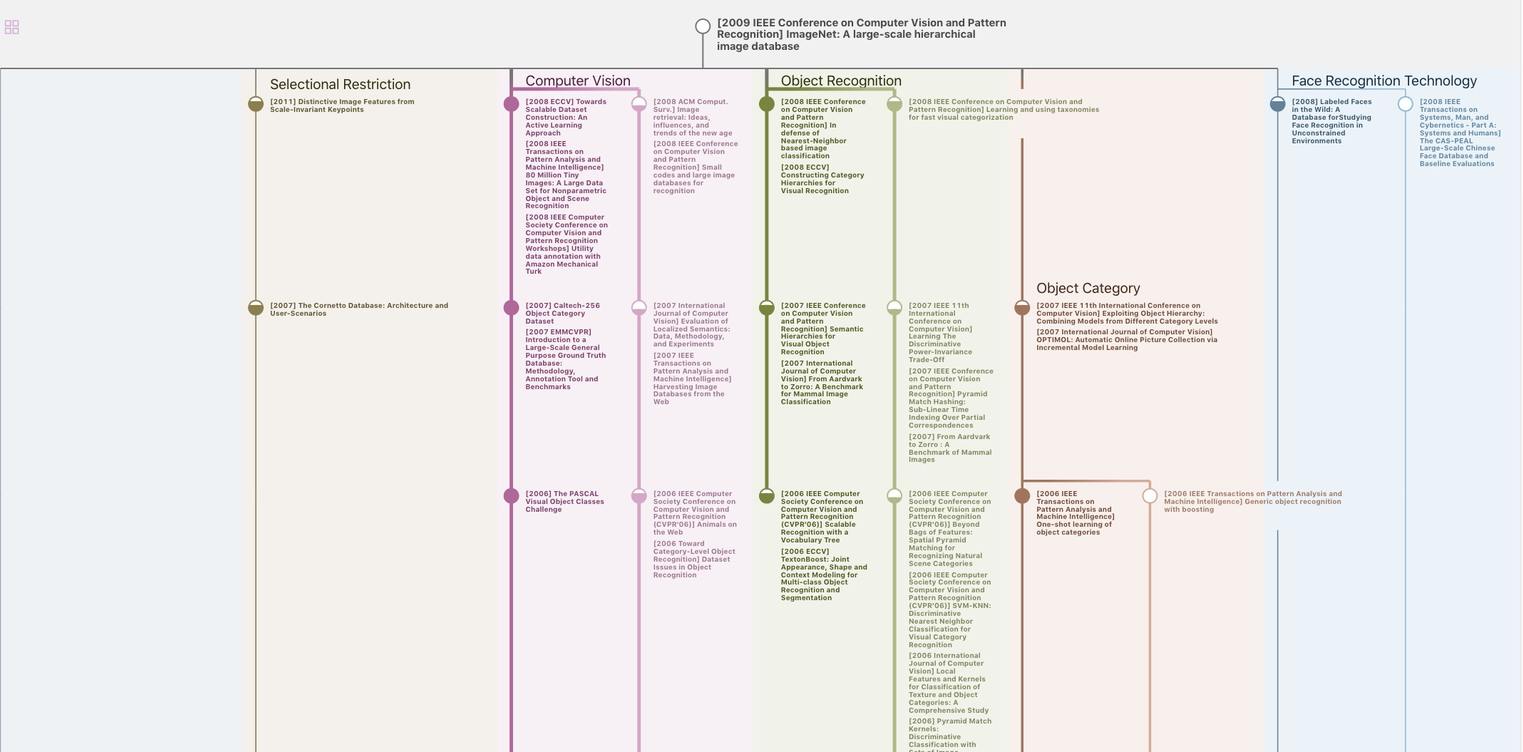
生成溯源树,研究论文发展脉络
Chat Paper
正在生成论文摘要