Modeling Reviews for Few-Shot Recommendation via Enhanced Prototypical Network
IEEE Transactions on Knowledge and Data Engineering(2023)
摘要
Although some existing models are proposed to exploit reviews for improving performance for recommender systems, few of them can handle the following issues led by the insufficient review data: (i) The regular training process does not exactly fit the scenario of preference prediction with few historical behaviors. (ii) Extracting informative and sufficient semantic features from limited review texts is a challenging work. To alleviate these issues, this paper proposes an enhanced prototypical network, FS-EPN, that leverages reviews for recommendation under the few-shot setting. FS-EPN consists of an attentional prototypical network being the basic architecture, a sentiment encoder and a memory collector cooperating to capture the extra sentimental and collaborative information from both user and item perspectives for semantic information supplement. We train FS-EPN under the meta-learning framework, which models the training process in the episodic manner to mimic the few-shot test environment. Extensive experiments conducted on six publicly available datasets demonstrate the superior capability of FS-EPN over several state-of-the-art models in few-shot recommendation.
更多查看译文
关键词
reviews,few-shot
AI 理解论文
溯源树
样例
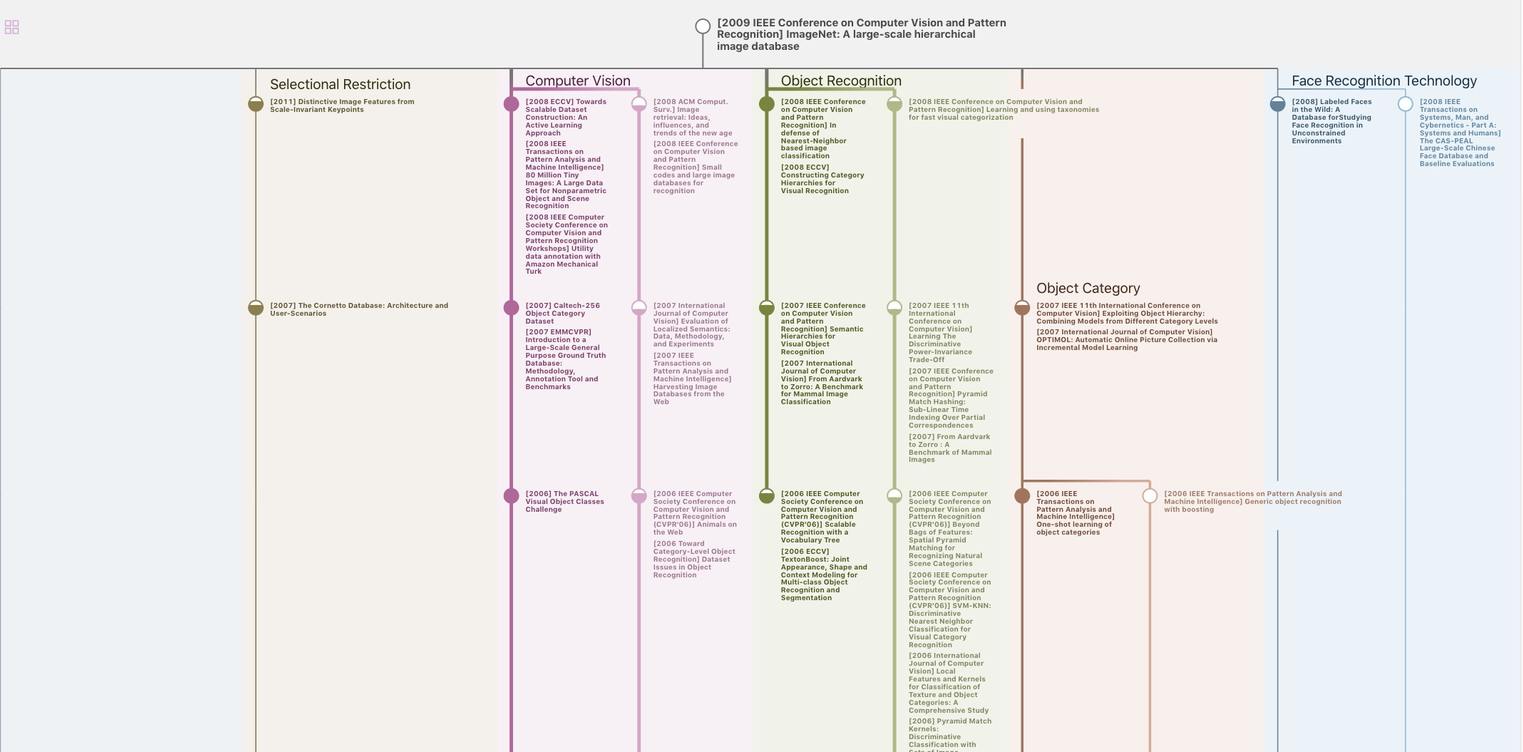
生成溯源树,研究论文发展脉络
Chat Paper
正在生成论文摘要