HSDN: A High-Order Structural Semantic Disentangled Neural Network
IEEE Transactions on Knowledge and Data Engineering(2023)
摘要
Graph disentangling is a new promising direction that can help us to discover the latent patterns in the data and understand the behaviors of a graph learning model. Despite the many efforts in disentangling representation learning, few works focus on disentangling the latent factors behind a graph. Most current foci are mainly on studying node-level semantics in the graphs. Compared with node-level, the structure-level view can provide a new interpretable and in-depth insight into graph data. The study of structure-level relations enables us to reveal the high-order structural semantics in the data. To explore the complex high-order structural semantics in the data, we propose the High-order Structural Semantic Disentangled Neural Network (HSDN) to model the graph structure units and disentangle structural semantics. It's the first attempt to hypergraph disentangled networks. Unlike prior methods that disentangle factor graphs based on pair-wise relations only, we introduce hyperedges on pair-wise graphs to model structure units and disentangle the complex high-order structural semantics between different structures. Extensive experiments demonstrate that HSDN achieves state-of-the-art performances in terms of both disentangling and downstream tasks.
更多查看译文
关键词
Disentangling, structural semantics, structure-level, hypergraph
AI 理解论文
溯源树
样例
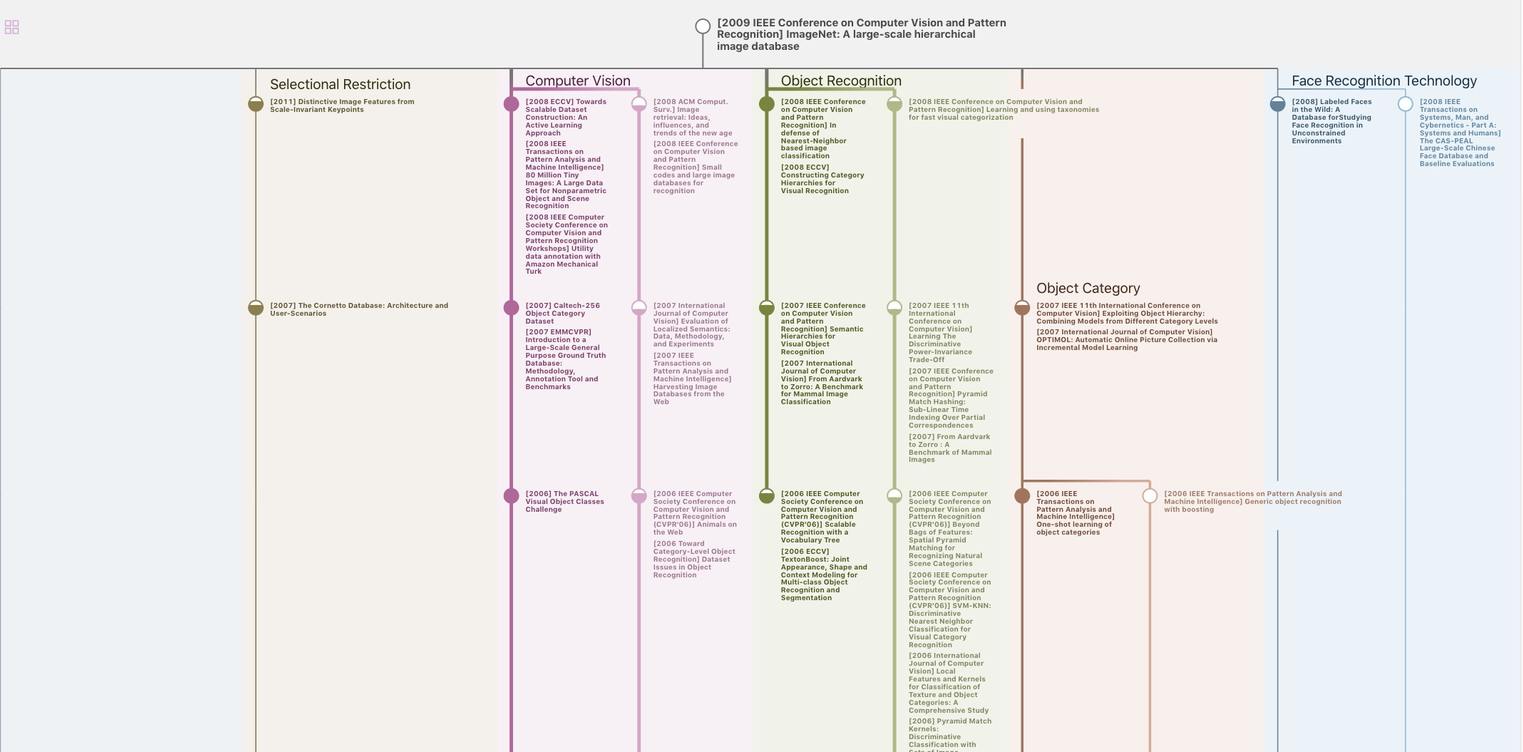
生成溯源树,研究论文发展脉络
Chat Paper
正在生成论文摘要