[Soil Pollution Prediction from Enterprise for Coking Industry in China Based on Machine Learning and Scenario Analysis].
PubMed(2023)
摘要
Owing to the lack of sequential monitoring data of soil pollutants in coking industry enterprises, it is hard to accurately predict their soil pollution. To predict the trend of soil pollution of coking industry enterprises in the future, a prediction model should be developed using machine learning based on the influencing factors. A total of 13 potential factors were selected from the enterprise characteristics, enterprise management level, pollutant characteristics, and natural factors, and the main controlling factors were identified. On this basis, the prediction models were developed using a support vector machine, BP neural network model, decision tree model, and logistic model, and then the pollution situation of enterprises in the coking industry in 2025 and 2030 was predicted under different scenarios. The results indicated that time of service for the enterprise, time of establishment for the enterprise, the environmental illegal record, soil clay, and annual wind speed were the major controlling factors of soil pollution of enterprises in the coking industry. Compared with the support vector machine, BP neural network model, and decision tree model, the logistic model had a robust performance index, with an area under the receiver operating characteristic curve of 0.91. The accurate rate and recall rate were 84% and 88%, respectively. Under the optimistic scenario, there will be 1599 and 1695 plots with a high probability of pollution in the coking industry in 2025 and 2030, respectively; under the pessimistic scenario, there will be 1671 and 1715 plots with a high probability of pollution in the coking industry in 2025 and 2030, respectively. The results of this study provided a scientific basis for soil environmental remediation and eco-environmental strategy development for the coking industry.
更多查看译文
关键词
coking industry,machine learning,model performance,pollution prediction,scenario analysis
AI 理解论文
溯源树
样例
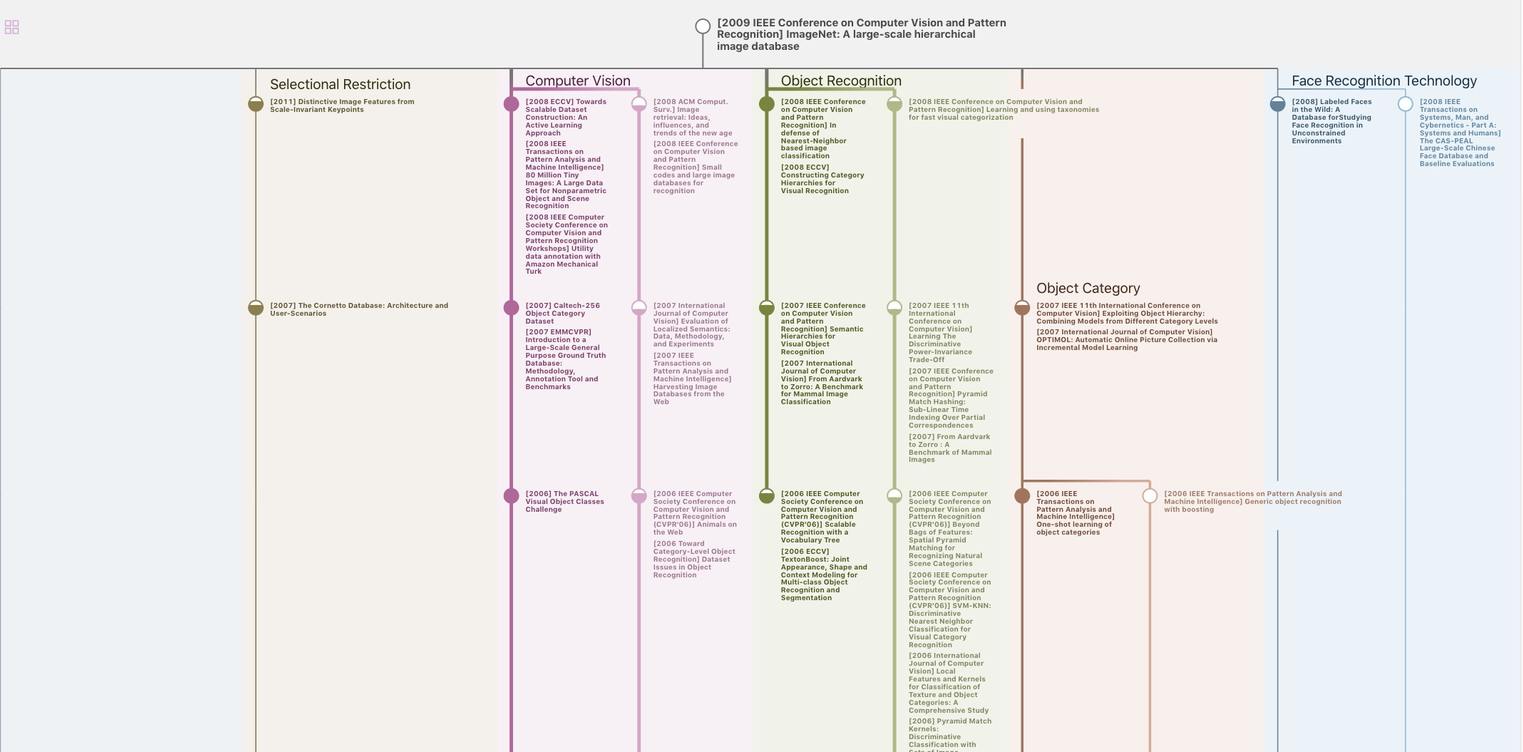
生成溯源树,研究论文发展脉络
Chat Paper
正在生成论文摘要