DCMA-Net: dual cross-modal attention for fine-grained few-shot recognition
Multimedia Tools and Applications(2024)
摘要
Since obtaining comprehensive labeled samples is expensive, the Fine-grained Few-shot Recognition task aims to identify unseen meta classes by using one or several labeled known meta classes. Besides, Fine-grained Recognition suffers some challenges such as minimal inter-class variation, backgrounds clutter, and most of the previous methods are single visual modality. In this paper, we propose a novel Dual Cross-modal Attention Network (DCMA-Net) to address the mentioned problems. Concretely, we first propose the Local Mutuality Attention branch that encodes contextual information by merging cross-modal information to learn more discriminatory information and increase inter-class differences. Meanwhile, we add a regularization mechanism to filter the visual features that match the attribute information to ensure the effectiveness of learning. Focusing on local features is easy to ignore instance information, so we propose the Global Correlation Attention branch which gains details activation representation acquired by global pooling of visual features serially in spatial and channel dimensions. This branch avoids learning bias as the counterpart of the Local Mutuality Attention branch. After that, both outputs of the two branches are aggregated as an integral feature embedding, which can be used to enhance the prototypes. Extensive experiments on CUB and SUN datasets demonstrate that our framework is effective. Particularly, our method has improved the accuracy of Prototype Network from 51.31 to 77.67 on 5-way 1-shot scenarios on the CUB dataset with Conv-4 backbone.
更多查看译文
关键词
Few-shot learning,Fine-grained image recognition,Attention mechanism,Cross-modal fusion,Prototype
AI 理解论文
溯源树
样例
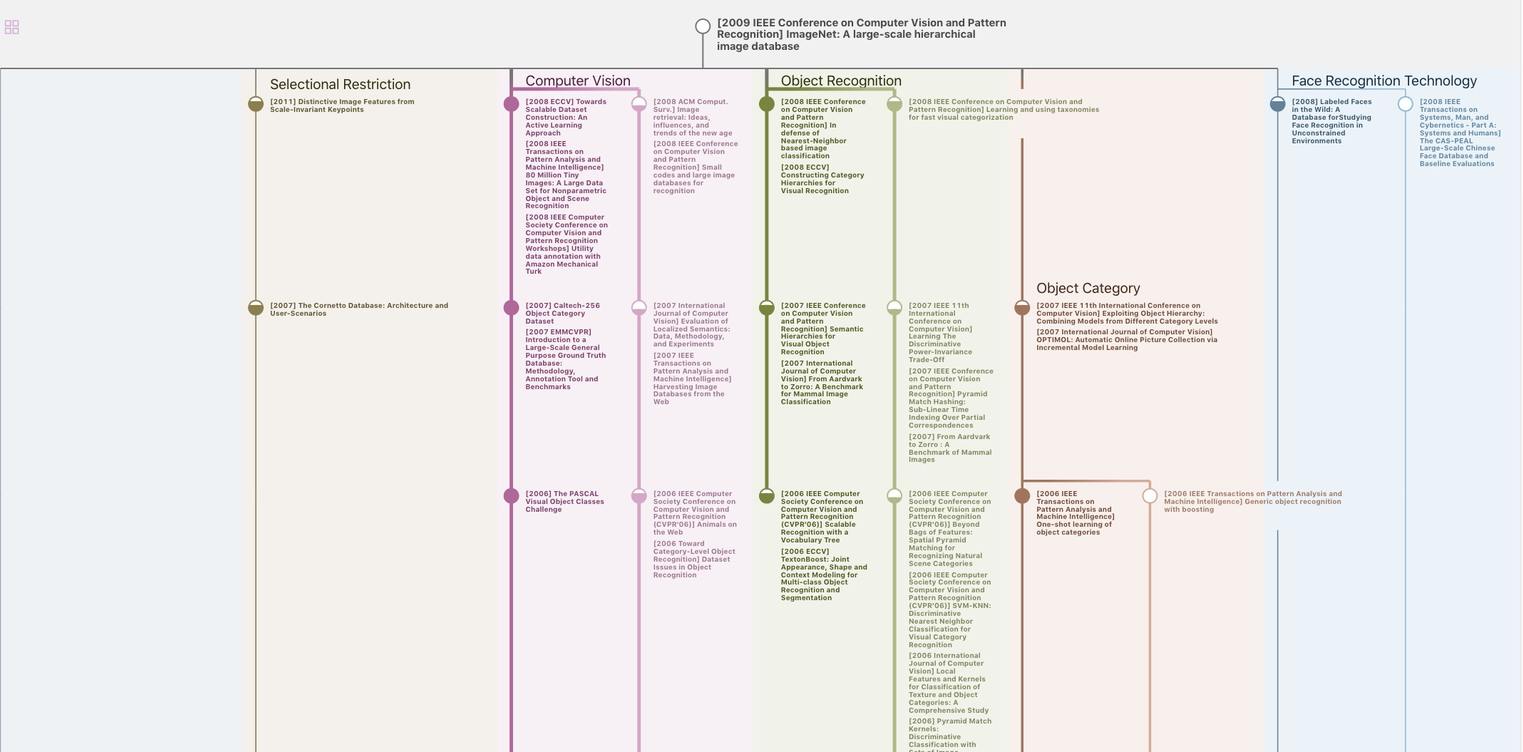
生成溯源树,研究论文发展脉络
Chat Paper
正在生成论文摘要