Dimensionality Reduction for Improving Out-of-Distribution Detection in Medical Image Segmentation
UNCERTAINTY FOR SAFE UTILIZATION OF MACHINE LEARNING IN MEDICAL IMAGING, UNSURE 2023(2023)
摘要
Clinically deployed deep learning-based segmentation models are known to fail on data outside of their training distributions While clinicians review the segmentations, these models do tend to perform well in most instances, which could exacerbate automation bias. Therefore, it is critical to detect out-of-distribution images at inference to warn the clinicians that the model likely failed. This work applies the Mahalanobis distance post hoc to the bottleneck features of a Swin UNETR model that segments the liver on T1-weighted magnetic resonance imaging. By reducing the dimensions of the bottleneck features with principal component analysis, images the model failed on were detected with high performance and minimal computational load. Specifically, the proposed technique achieved 92% area under the receiver operating characteristic curve and 94% area under the precision-recall curve and can run in seconds on a central processing unit.
更多查看译文
关键词
Out-of-distribution detection,Swin UNETR,Mahalanobis distance,Principal component analysis
AI 理解论文
溯源树
样例
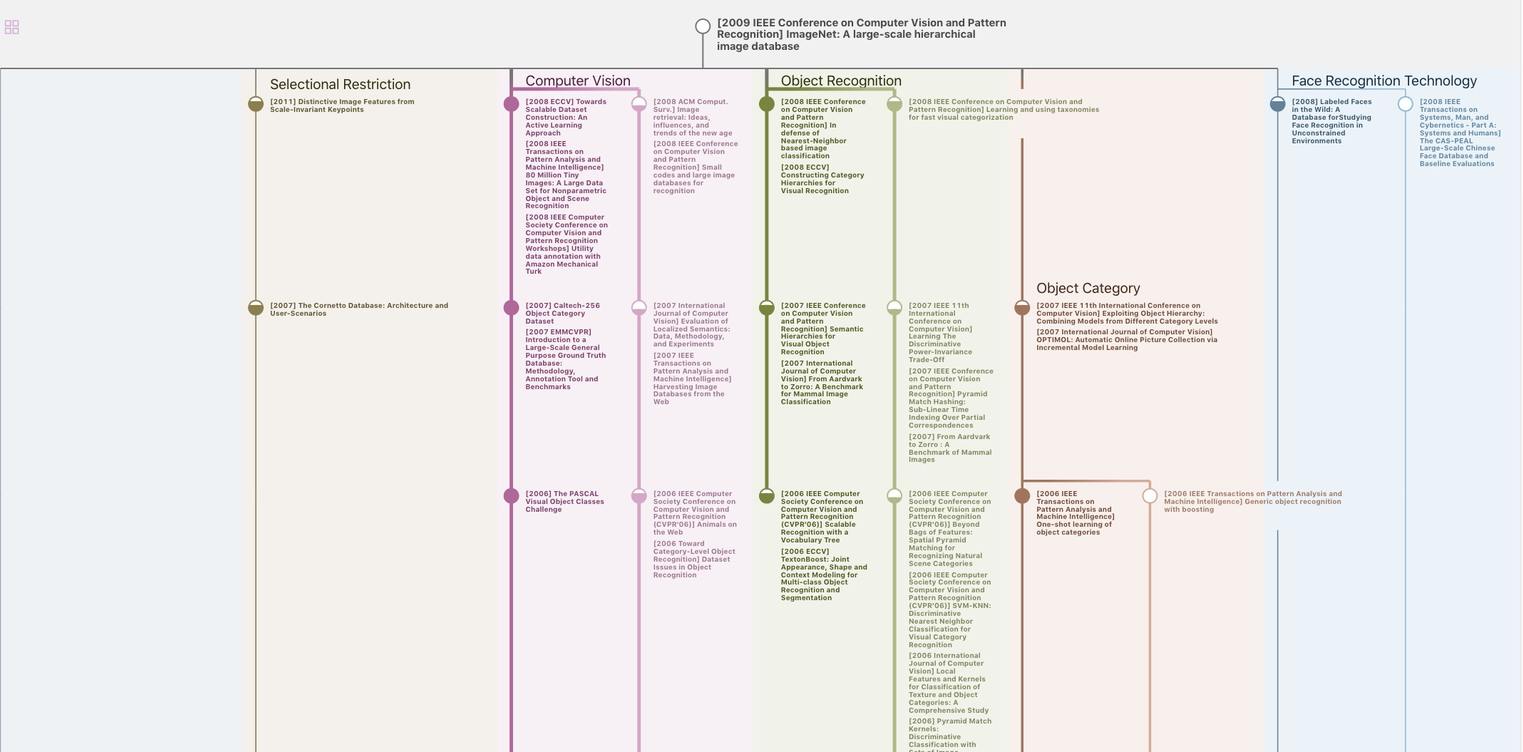
生成溯源树,研究论文发展脉络
Chat Paper
正在生成论文摘要