Robust estimation for functional logistic regression models
arXiv (Cornell University)(2023)
摘要
This paper addresses the problem of providing robust estimators under a functional logistic regression model. Logistic regression is a popular tool in classification problems with two populations. As in functional linear regression, regularization tools are needed to compute estimators for the functional slope. The traditional methods are based on dimension reduction or penalization combined with maximum likelihood or quasi--likelihood techniques and for that reason, they may be affected by misclassified points especially if they are associated to functional covariates with atypical behaviour. The proposal given in this paper adapts some of the best practices used when the covariates are finite--dimensional to provide reliable estimations. Under regularity conditions, consistency of the resulting estimators and rates of convergence for the predictions are derived. A numerical study illustrates the finite sample performance of the proposed method and reveals its stability under different contamination scenarios. A real data example is also presented.
更多查看译文
关键词
robust estimation,logistic regression,models
AI 理解论文
溯源树
样例
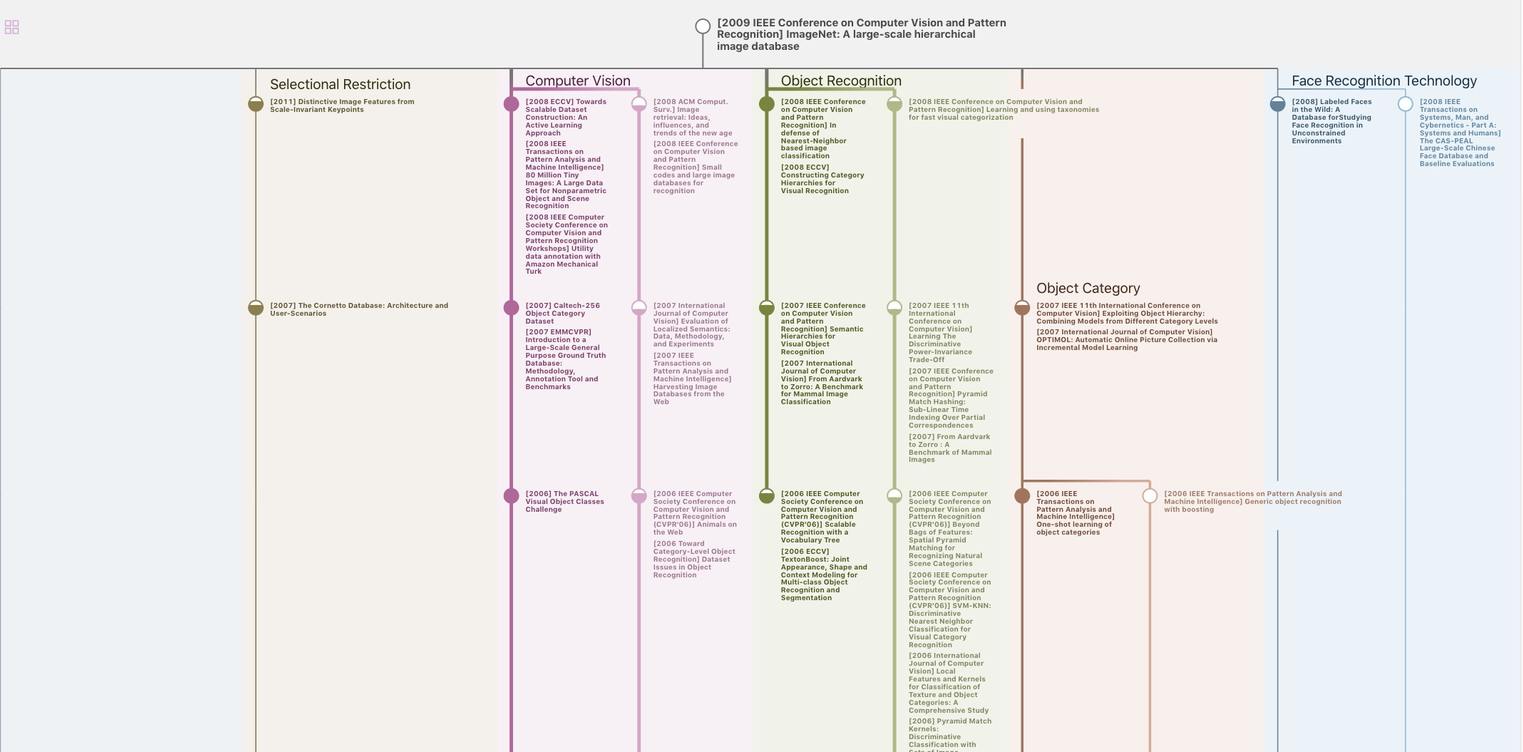
生成溯源树,研究论文发展脉络
Chat Paper
正在生成论文摘要