EDI: ESKF-based Disjoint Initialization for Visual-Inertial SLAM Systems
2023 IEEE/RSJ INTERNATIONAL CONFERENCE ON INTELLIGENT ROBOTS AND SYSTEMS, IROS(2023)
摘要
Visual-inertial initialization can be classified into joint and disjoint approaches. Joint approaches tackle both the visual and the inertial parameters together by aligning observations from feature-bearing points based on IMU integration then use a closed-form solution with visual and acceleration observations to find initial velocity and gravity. In contrast, disjoint approaches independently solve the Structure from Motion (SFM) problem and determine inertial parameters from up-to-scale camera poses obtained from pure monocular SLAM. However, previous disjoint methods have limitations, like assuming negligible acceleration bias impact or accurate rotation estimation by pure monocular SLAM. To address these issues, we propose EDI, a novel approach for fast, accurate, and robust visual-inertial initialization. Our method incorporates an Error-state Kalman Filter (ESKF) to estimate gyroscope bias and correct rotation estimates from monocular SLAM, overcoming dependence on pure monocular SLAM for rotation estimation. To estimate the scale factor without prior information, we offer a closed-form solution for initial velocity, scale, gravity, and acceleration bias estimation. To address gravity and acceleration bias coupling, we introduce weights in the linear least-squares equations, ensuring acceleration bias observability and handling outliers. Extensive evaluation on the EuRoC dataset shows that our method achieves an average scale error of 5.8% in less than 3 seconds, outperforming other state-of-the-art disjoint visual-inertial initialization approaches, even in challenging environments and with artificial noise corruption.
更多查看译文
AI 理解论文
溯源树
样例
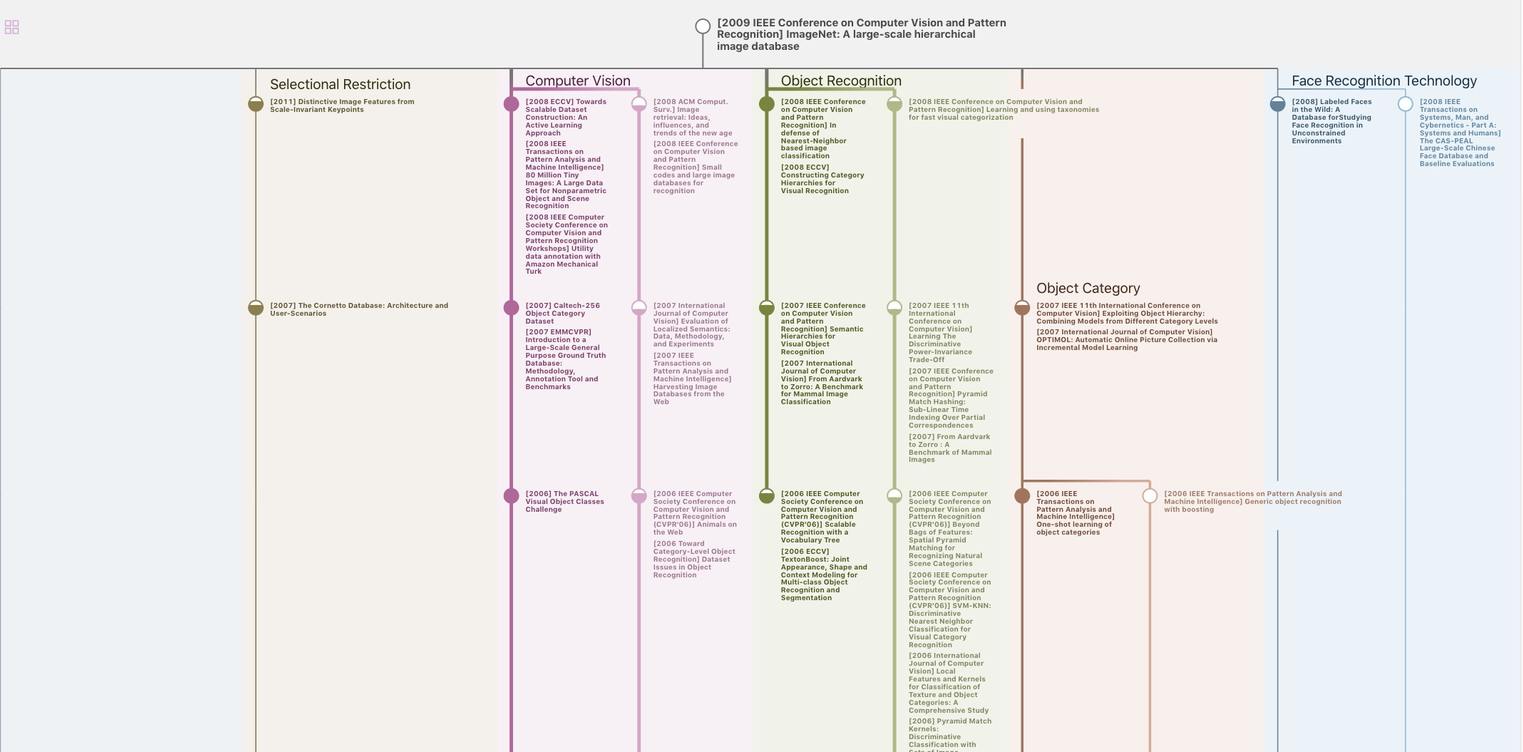
生成溯源树,研究论文发展脉络
Chat Paper
正在生成论文摘要