Reconstructing Lyman-$\alpha$ Fields from Low-Resolution Hydrodynamical Simulations with Deep Learning
The Astrophysical Journal(2023)
摘要
Hydrodynamical cosmological simulations are a powerful tool for accurately predicting the properties of the intergalactic medium (IGM) and for producing mock skies that can be compared against observational data. However, the need to resolve density fluctuation in the IGM puts a stringent requirement on the resolution of such simulations which in turn limits the volumes which can be modelled, even on most powerful supercomputers. In this work, we present a novel modeling method which combines physics-driven simulations with data-driven generative neural networks to produce outputs that are qualitatively and statistically close to the outputs of hydrodynamical simulations employing 8 times higher resolution. We show that the Ly-$\alpha$ flux field, as well as the underlying hydrodynamic fields, have greatly improved statistical fidelity over a low-resolution simulation. Importantly, the design of our neural network allows for sampling multiple realizations from a given input, enabling us to quantify the model uncertainty. Using test data, we demonstrate that this model uncertainty correlates well with the true error of the Ly-$\alpha$ flux prediction. Ultimately, our approach allows for training on small simulation volumes and applying it to much larger ones, opening the door to producing accurate Ly-$\alpha$ mock skies in volumes of Hubble size, as will be probed with DESI and future spectroscopic sky surveys.
更多查看译文
关键词
Cosmology,Intergalactic medium,Large-scale structure of the universe,Lyman alpha forest,Convolutional neural networks
AI 理解论文
溯源树
样例
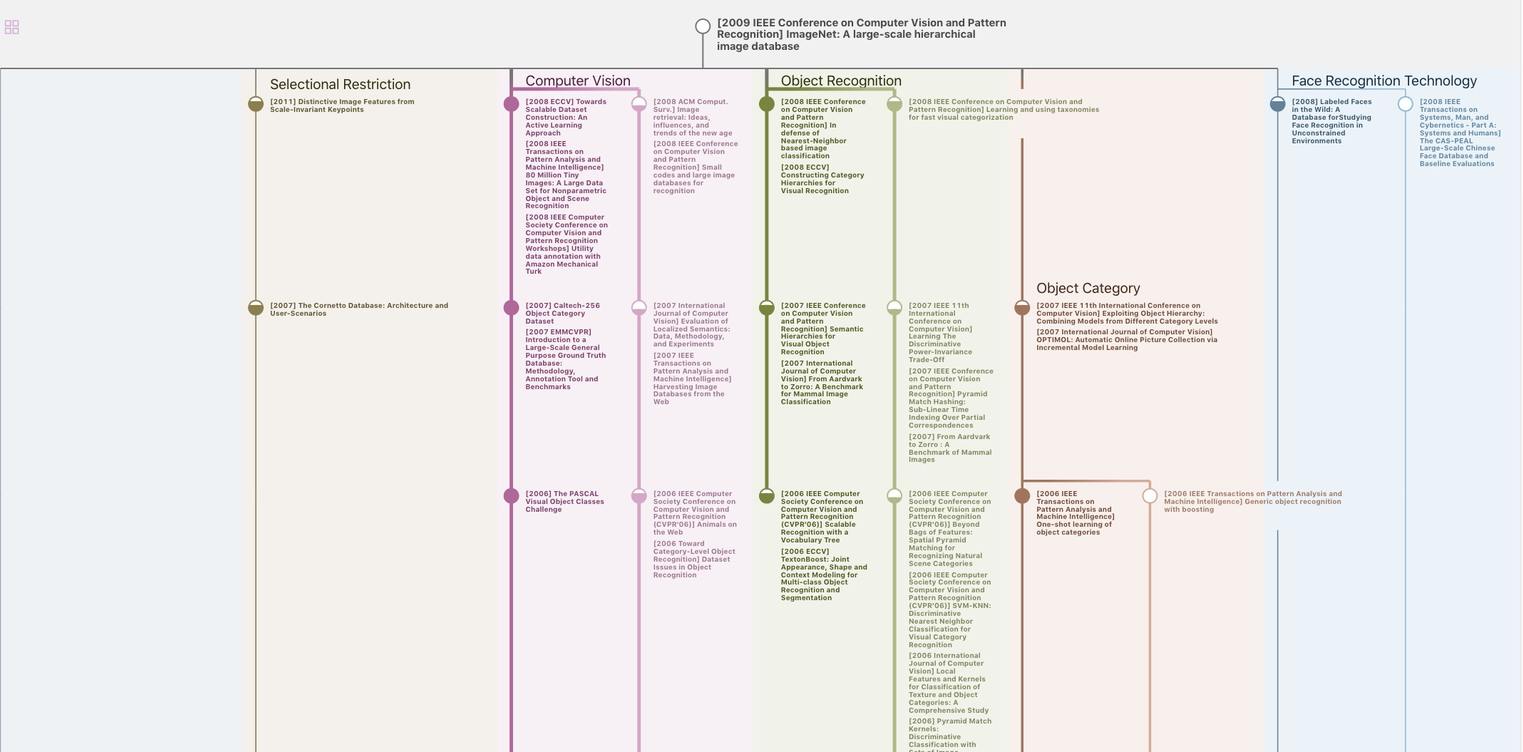
生成溯源树,研究论文发展脉络
Chat Paper
正在生成论文摘要