Two-subnet network for real-world image denoising
Multimedia Tools and Applications(2024)
摘要
Previous researches in synthetic noise image denoising have performed well. However, while these methods remove real-world noise, they result in loss of image detail. To solve the problem, this article proposes a two-subnet network for real-world image denoising (TSIDNet). The proposed TSIDNet consists of two subnets, each subnet is designed with independent purpose. The data processing subnet is used to fit the current training data for denoising. We design a cross fusion module in data processing subnet to fuse the encoder information well and then pass the fusion result to the decoder. To decode the context well, we also design a residual attention block based on polarized self-attention as the decoder. The feature extracting subnet based on transfer learning is used to obtain global robust features of the degraded images. By fusing the information from both subnets, high-quality noise-free images can be obtained. Quantitative and qualitative experimental results on four real-world noisy datasets demonstrate the excellent generalization and denoising performance of our method.
更多查看译文
关键词
Image denoising,Real-world,Deep learning,Fusion mechanism,Transfer learning
AI 理解论文
溯源树
样例
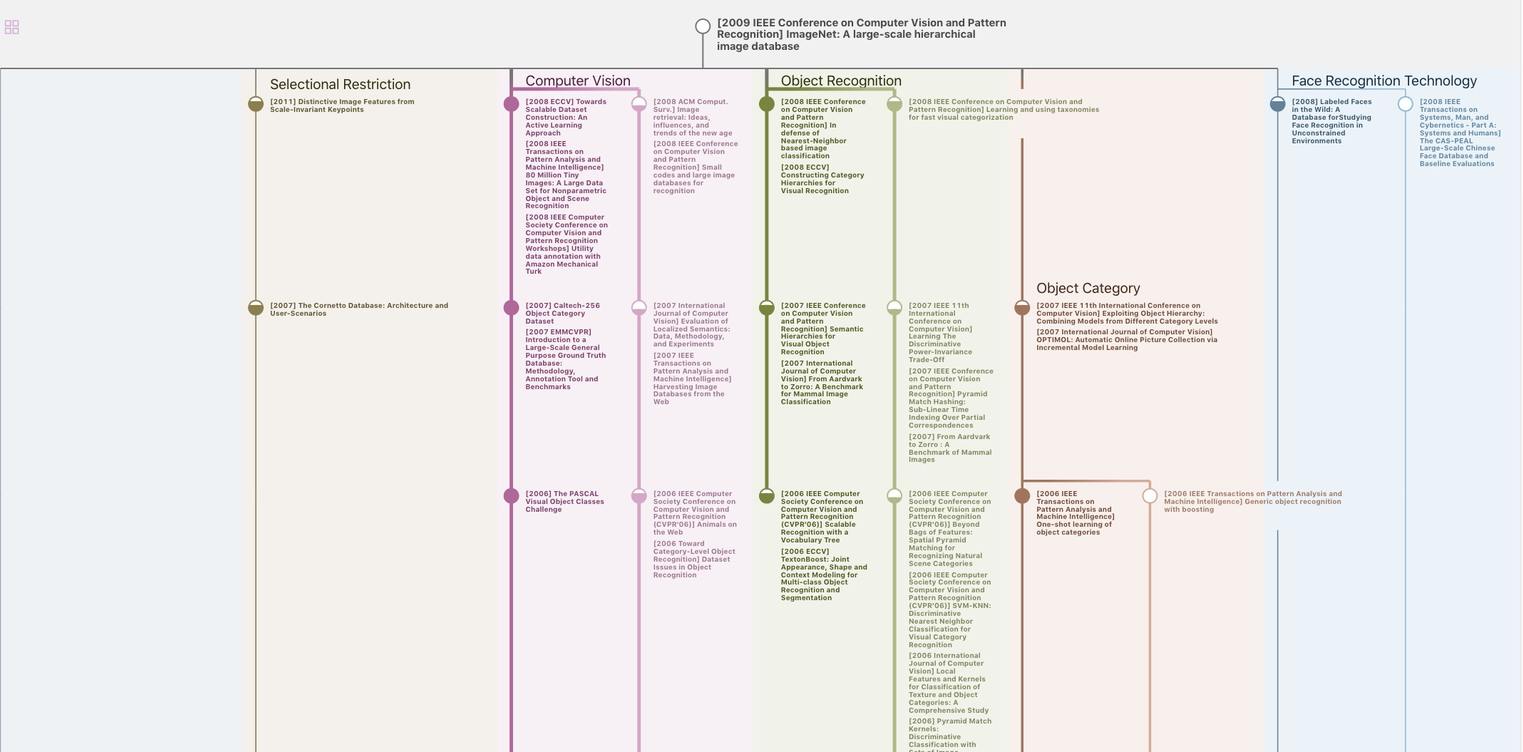
生成溯源树,研究论文发展脉络
Chat Paper
正在生成论文摘要