A novel deep neural network model using network deconvolution with attention based activation for crop disease classification
Multimedia Tools and Applications(2024)
摘要
Accurate classification of crop diseases in its initial phase can help farmers to take necessary actions against the damage to their crops. The paper presents a Deep Learning (DL)-based crop disease classification approach that uses network deconvolution operation and attention-based activation function in each feature extraction layer. The existence of channel-wise and pixel-wise correlations in real-world images makes model training challenging. There is hardly any method available in the current literature that proposes the image correlation removal technique. The network deconvolution operation can efficiently remove both the correlations layer-wise. Hence, it is used in the proposed model. An attention-based activation function called AReLU is adopted in the model. The significance of AReLU activation function is to facilitate faster training. It can deal with gradient vanishing issue. The study considered Plant Village (PV), Tomato, and Grape datasets for performance evaluation. 80: 20 train-test split of the dataset was considered. The proposed model delivered significant results in comparison to other existing models, offering 100%, 99.27% and 99.10% classification accuracies and 99.88%, 99.06% and 99.01% F1-scores on Grape, PV and Tomato datasets respectively.
更多查看译文
关键词
Image classification,Crop disease,Convolutional neural network,Network deconvolution,Attention based activation
AI 理解论文
溯源树
样例
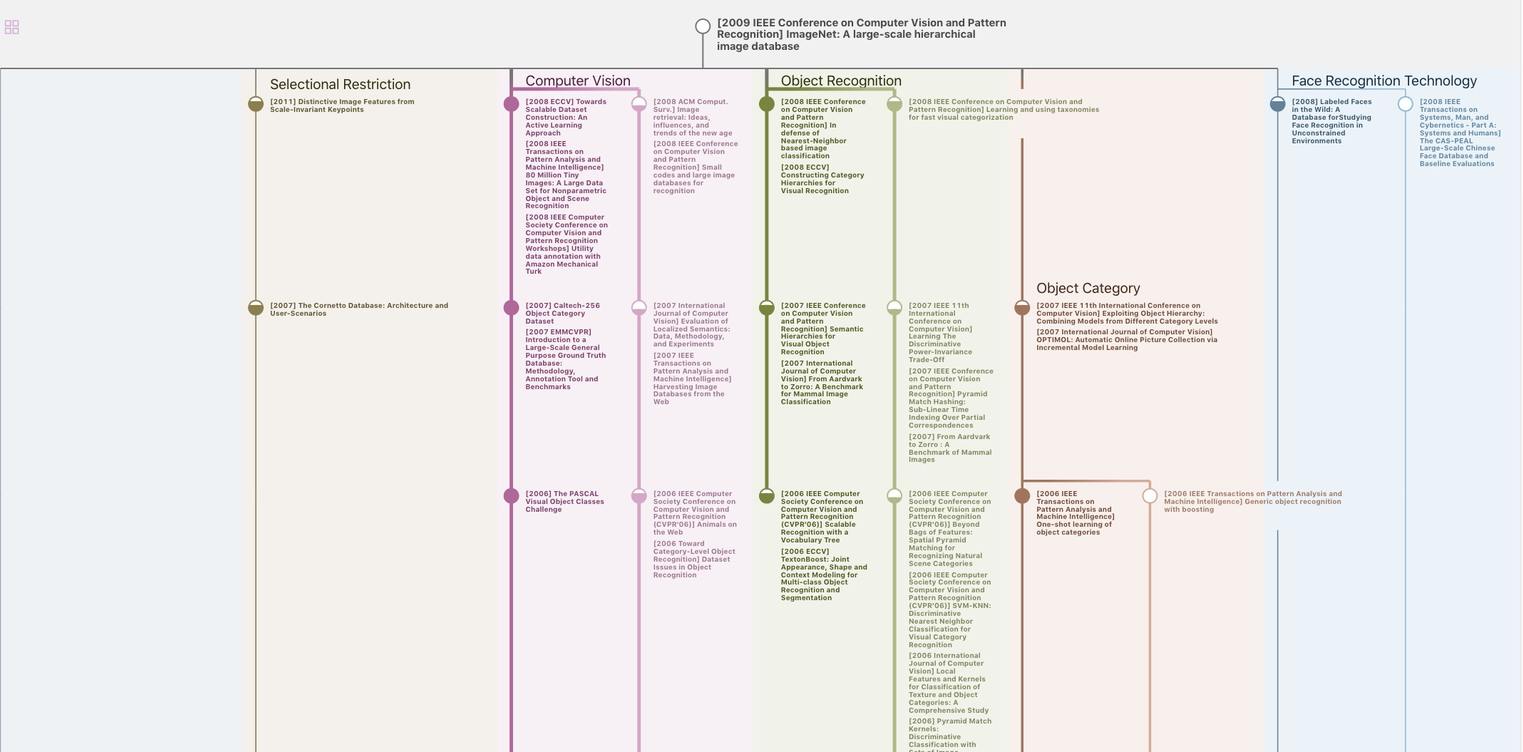
生成溯源树,研究论文发展脉络
Chat Paper
正在生成论文摘要