Dense deep transformer for medical image segmentation: DDTraMIS
Multimedia Tools and Applications(2024)
摘要
In this work, DDTraMIS architecture based on vision has been designed for medical image segmentation for different medical imaging such as MRI and CT-scan. This methodology contributes novel hybrid features extracted with a bi-directional attention-based transformer encoder-decoder network along with all stage features fused with an approximation fusing algorithm. The novelty is to combine Convolution neural networks (CNNs) and shift-invariant methods to develop hybrid features. For the verification of this novel network experiment, three different datasets, such as the ACDC, LiTS, and BraTS datasets have been used. Performance analysis has been conducted using the dice similarity coefficient (DSC) and the Hausdorff distance (HD) metrics. The proposed architecture accomplished DSC values of 92.80, 96.45, 72.80, and 73.12 for ACDC, LiTS Liver, LiTS tumor, and BraTS tumor segmentation analysis, respectively. Similarly, HD metric numeric values are 8.53, 12.84, 9.69, and 10.38 for ACDC, LiTS Liver, LiTS tumor, and BraTS tumor segmentation analysis, respectively. With advantageous performance for highly features incorporated, this method learned from tiny to extensive scaling information. Comparative and quantitative analysis has proven its superior performance and effective segmenting architecture.
更多查看译文
关键词
Vision transformer,Medical image segmentation,Attention network,Convolution neural network,Shift-invariant feature
AI 理解论文
溯源树
样例
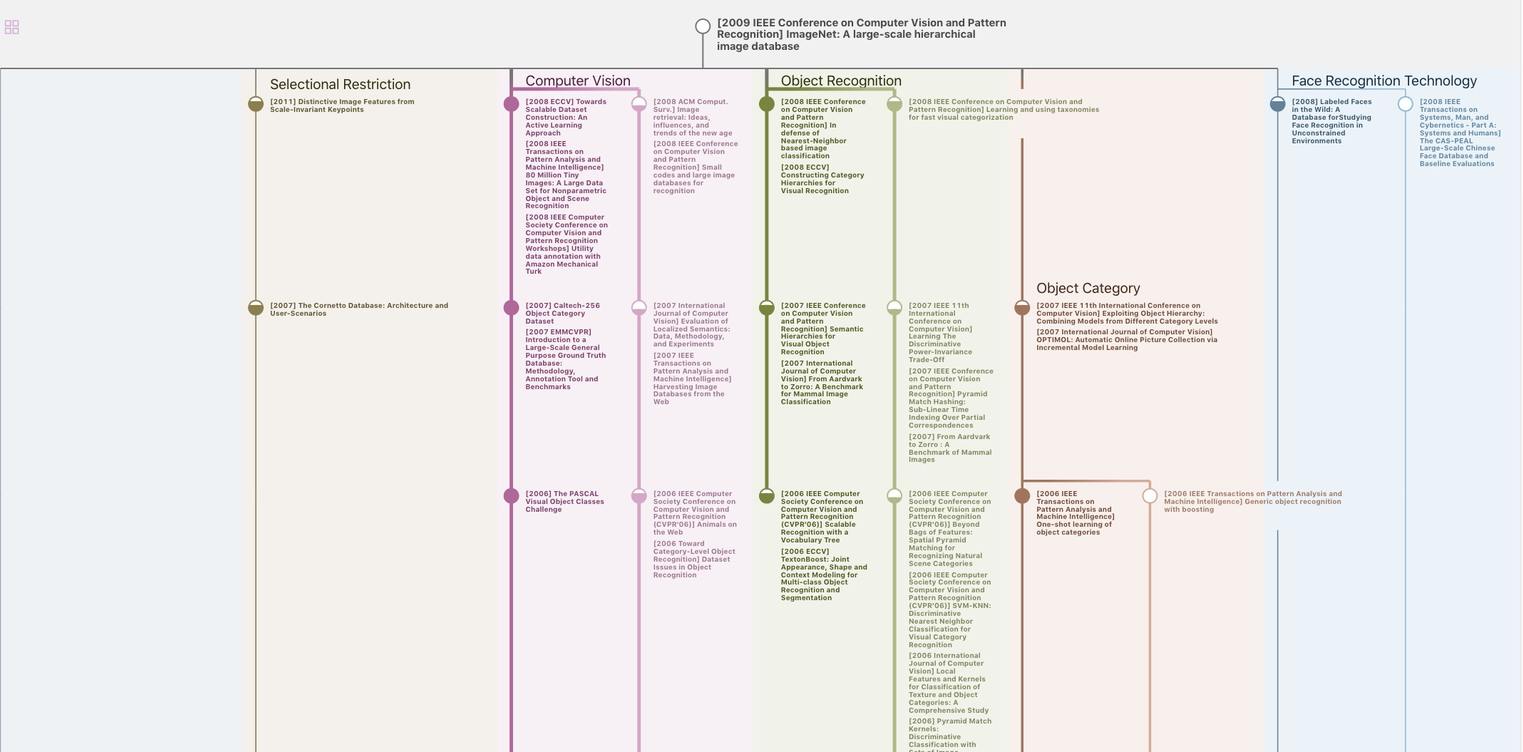
生成溯源树,研究论文发展脉络
Chat Paper
正在生成论文摘要