Evaluation of wastewater-based epidemiology of COVID-19 approaches in Singapore's 'closed-system' scenario: A long-term country-wide assessment.
Water research(2023)
摘要
With the COVID-19 pandemic the use of WBE to track diseases spread has rapidly evolved into a widely applied strategy worldwide. However, many of the current studies lack the necessary systematic approach and supporting quality of epidemiological data to fully evaluate the effectiveness and usefulness of such methods. Use of WBE in a very low disease prevalence setting and for long-term monitoring has yet to be validated and it is critical for its intended use as an early warning system. In this study we seek to evaluate the sensitivity of WBE approaches under low prevalence of disease and ability to provide early warning. Two monitoring scenarios were used: (i) city wide monitoring (population 5,700,000) and (ii) community/localized monitoring (population 24,000 to 240,000). Prediction of active cases by WBE using multiple linear regression shows that a multiplexed qPCR approach with three gene targets has a significant advantage over single-gene monitoring approaches, with R2 = 0.832 (RMSE 0.053) for an analysis using N, ORF1ab and S genes (R2 = 0.677 to 0.793 for single gene strategies). A predicted disease prevalence of 0.001% (1 in 100,000) for a city-wide monitoring was estimated by the multiplexed RT-qPCR approach and was corroborated by epidemiological data evidence in three 'waves'. Localized monitoring setting shows an estimated detectable disease prevalence of ∼0.002% (1 in 56,000) and is supported by the geospatial distribution of active cases and local population dynamics data. Data analysis also shows that this approach has a limitation in sensitivity, or hit rate, of 62.5 % and an associated high miss rate (false negative rate) of 37.5 % when compared to available epidemiological data. Nevertheless, our study shows that, with enough sampling resolution, WBE at a community level can achieve high precision and accuracies for case detection (96 % and 95 %, respectively) with low false omission rate (4.5 %) even at low disease prevalence levels.
更多查看译文
AI 理解论文
溯源树
样例
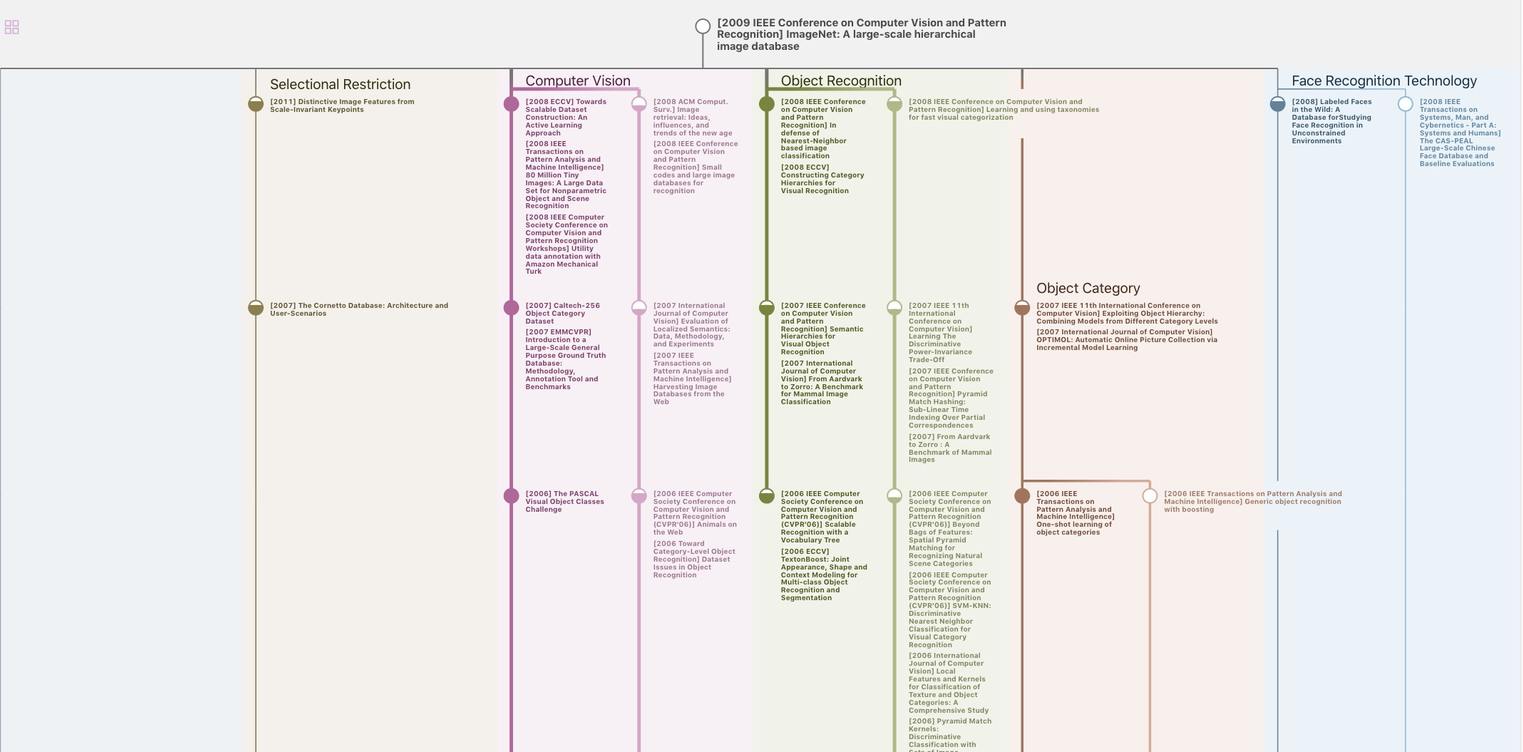
生成溯源树,研究论文发展脉络
Chat Paper
正在生成论文摘要