SpaDen : Sparse and Dense Keypoint Estimation for Real-World Chart Understanding
ICDAR (2)(2023)
摘要
We introduce a novel bottom-up approach for the extraction of chart data. Our model utilizes images of charts as inputs and learns to detect keypoints (KP), which are used to reconstruct the components within the plot area. Our novelty lies in detecting a fusion of continuous and discrete KP as predicted heatmaps. A combination of sparse and dense per-pixel objectives coupled with a uni-modal self-attention-based feature-fusion layer is applied to learn KP embeddings. Further leveraging deep metric learning for unsupervised clustering, allows us to segment the chart plot area into various objects. By further matching the chart components to the legend, we are able to obtain the data series names. A post-processing threshold is applied to the KP embeddings to refine the object reconstructions and improve accuracy. Our extensive experiments include an evaluation of different modules for KP estimation and the combination of deep layer aggregation and corner pooling approaches. The results of our experiments provide extensive evaluation for the task of real-world chart data extraction.
更多查看译文
关键词
dense keypoint estimation,chart,sparse,real-world
AI 理解论文
溯源树
样例
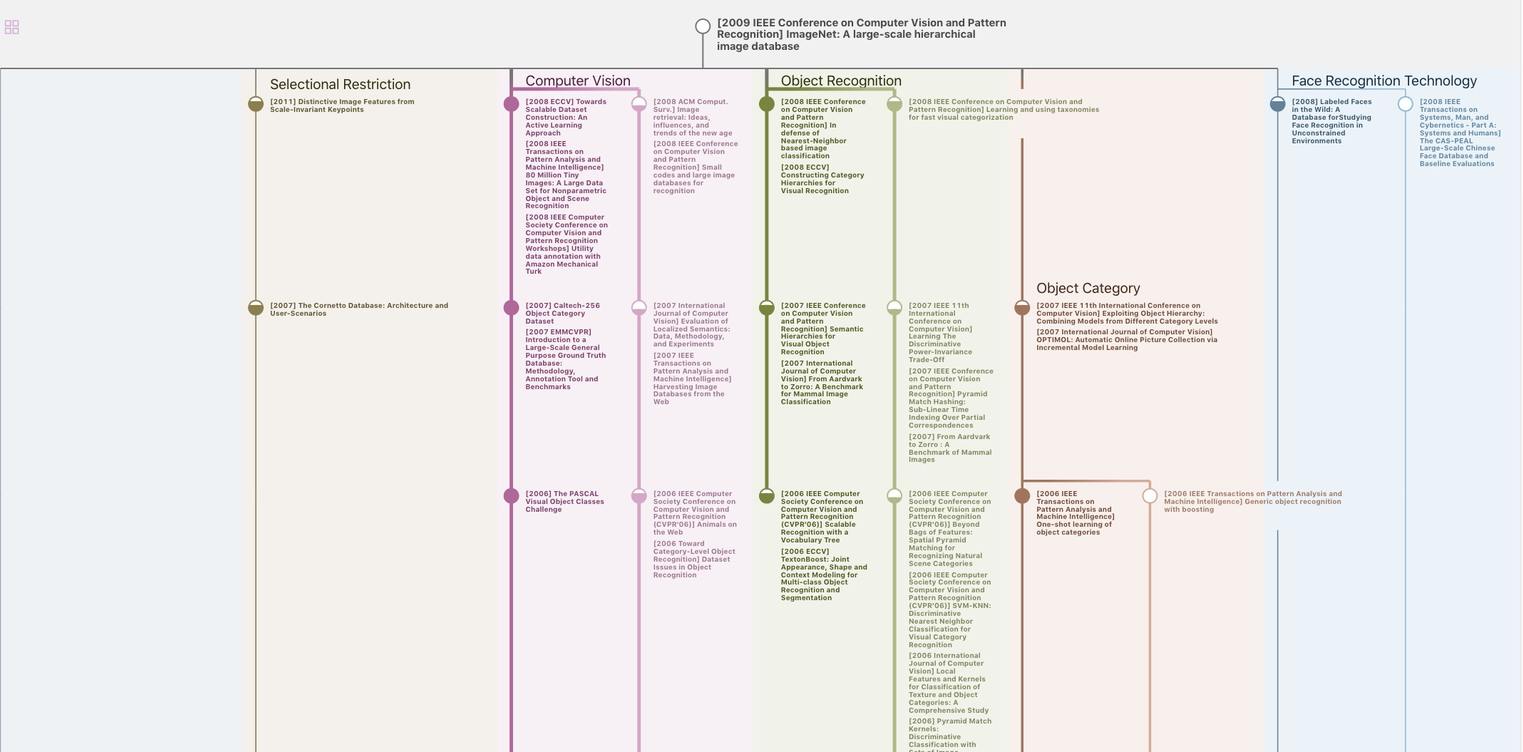
生成溯源树,研究论文发展脉络
Chat Paper
正在生成论文摘要