Safe residual reinforcement learning for helicopter aerial refueling
2023 IEEE/ASME International Conference on Advanced Intelligent Mechatronics (AIM)(2023)
摘要
Autonomous helicopter aerial refueling is a challenging problem because of the complex aerodynamic interactions between the helicopter, the tanker and the refueling hose-drogue system. Methodologies solely relying on model-based control approaches are unable to directly address the aerodynamic interactions, whereas pure data-driven methods such as reinforcement learning (RL) often do not provide safety guarantees. Therefore, in this paper, we propose a novel residual RL control methodology that works in conjunction with a model-based outer-loop position controller. Further, we incorporate a safe RL algorithm that assures probabilistic safety guarantees by imposing appropriate constraints. This algorithm leverages the primal-dual formulation of a constrained optimal control problem to solve a sequence of RL problems that ultimately guarantees a probabilistic safety assurance requirement. The RL agent is trained in a simulation platform that consists of a reduced-order helicopter model and a state-dependent control mixer that appropriately delegates the control authority between the outer-loop controller and the RL controller. Once trained, the RL agent is deployed on a physics-based high-fidelity helicopter model without additional parameter tuning. These high-fidelity simulations reveal that the application of the proposed methodology yields a mean 2-norm error of 0.25m at the time of docking, which outperforms a purely model-based controller by 24%.
更多查看译文
AI 理解论文
溯源树
样例
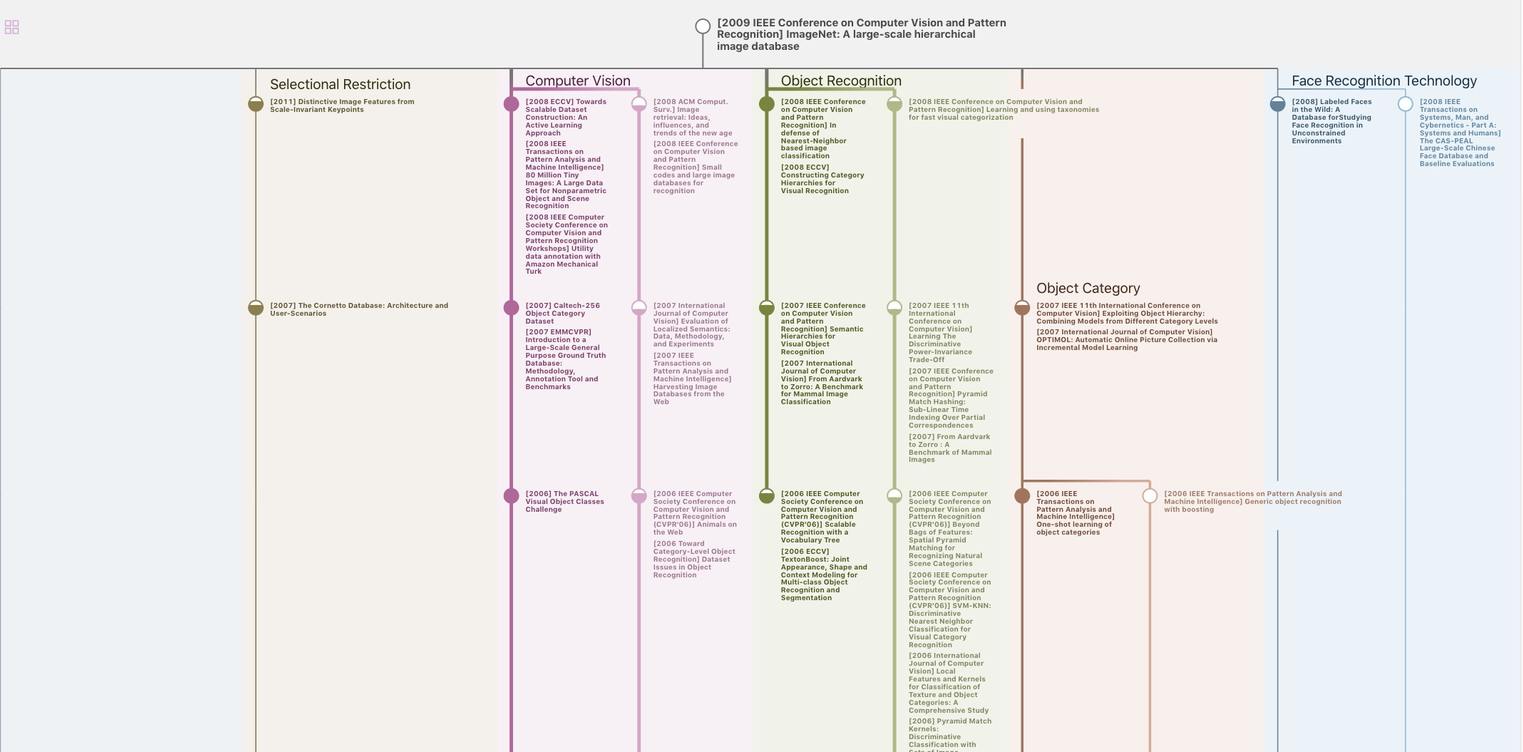
生成溯源树,研究论文发展脉络
Chat Paper
正在生成论文摘要