A Parametrical Model for Instance-Dependent Label Noise
IEEE TRANSACTIONS ON PATTERN ANALYSIS AND MACHINE INTELLIGENCE(2023)
摘要
In label-noise learning, estimating the transition matrix is a hot topic as the matrix plays an important role in building statistically consistent classifiers. Traditionally, the transition from clean labels to noisy labels (i.e., clean-label transition matrix (CLTM)) has been widely exploited on class-dependent label-noise (wherein all samples in a clean class share the same label transition matrix). However, the CLTM cannot handle the more common instance-dependent label-noise well (wherein the clean-to-noisy label transition matrix needs to be estimated at the instance level by considering the input quality). Motivated by the fact that classifiers mostly output Bayes optimal labels for prediction, in this paper, we study to directly model the transition from Bayes optimal labels to noisy labels (i.e., Bayes-Label Transition Matrix (BLTM)) and learn a classifier to predict Bayes optimal labels. Note that given only noisy data, it is ill-posed to estimate either the CLTM or the BLTM. But favorably, Bayes optimal labels have no uncertainty compared with the clean labels, i.e., the class posteriors of Bayes optimal labels are one-hot vectors while those of clean labels are not. This enables two advantages to estimate the BLTM, i.e., (a) a set of examples with theoretically guaranteed Bayes optimal labels can be collected out of noisy data; (b) the feasible solution space is much smaller. By exploiting the advantages, this work proposes a parametrical model for estimating the instance-dependent label-noise transition matrix by employing a deep neural network, leading to better generalization and superior classification performance.
更多查看译文
关键词
Label-noise learning,label transition matrix,statistically consistent algorithm
AI 理解论文
溯源树
样例
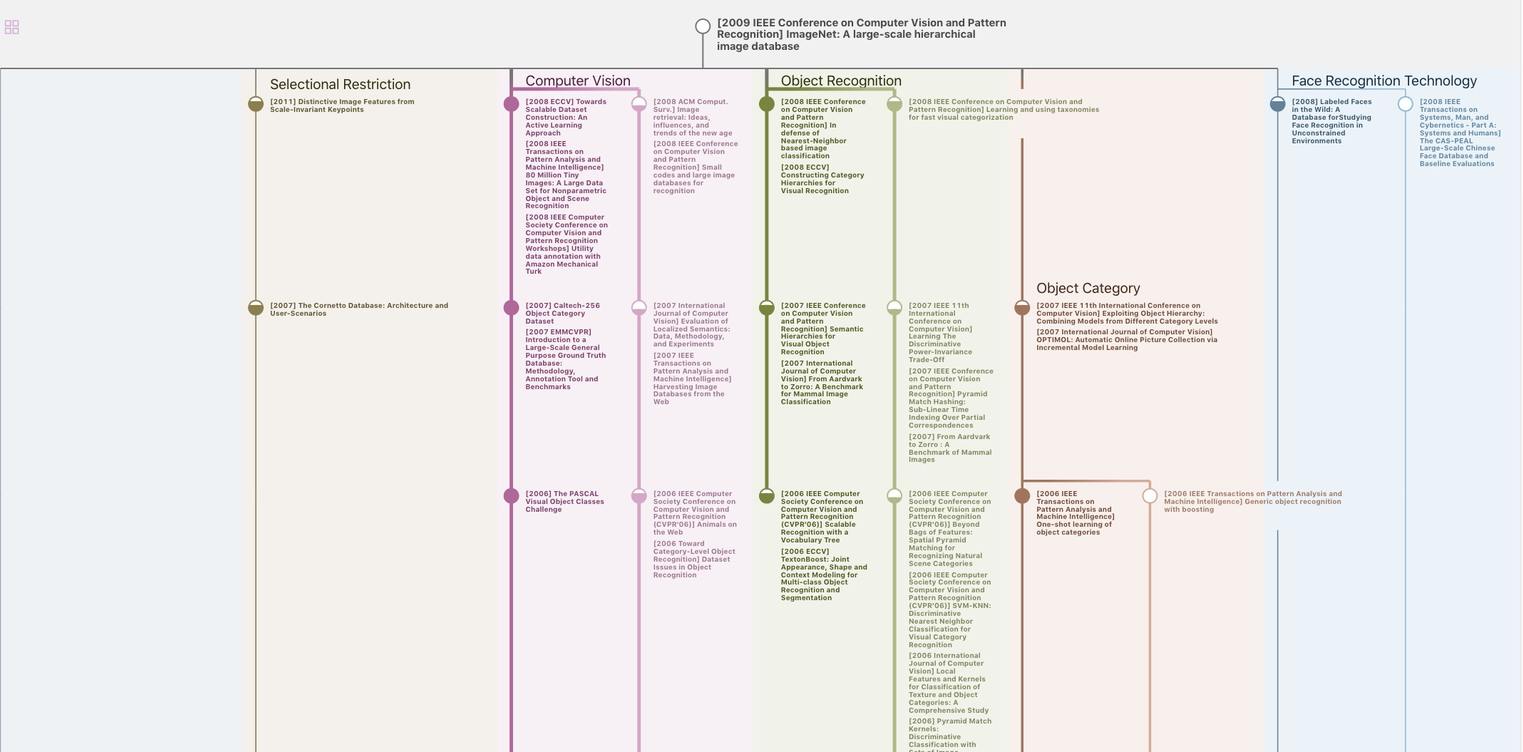
生成溯源树,研究论文发展脉络
Chat Paper
正在生成论文摘要