Automatic Defect Classification Using Semi-Supervised Learning With Defect Localization
IEEE Transactions on Semiconductor Manufacturing(2023)
摘要
Automatic defect classification (ADC) systems automatically classify defects that inevitably occur during semiconductor manufacturing processes. ADC is the beginning of defect management that increases the yield of semiconductor chip production, and prevents accidents in the process. It takes a lot of engineer’s labor to classify defects, but ADC can be the answer to classify all defects at low cost. ADC employs the defect image of a wafer surface, captured using scanning electron microscopy (SEM). SEM images can feature a variety of backgrounds based on the defect position on the wafer and the process steps. The manual classification of SEM images involves significant labor costs related to hiring experienced engineers. Despite recent ADC studies reporting good performance, the lack of labeled images and various backgrounds make it difficult to apply ADC in actual manufacturing processes. To address this issue, automated defect classification with defect localization is proposed herein. To this end, a classification model is specifically designed for reducing the effect of varying backgrounds using defect localization. Defect localization uses an object detection model to provide the region information of defects in SEM images. We aimed to design a classification model and defect detection model using semi-supervised learning to reduce labeling costs. Experimental results indicate that the classification performance, over 15 classes, is improved by 12.56% (9.82%p), as compared with that of supervised models.
更多查看译文
关键词
Auto defect classification, semi-supervised learning, object detection, image classification, deep learning
AI 理解论文
溯源树
样例
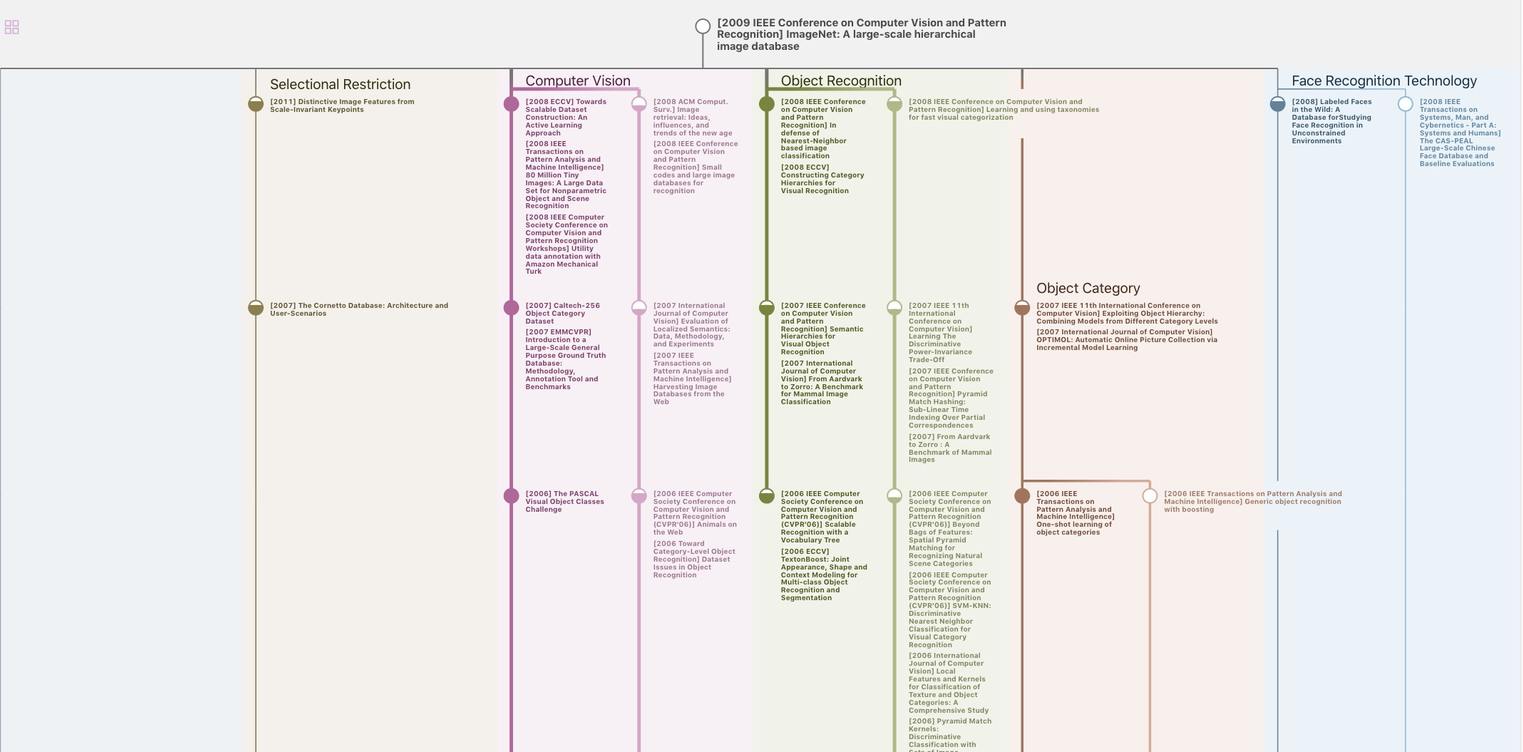
生成溯源树,研究论文发展脉络
Chat Paper
正在生成论文摘要