Angular Central Gaussian and Watson Mixture Models for Assessing Dynamic Functional Brain Connectivity During a Motor Task
2023 IEEE International Conference on Acoustics, Speech, and Signal Processing Workshops (ICASSPW)(2023)
摘要
The development of appropriate models for dynamic functional connectivity is imperative to gain a better understanding of the brain both during rest and while performing a task. Leading eigenvector dynamics analysis is among the favored methods for assessing frame-wise connectivity, but eigenvectors are distributed on the sign-symmetric unit hypersphere, which is typically disregarded during modeling. Here we develop both mixture model and Hidden Markov model formulations for two sign-symmetric spherical statistical distributions and display their performance on synthetic data and task-fMRI data involving a finger-tapping task.
更多查看译文
关键词
Dynamic functional connectivity,Leading eigenvector dynamics analysis,Watson,Angular Central Gaussian,Hidden Markov models
AI 理解论文
溯源树
样例
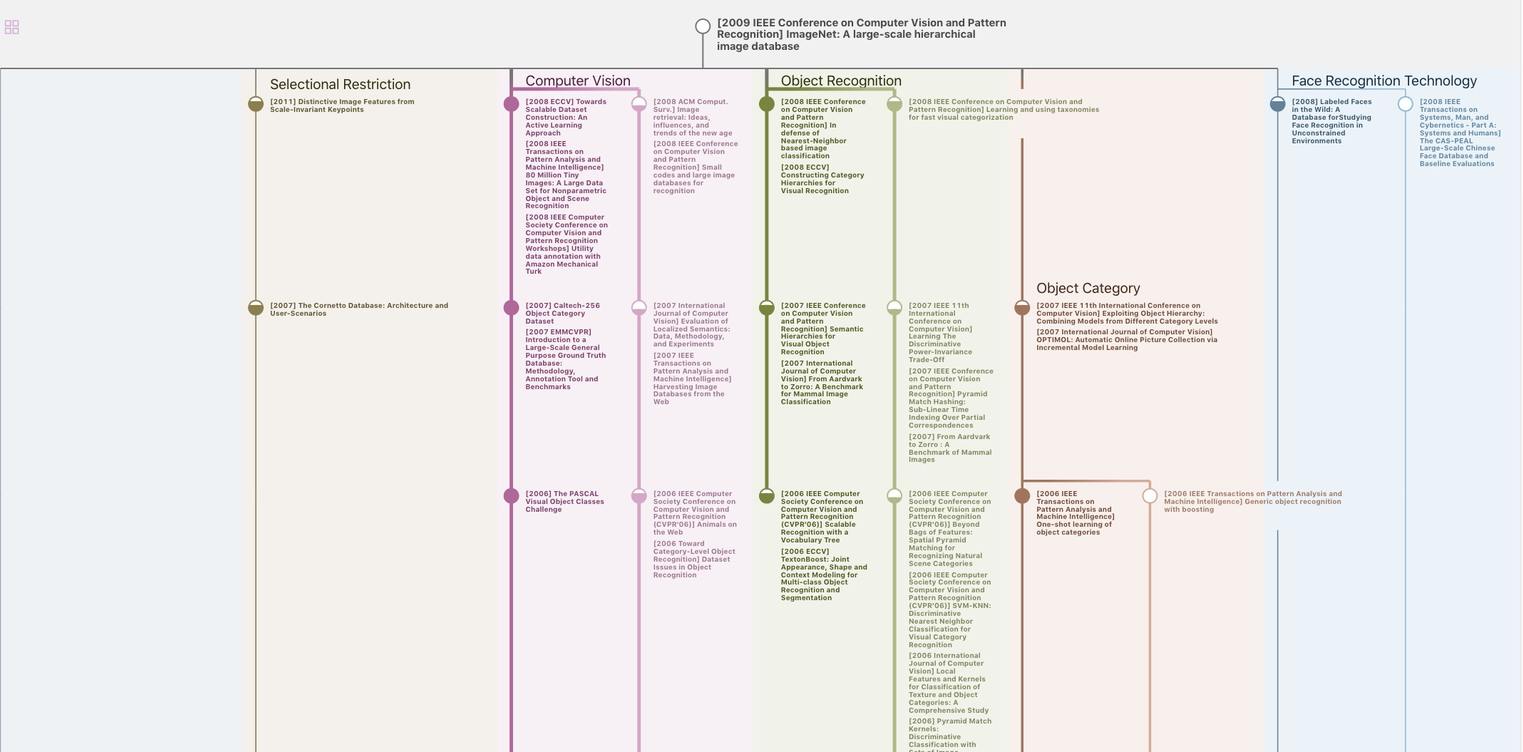
生成溯源树,研究论文发展脉络
Chat Paper
正在生成论文摘要