HEAP: a task adaptive-based explainable deep learning framework for enhancer activity prediction.
Briefings in bioinformatics(2023)
摘要
Enhancers are crucial cis-regulatory elements that control gene expression in a cell-type-specific manner. Despite extensive genetic and computational studies, accurately predicting enhancer activity in different cell types remains a challenge, and the grammar of enhancers is still poorly understood. Here, we present HEAP (high-resolution enhancer activity prediction), an explainable deep learning framework for predicting enhancers and exploring enhancer grammar. The framework includes three modules that use grammar-based reasoning for enhancer prediction. The algorithm can incorporate DNA sequences and epigenetic modifications to obtain better accuracy. We use a novel two-step multi-task learning method, task adaptive parameter sharing (TAPS), to efficiently predict enhancers in different cell types. We first train a shared model with all cell-type datasets. Then we adapt to specific tasks by adding several task-specific subset layers. Experiments demonstrate that HEAP outperforms published methods and showcases the effectiveness of the TAPS, especially for those with limited training samples. Notably, the explainable framework HEAP utilizes post-hoc interpretation to provide insights into the prediction mechanisms from three perspectives: data, model architecture and algorithm, leading to a better understanding of model decisions and enhancer grammar. To the best of our knowledge, HEAP will be a valuable tool for insight into the complex mechanisms of enhancer activity.
更多查看译文
关键词
explainable deep learning framework,deep learning,activity,task,adaptive-based
AI 理解论文
溯源树
样例
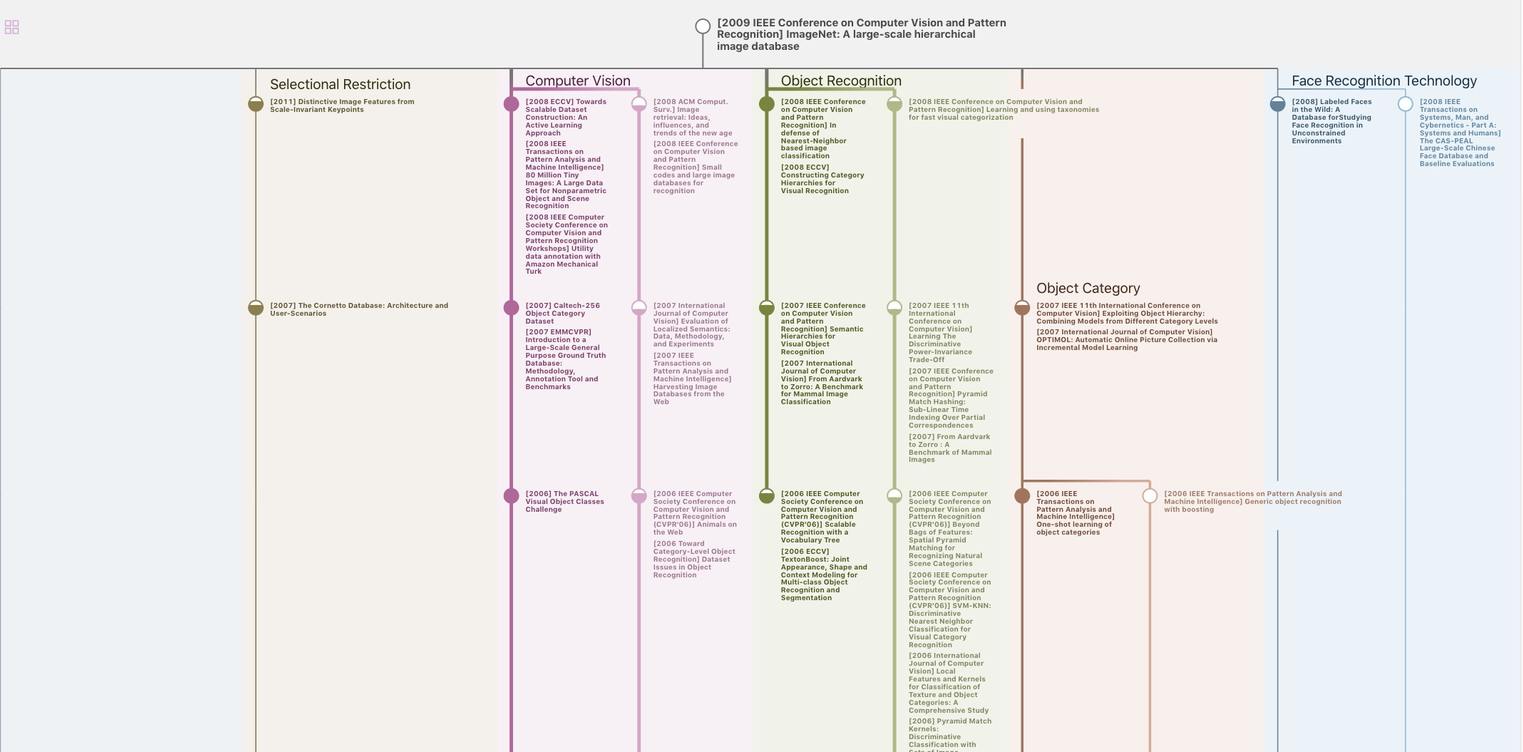
生成溯源树,研究论文发展脉络
Chat Paper
正在生成论文摘要