Deep multi-view contrastive learning for cancer subtype identification
Briefings in bioinformatics(2023)
摘要
Cancer heterogeneity has posed great challenges in exploring precise therapeutic strategies for cancer treatment. The identification of cancer subtypes aims to detect patients with distinct molecular profiles and thus could provide new clues on effective clinical therapies. While great efforts have been made, it remains challenging to develop powerful computational methods that can efficiently integrate multi-omics datasets for the task. In this paper, we propose a novel self-supervised learning model called Deep Multi-view Contrastive Learning (DMCL) for cancer subtype identification. Specifically, by incorporating the reconstruction loss, contrastive loss and clustering loss into a unified framework, our model simultaneously encodes the sample discriminative information into the extracted feature representations and well preserves the sample cluster structures in the embedded space. Moreover, DMCL is an end-to-end framework where the cancer subtypes could be directly obtained from the model outputs. We compare DMCL with eight alternatives ranging from classic cancer subtype identification methods to recently developed state-of-the-art systems on 10 widely used cancer multi-omics datasets as well as an integrated dataset, and the experimental results validate the superior performance of our method. We further conduct a case study on liver cancer and the analysis results indicate that different subtypes might have different responses to the selected chemotherapeutic drugs.
更多查看译文
关键词
cancer subtype,multi-view,contrastive learning,clustering
AI 理解论文
溯源树
样例
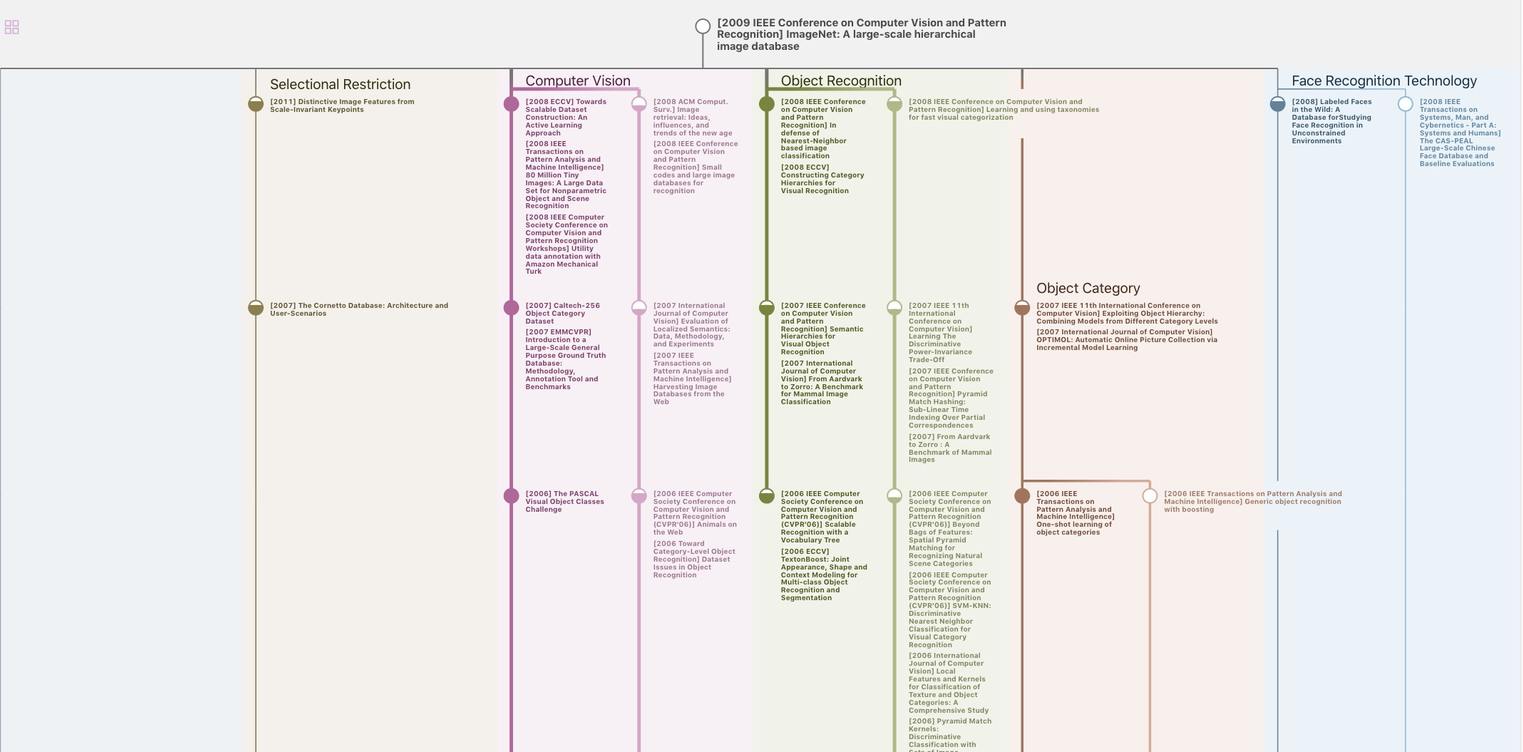
生成溯源树,研究论文发展脉络
Chat Paper
正在生成论文摘要