Multiscale Feature Fusion and Semi-Supervised Temporal-Spatial Learning for Performance Monitoring in the Flotation Industrial Process
IEEE TRANSACTIONS ON CYBERNETICS(2024)
摘要
This article studies the performance monitoring problem for the potassium chloride flotation process, which is a critical component of potassium fertilizer processing. To address its froth image segmentation problem, this article proposes a multiscale feature extraction and fusion network (MsFEFNet) to overcome the multiscale and weak edge characteristics of potassium chloride flotation froth images. MsFEFNet performs simultaneous feature extraction at multiple image scales and automatically learns spatial information of interest at each scale to achieve efficient multiscale information fusion. In addition, the potassium chloride flotation process is a multistage dynamic process with massive unlabeled data. To overcome its dynamic time-varying and working condition spatial similarity characteristics, a semi-supervised froth-grade prediction model based on a temporal-spatial neighborhood learning network combined with Mean Teacher (MT-TSNLNet) is proposed. MT-TSNLNet designs a new objective function for learning the temporal-spatial neighborhood structure of data. The introduction of Mean Teacher can further utilize unlabeled data to promote the proposed prediction model to better track the concentrate grade. To verify the effectiveness of the proposed MsFEFNet and MT-TSNLNet, froth image segmentation and grade prediction experiments are performed on a real-world potassium chloride flotation process dataset.
更多查看译文
关键词
Froth image segmentation,grade prediction,industrial performance monitoring,potassium chloride flotation process,semi-supervised temporal-spatial learning
AI 理解论文
溯源树
样例
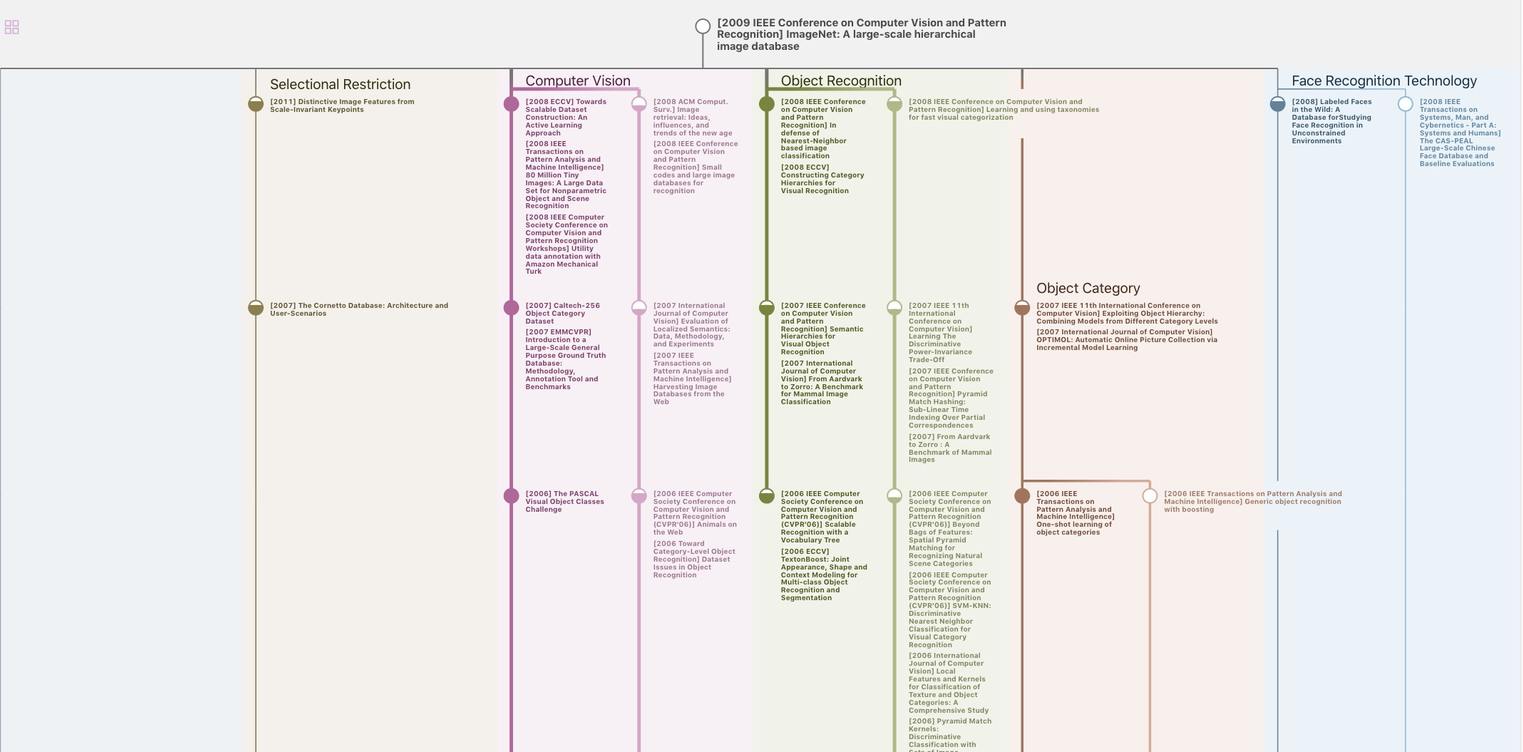
生成溯源树,研究论文发展脉络
Chat Paper
正在生成论文摘要