An artificial viscosity augmented physics-informed neural network for incompressible flow
Applied Mathematics and Mechanics(2023)
摘要
Physics-informed neural networks (PINNs) are proved methods that are effective in solving some strongly nonlinear partial differential equations (PDEs), e.g., Navier-Stokes equations, with a small amount of boundary or interior data. However, the feasibility of applying PINNs to the flow at moderate or high Reynolds numbers has rarely been reported. The present paper proposes an artificial viscosity (AV)-based PINN for solving the forward and inverse flow problems. Specifically, the AV used in PINNs is inspired by the entropy viscosity method developed in conventional computational fluid dynamics (CFD) to stabilize the simulation of flow at high Reynolds numbers. The newly developed PINN is used to solve the forward problem of the two-dimensional steady cavity flow at Re = 1 000 and the inverse problem derived from two-dimensional film boiling. The results show that the AV augmented PINN can solve both problems with good accuracy and substantially reduce the inference errors in the forward problem.
更多查看译文
关键词
physics-informed neural network(PINN),artificial viscosity(AV),cavity driven flow,high Reynolds number
AI 理解论文
溯源树
样例
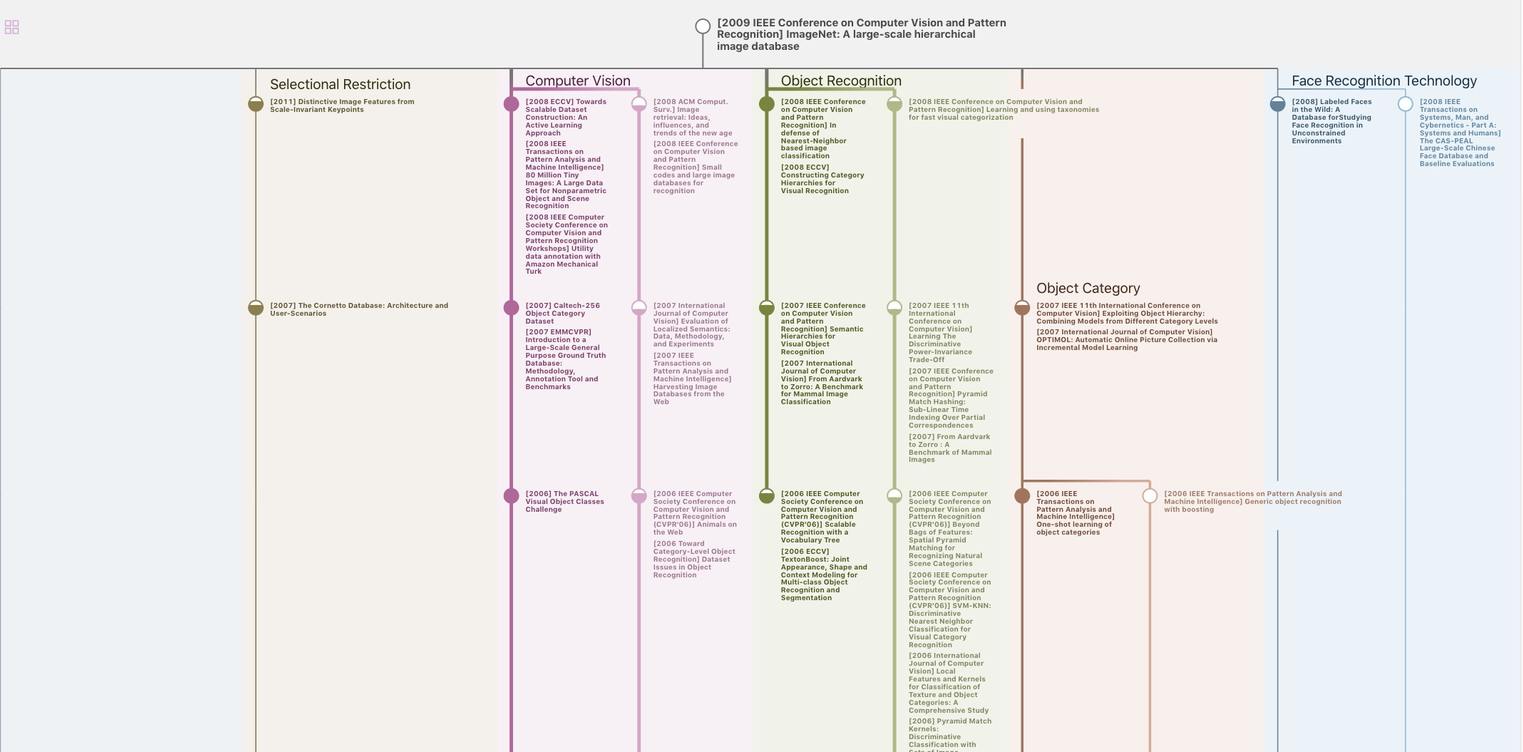
生成溯源树,研究论文发展脉络
Chat Paper
正在生成论文摘要