Deployment of Future Services in a Multi-access Edge Computing Environment Using Intelligence at the Edge
Journal of Network and Systems Management(2023)
摘要
The transition to beyond fifth generation (B5G) and sixth generation (6G) networks will lead to the support of new and future services, like virtual reality, vehicle collision avoidance or Internet-of-Things applications, among others. Such applications will be deployed based on loosely-coupled and independent microservices under different Quality of Service (QoS) requirements. Moreover, future applications will be either delay-tolerant or delay-sensitive, resulting in different application categories. Therefore, any provisioning technique will have to meet a large range of requirements, with flexibility and optimally, to accommodate the different service demands onto a common network. This is a major challenge for current networks, having to provide such services under strict QoS requirements, at minimum cost, and in a reasonable time. Currently, multi-access edge computing (MEC) has been proposed to deploy time-sensitive microservice-based applications, increasing the complexity of an optimal deployment in resource-constrained systems. Moreover, traditional heuristic algorithms do follow static solving strategies, resulting in sub-optimal provisioning decisions. To address this problem, we propose a combined heuristic and artificial intelligence technique that minimizes the cost of deployment at the edge of the network, optimally provisioning, in reasonable time, different types of applications with different requirements, to be done on single or multiple MECs, according to the category of the requested application and current MEC state features. Extensive simulation results show that the use of an intelligent algorithm significantly improves most of the performance metrics used, especially the minimization deployment cost, compared to other baseline techniques.
更多查看译文
关键词
Multi-access edge computing,Critical path,Deep reinforcement learning,Microservice composition,MSFs
AI 理解论文
溯源树
样例
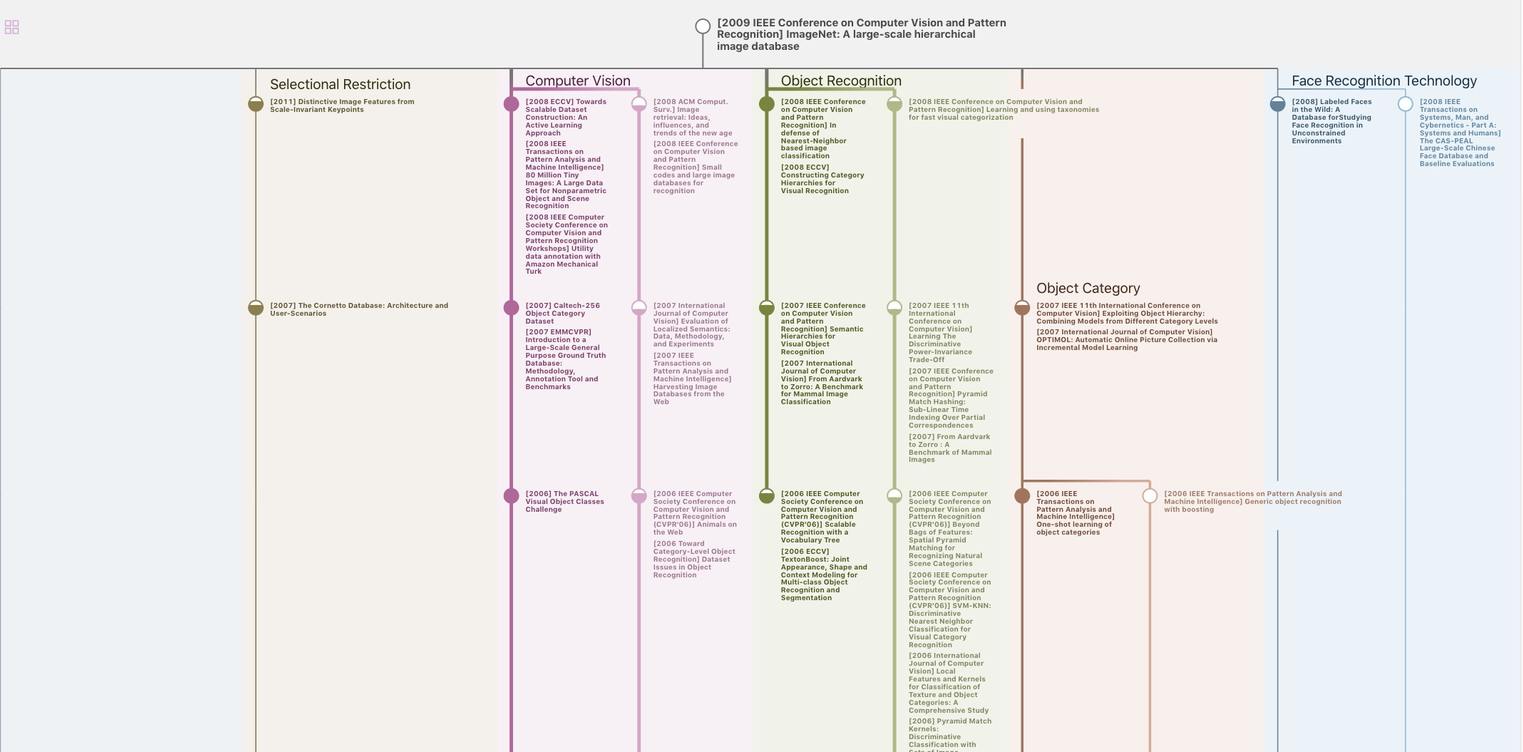
生成溯源树,研究论文发展脉络
Chat Paper
正在生成论文摘要