RF-Q: Unsupervised Signal Quality Assessment for Robust RF-based Respiration Monitoring.
CHASE(2023)
摘要
Continuous monitoring of respiration provides invaluable insights about health status management (e.g., the progression or recovery of diseases). Recent advancements in radio frequency (RF) technologies show promise for continuous respiration monitoring by virtue of their non-invasive nature, and preferred over wearable solutions that require frequent charging and continuous wearing. However, RF signals are susceptible to large body movements, which are inevitable in real life, challenging the robustness of respiration monitoring. While many existing methods have been proposed to achieve robust RF-based respiration monitoring, their reliance on supervised data limits their potential for broad applicability. In this context, we propose, RF-Q, an unsupervised/self-supervised model to achieve signal quality assessment and quality-aware estimation for robust RF-based respiration monitoring. RF-Q uses the reconstruction error of an autoencoder (AE) neural network to quantify the quality of respiratory information in RF signals without the need for data labeling. With the combination of the quantified signal quality and reconstructed signal in a weighted fusion, we are able to achieve improved robustness of RF respiration monitoring. We demonstrate that, instead of applying sophisticated models devised with respective expertise using a considerable amount of labeled data, by just quantifying the signal quality in an unsupervised manner we can significantly boost the average end-to-end (e2e) respiratory rate estimation accuracy of a baseline by an improvement ratio of 2.75, higher than the gain of 1.94 achieved by a supervised baseline method that excludes distorted data.
更多查看译文
关键词
RF sensing, signal quality assessment, vital signs monitoring, signal reconstruction, unsupervised learning, autoencoder (AE)
AI 理解论文
溯源树
样例
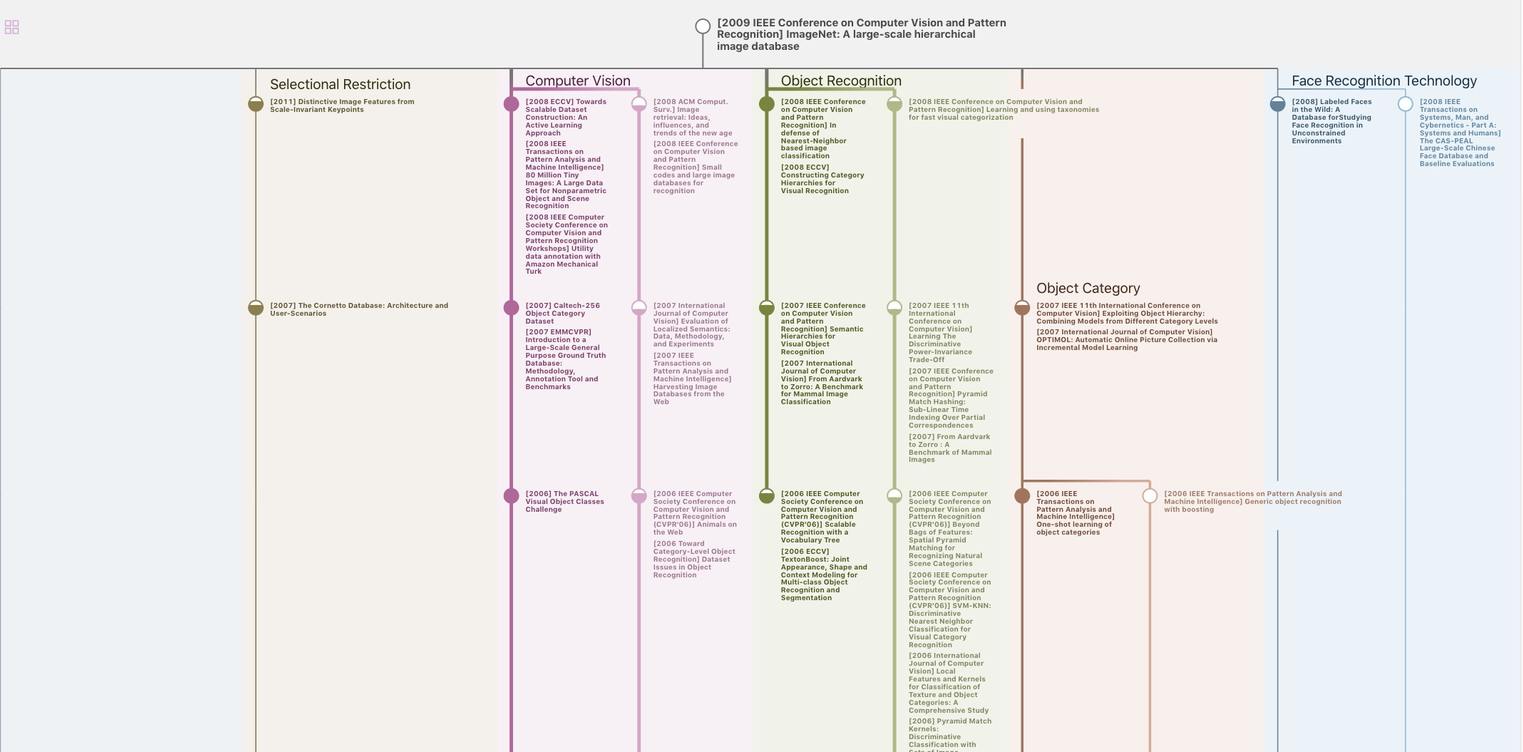
生成溯源树,研究论文发展脉络
Chat Paper
正在生成论文摘要