Short: Deep Learning Approach to Skeletal Performance Evaluation of Physical Therapy Exercises.
CHASE(2023)
摘要
At-home exercising strongly predicts physical therapy patient out-comes, underscoring the need for analyzing patient behaviors at-home via remote patient monitoring. Contemporary methods for remote patient monitoring rely on specialized sensors, i.e., Inertial Measurement Units, RGB-Depth cameras, motion capture systems, or stereo vision which are costly and not scalable to all physical therapy patients. Here, we observe a lack of literature using only a monocular RGB camera. In this paper, we demonstrate a skeletal feedback model for at-home exercises using only video acquired from a smartphone camera. We propose models for (i) Patient Performance Evaluation - which classifies the correctness of exercises, and (ii) Guidance - which identifies why the exercise went wrong so the patient can correct themselves. We use these models on our dataset of four common physical therapy exercises labeled by a physical therapist. Our results demonstrate the feasibility of using skeletal data from state-of-the-art 3D human pose estimation models for physical rehabilitation exercise evaluation and guidance. Thus, we enable remote patient monitoring and guidance from a single camera - making it highly cost-effective and scalable.
更多查看译文
关键词
PT rehabilitation,Patient Performance Evaluation,posture correction,deep learning,self attention,home monitoring
AI 理解论文
溯源树
样例
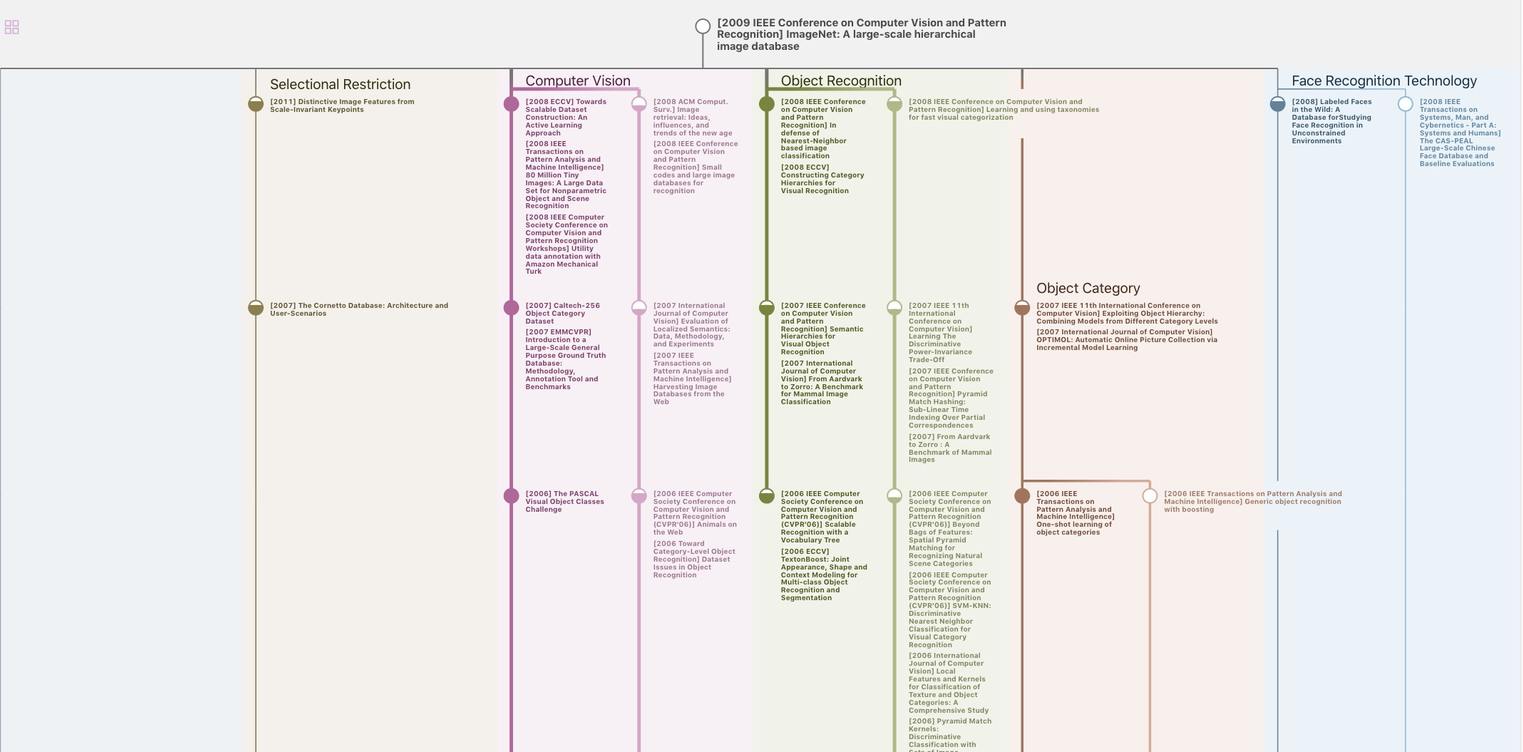
生成溯源树,研究论文发展脉络
Chat Paper
正在生成论文摘要