A/D Alleviator: Reducing Analog-to-Digital Conversions in Compute-In-Memory with Augmented Analog Accumulation.
ISCAS(2023)
摘要
Compute-in-memory (CIM) has shown great promise in accelerating numerous deep-learning tasks. However, existing analog CIM (ACIM) accelerators often suffer from frequent and energyintensive analog-to-digital (A/D) conversions, severely limiting their energy efficiency. This paper proposes A/D Alleviator, an energy-efficient augmented analog accumulation data flow to reduce A/D conversions in ACIM accelerators. Tomake it, switched-capacitor-based multiplication and accumulation circuits are used to connect the bitlines (BLs) of memory crossbar arrays and the finalA/Dconversion stage. In thisway, analog partial sums can be accumulated both spatially across all adjacent BLs that store high-precision weights and temporarily across all input cycles before the final quantization, thereby minimizing the need for explicit A/D conversions. Evaluations demonstrate that A/D Alleviator can improve energy efficiency by 4.9x and 1.9x with a high signal-to-noise ratio, as compared to state-of-the-art ACIM accelerators.
更多查看译文
关键词
CIM,A/D conversions,analog accumulation
AI 理解论文
溯源树
样例
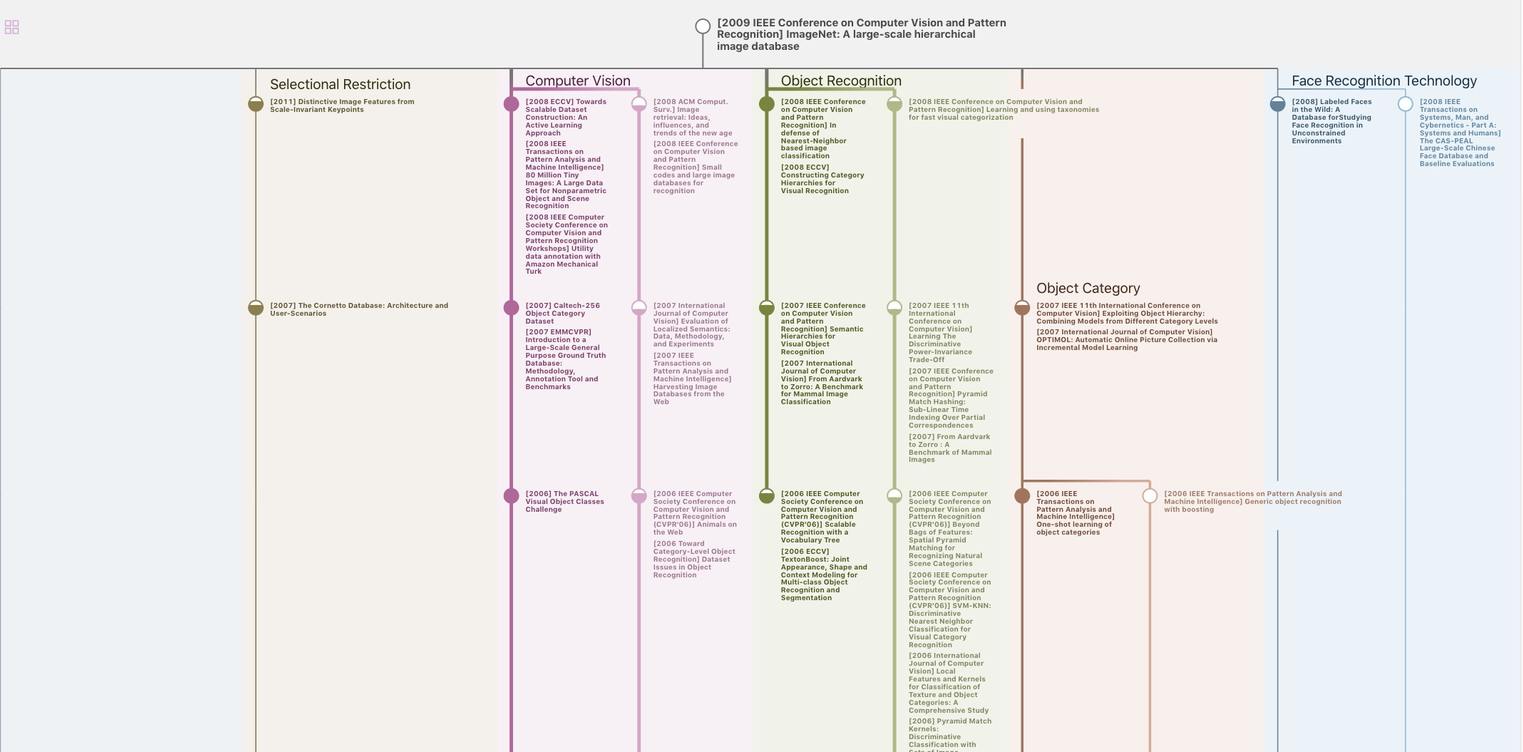
生成溯源树,研究论文发展脉络
Chat Paper
正在生成论文摘要