A $2.53 \mu \mathrm{W}/\text{channel}$ Event-Driven Neural Spike Sorting Processor with Sparsity-Aware Computing-In-Memory Macros.
ISCAS(2023)
摘要
Spike sorting processors with high energy efficiency are widely used in large-scale neural signal processing tasks to monitor the activity of neurons in brains. This paper presents a low-power processor for high-accuracy spike sorting and on-chip incremental learning using an algorithm-hardware co-design approach. The processor introduces an event-driven mechanism with adaptive-threshold detection to conditionally activate the system in order to reduce power consumption. Sparsity-aware computing-in-memory (CIM) macros are also developed in our design to store templates and perform complicated computations efficiently. The prototype is designed using 28nm technology with an area of 0.018 mm
2
/channel and an overall power efficiency of
$\mathbf{2.53} \mu \mathbf{W}/\mathbf{channel}$
and 84nW/(channel.cluster) at the voltage of 0.72V. Moreover, the accuracy of the whole design can reach 94.5% in a 32-channel scenario.
更多查看译文
关键词
spike sorting,template matching,on-chip learning,event-driven,adaptive threshold,computing-in-memory,sparsity,low power
AI 理解论文
溯源树
样例
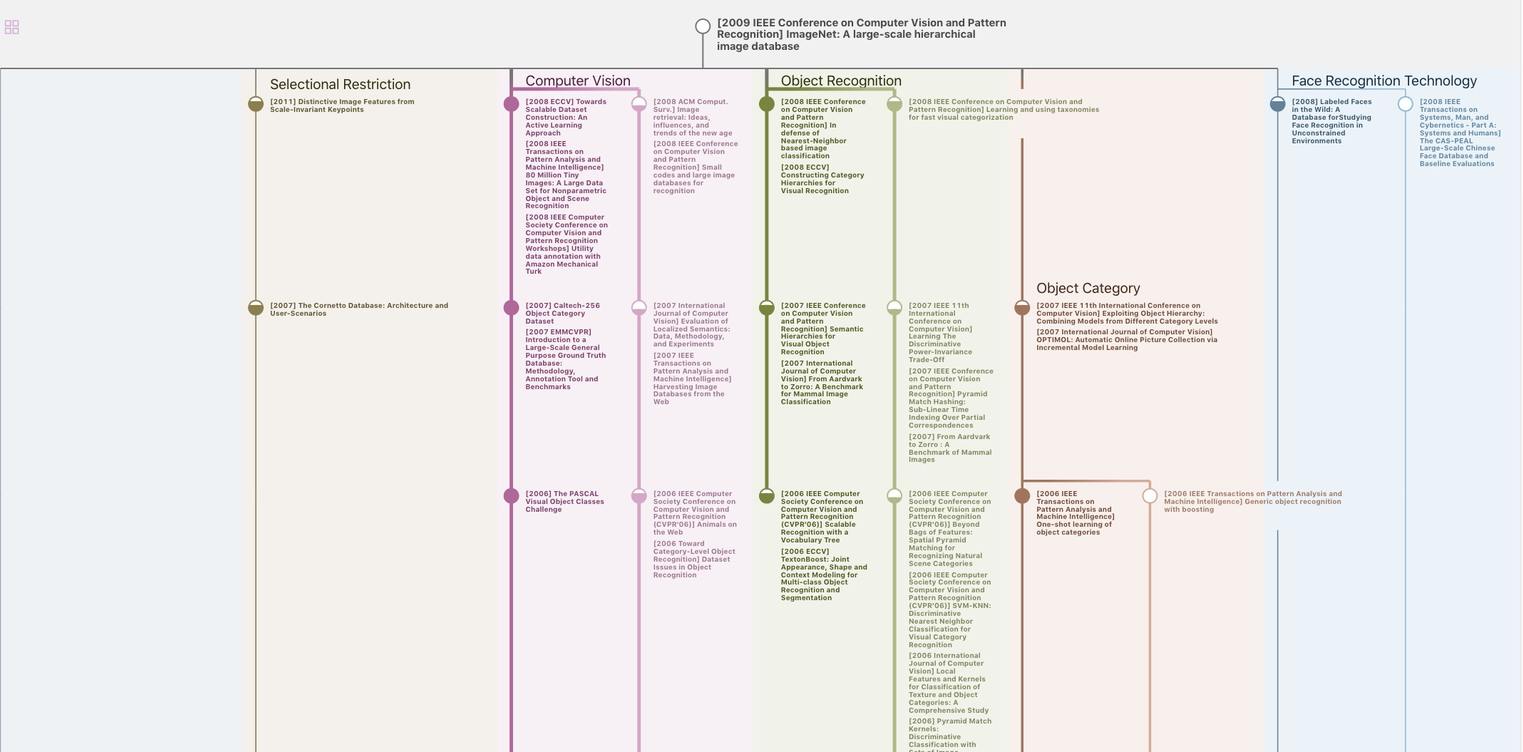
生成溯源树,研究论文发展脉络
Chat Paper
正在生成论文摘要