Extreme Generative Human-Oriented Video Coding via Motion Representation Compression.
ISCAS(2023)
摘要
The increasing popularity of video conferencing and live streaming raises the growing demand for encoding human-oriented videos at ultra-low bit rates. Recently, several ultra-low bitrate video codecs have proposed using inter-frame keypoints or landmarks to derive motion representations, which are then used to warp decoded frames in a generative manner. Despite its success, compression of the motion representation has been less investigated in the literature. In this work, we propose a novel principal component analysis (PCA)-based decomposing method to fully exploit the compression potential of motion representations. In particular, we decompose the derived motion affine matrices into three parts and apply quantization and entropy estimation to each part in a different way depending on its significance. Using such compressed-friendly motion representations allows for preserving most of the motion information and achieving lower coding costs. Extensive qualitatively and quantitatively experimental results on the human video datasets demonstrate the superiority of the proposed paradigm over existing video codecs under extreme compression ratios.
更多查看译文
关键词
Generative compression, human video, motion compression, ultra-low bitrate
AI 理解论文
溯源树
样例
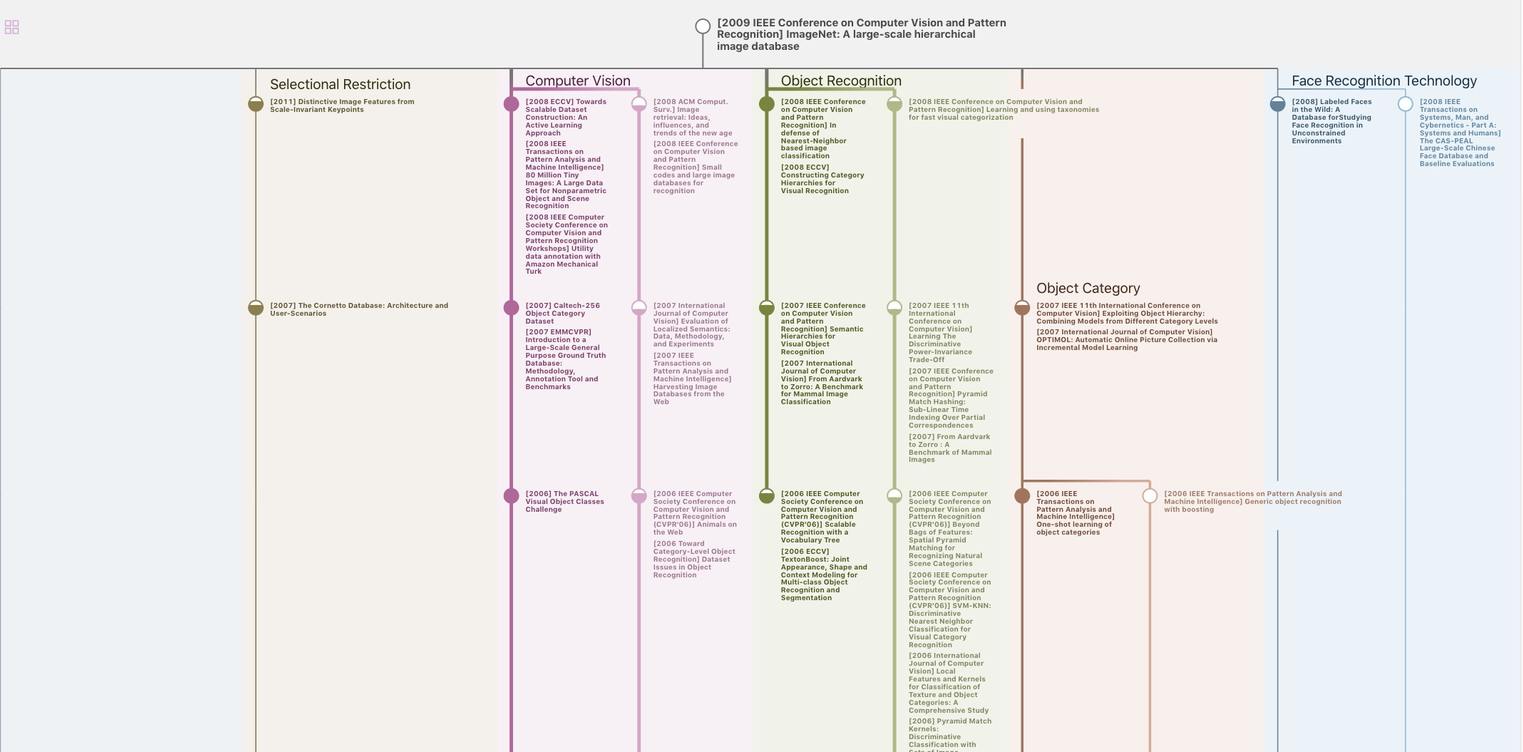
生成溯源树,研究论文发展脉络
Chat Paper
正在生成论文摘要