Energy-Efficient Stochastic Computing for Convolutional Neural Networks by Using Kernel-wise Parallelism.
ISCAS(2023)
摘要
Stochastic computing (SC) is a low-cost computation paradigm that can replace conventional binary arithmetic to provide a low hardware footprint with high scalability. However, since the SC bitstream length grows with the precision of the represented data, regardless of its lower power consumption, the convolutional SC-based neural networks may not be efficient in hardware area and energy. This work proposes a novel SC accelerator, PSC-Conv, to implement the convolutional layer using a new binary-interfaced stochastic computing architecture. PSC-Conv exploits kernel-wise parallelism in CNNs, reducing hardware footprint and energy consumption. Experimental results show that the proposed implementation excels among several state-of-the-art SC-based implementations regarding area and power efficiency. We also compared the implementations of three modern CNNs, including LeNet-5, MobileNet, and ResNet-50. Experimental results demonstrate that, on average, PSC-Conv can achieve 5.02x speedup and 87.9% energy reduction compared with the binary implementation.
更多查看译文
关键词
Stochastic computing, Hardware accelerator, Convolutional Neural Networks, Kernel-wise parallelism
AI 理解论文
溯源树
样例
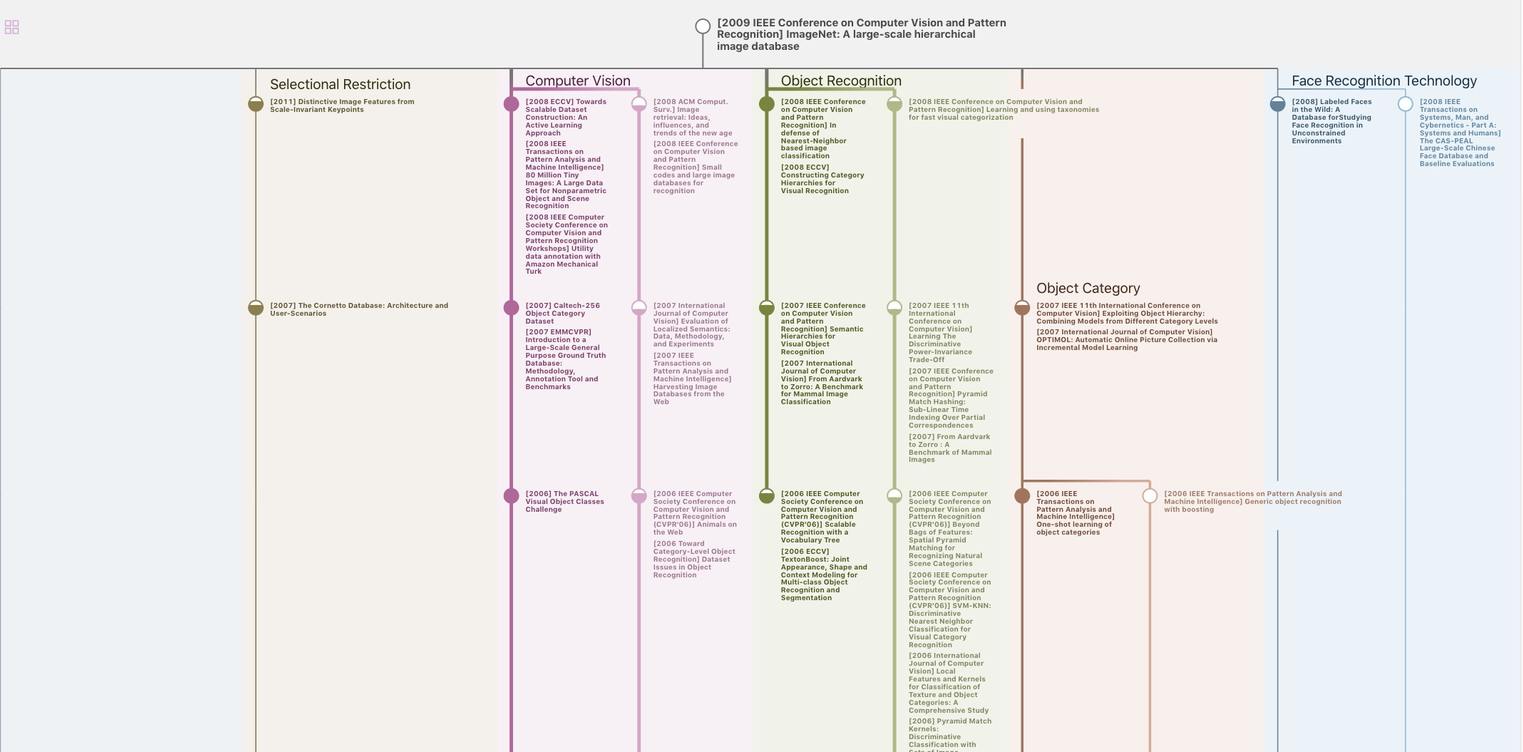
生成溯源树,研究论文发展脉络
Chat Paper
正在生成论文摘要