Low-Power Real-Time Sequential Processing with Spiking Neural Networks.
ISCAS(2023)
摘要
The biological brain is capable of processing temporal information at an incredible efficiency. Even with modern computing resources, traditional learning-based approaches are struggling to match its performance. Spiking neural networks that "mimic" certain functionalities of the biological neural networks in the brain is a promising avenue for solving sequential learning problems with high computational efficiency. Nonetheless, training such networks still remains a challenging task as conventional learning rules are not directly applicable to these bio-inspired neural networks. Recent efforts have focused on novel training paradigms that allow spiking neural networks to learn temporal correlations between inputs and solve sequential tasks such as audio or video processing. Such success has fueled the development of event-driven neuromorphic hardware that is specifically optimized for energy-efficient implementation of spiking neural networks. This paper highlights the ongoing development of spiking neural networks for low-power real-time sequential processing and the potential to improve their training through an understanding of the information flow.
更多查看译文
关键词
bio-inspired neural networks,biological brain,biological neural networks,computational efficiency,energy-efficient implementation,event-driven neuromorphic hardware,low-power real-time sequential processing,sequential learning problems,spiking neural networks
AI 理解论文
溯源树
样例
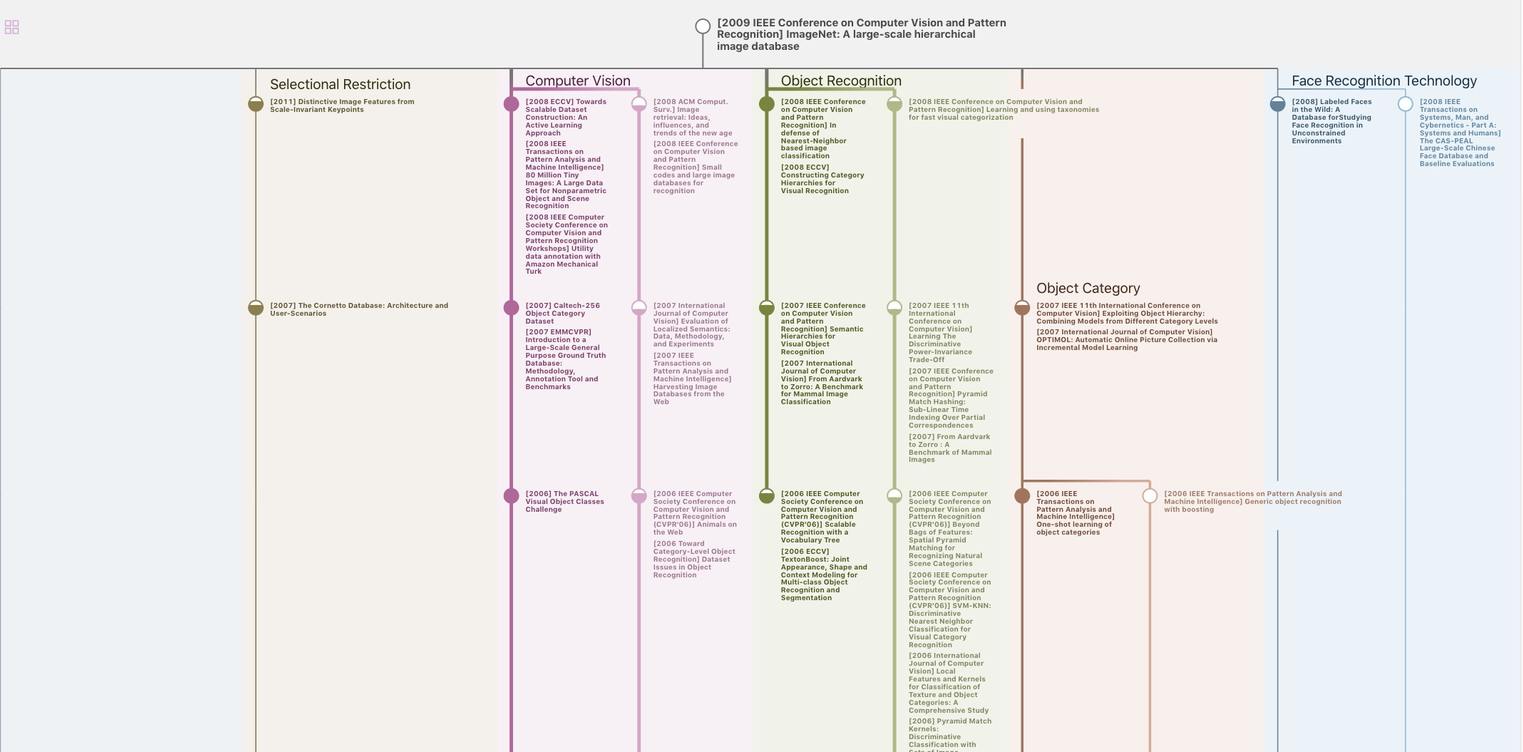
生成溯源树,研究论文发展脉络
Chat Paper
正在生成论文摘要