Designing Medium Access Control Protocol Sequences Through Deep Reinforcement Learning
EuCNC/6G Summit(2023)
摘要
This work aims to design protocol sequences through deep reinforcement learning (DRL). Protocol sequences are periodic binary sequences that define multiple access control among users, introduced for systems considering collision channel without feedback (CCw/oFB). In this paper, we leverage the recent advancement of DRL methods to design protocol sequences with desirable new properties, namely Throughput Maximizing User- Irrepressible (TMUI) sequences. TMUI has two specific properties: (i) user-irrepressibility (UI), and (ii) maximizing the minimum individual throughput among the users. We assumed that the transmission channel is divided into time slots and the starting time of each user in joining the system is arbitrary such that there exist random relative time offsets. We use a DRL approach to find TMUI sequences. We report the obtained TMUI protocol sequences and conduct numerical studies comparing TMUI against slotted ALOHA. Simulation results also show that the new medium access control (MAC) protocol does hold the UI property and can achieve substantially higher minimum individual user throughput, under the same system parameters.
更多查看译文
关键词
MAC, deep reinforcement learning (DRL), collision channel without feedback, protocol sequence, TMUI
AI 理解论文
溯源树
样例
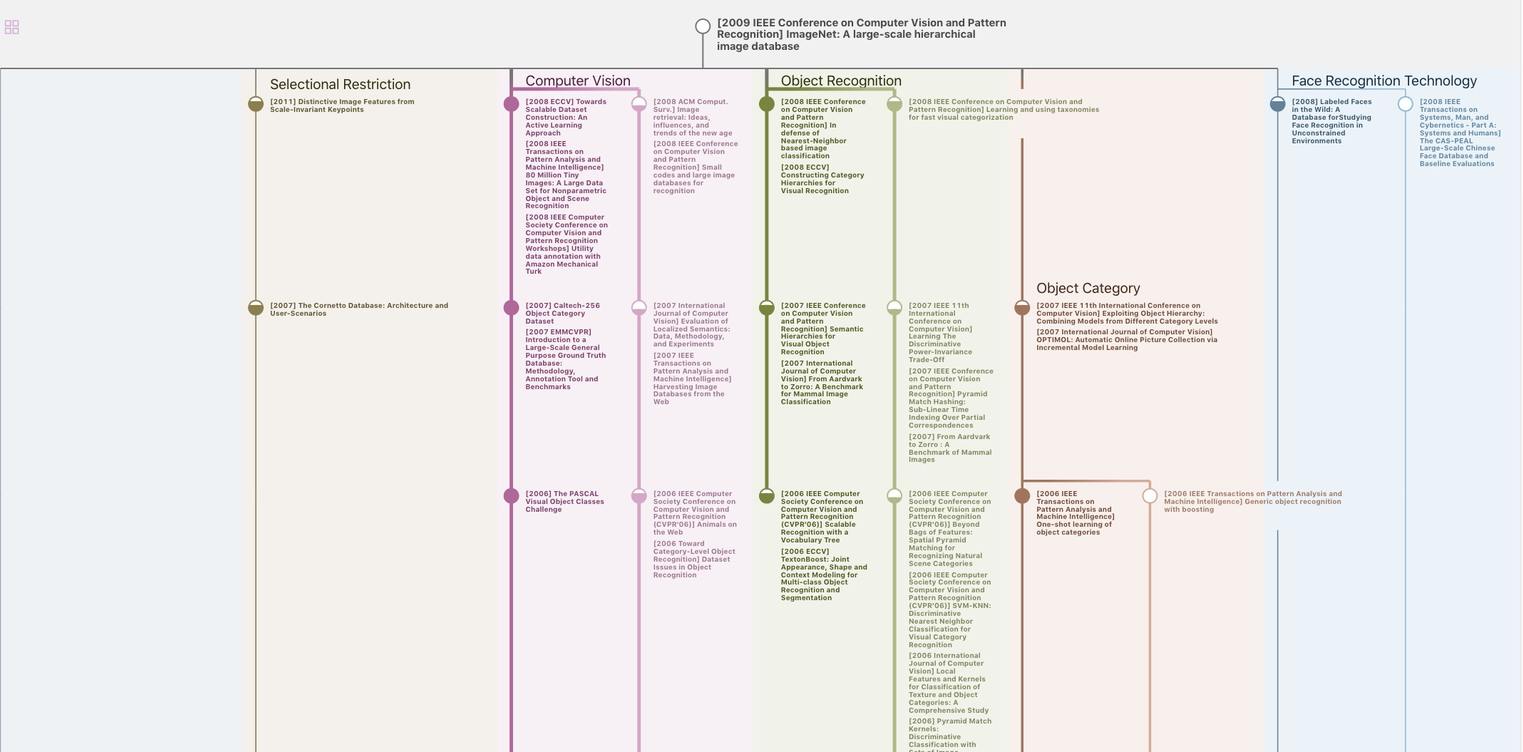
生成溯源树,研究论文发展脉络
Chat Paper
正在生成论文摘要