Residual Service Time Optimization for legacy Wireless-TSN end nodes.
WiMob(2023)
摘要
The emergence of Time-Sensitive Networking (TSN) has enabled network determinism to a new level, offering high reliability and bounded latency for critical communications. However, the unpredictable nature of traffic generation also poses new challenges to TSN. While TSN is designed to maintain backward compatibility with the 802.1 standards, many end nodes may not be equipped to understand TSN. This can result in a less deterministic TSN, and suboptimal resource utilization, mainly driven by Residual Service Time (RST). To address these challenges, this study proposes three scheduling mechanisms to reduce RST: q-learning, active time slot update, and polynomial forecasting. Real-world data captured from our wireless-TSN (WTSN) evaluation kit is used to compare the proposed approaches in terms of one-way latency. The results show that the machine learning approach outperforms the other methods in terms of overall latency. However, it is less effective in identifying the optimal time slot position compared to the other methods.
更多查看译文
关键词
wireless time-sensitive networking, scheduling, machine learning, reinforcement learning, residual service time
AI 理解论文
溯源树
样例
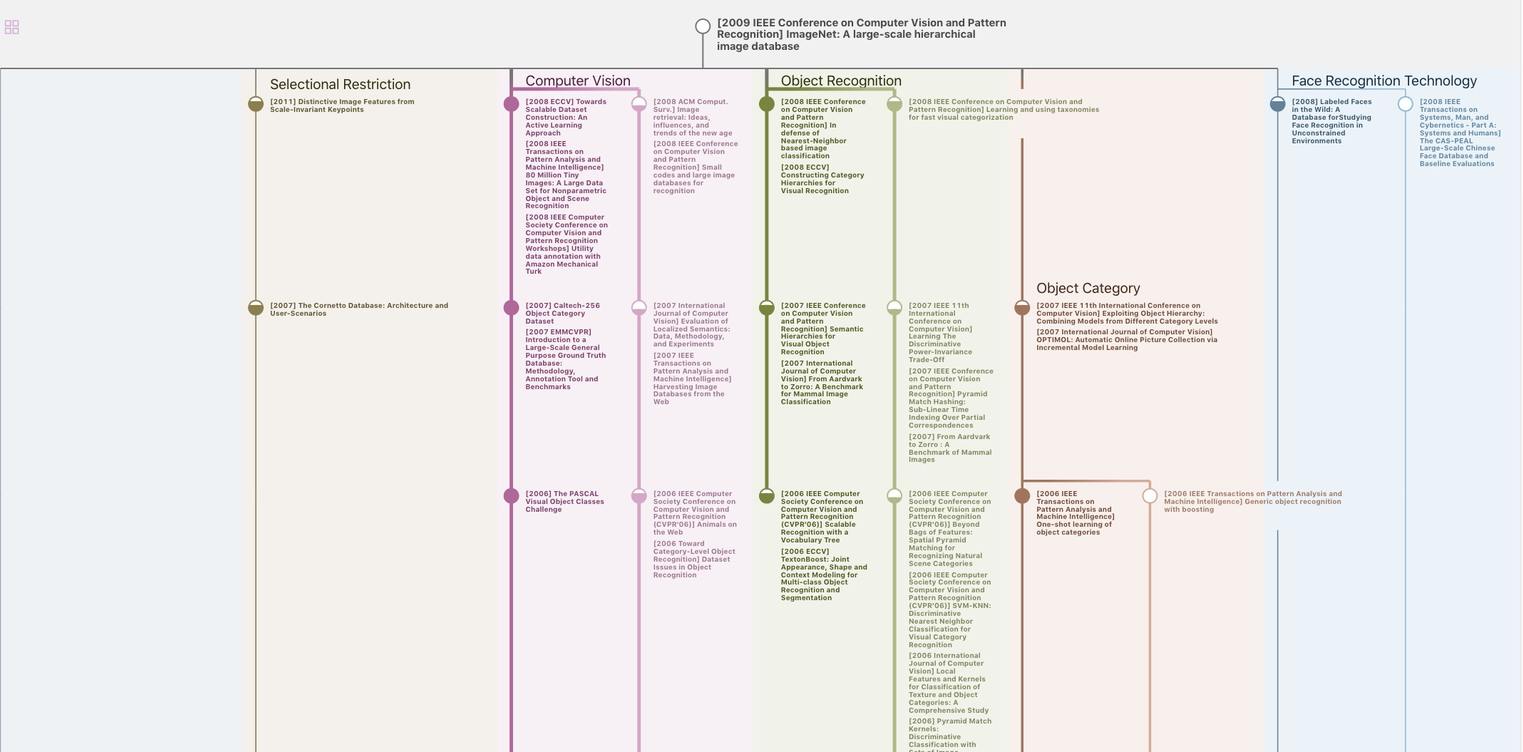
生成溯源树,研究论文发展脉络
Chat Paper
正在生成论文摘要