Evaluating Machine Learning Techniques for Predicting Link Instability in Wireless Networks to Support Live Video Streaming.
WiMob(2023)
摘要
This paper evaluates various Machine Learning (ML) techniques for classifying or predicting link instability, which we define as sudden increases in application-level packet loss and latency, or decreases in available bandwidth over a short, pre-determined period of time. To train and evaluate these ML techniques, we first construct attributes from raw wireless LTE traces. These attributes include statistical summaries (e.g., average and standard deviation) and trends (e.g., slope and correlation) of various network metrics. We then determine the attributes that provide the greatest predictive ability to construct our ML examples for training and testing. Using these ML examples, we evaluate the predictive capability of various ML techniques. We find that we can classify 5%-46% of sudden bandwidth drops, 40%-100% of increases in application-level packet loss, and 40%-100% of spikes in latency while maintaining false positive rates below 0.1. In general, we find that Decision Trees and K-Nearest Neighbours techniques provide the highest classification rate while incurring low false positive rates.
更多查看译文
关键词
application-level packet loss,decision trees,K-nearest neighbours techniques,link instability prediction,live video streaming,machine learning techniques,ML examples,ML techniques,network metrics,predictive ability,raw wireless LTE traces,sudden bandwidth drops,wireless networks
AI 理解论文
溯源树
样例
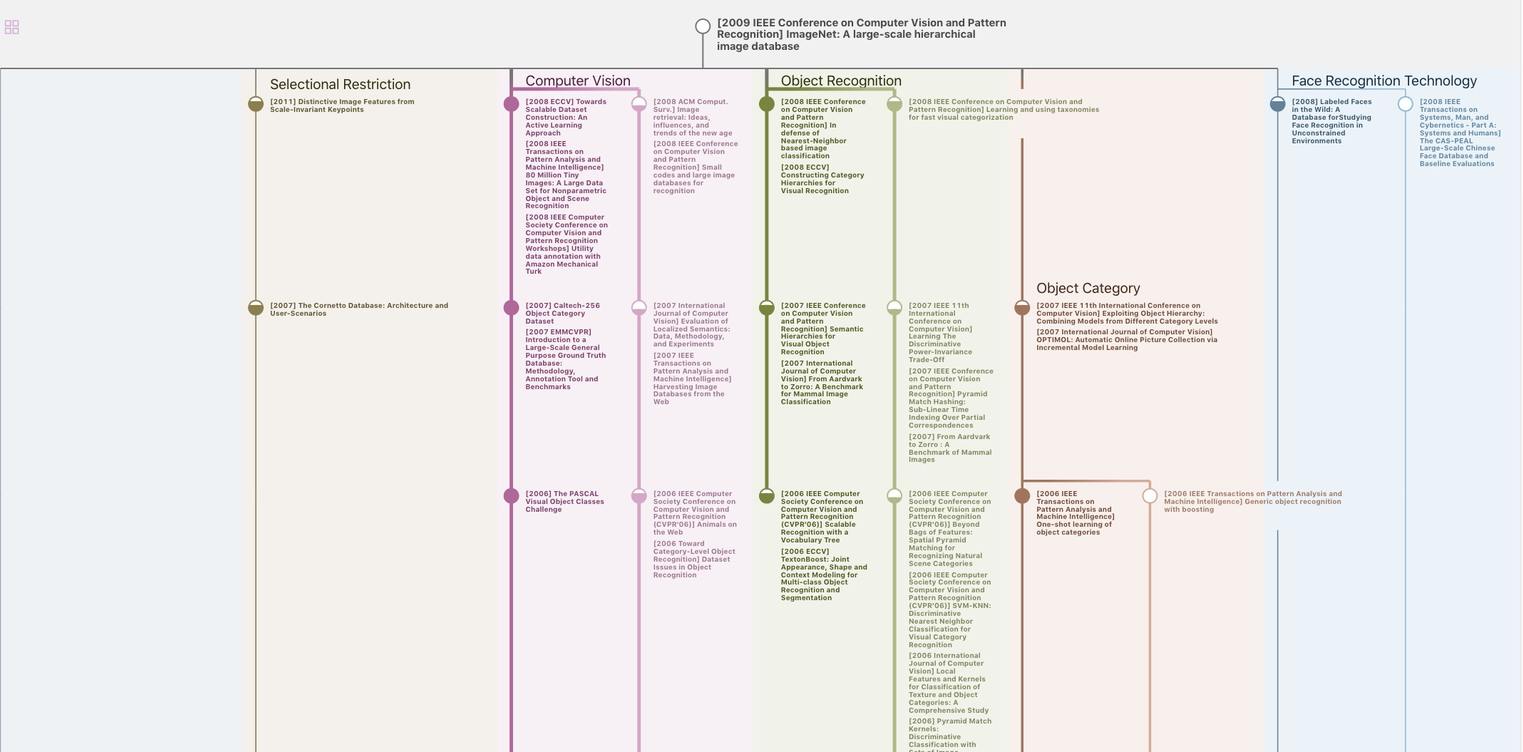
生成溯源树,研究论文发展脉络
Chat Paper
正在生成论文摘要