Gradient-Adaptive Pareto Optimization for Constrained Reinforcement Learning.
AAAI(2023)
摘要
Constrained Reinforcement Learning (CRL) burgeons broad interest in recent years, which pursues maximizing long-term returns while constraining costs. Although CRL can be cast as a multi-objective optimization problem, it is still facing the key challenge that gradient-based Pareto optimization methods tend to stick to known Pareto-optimal solutions even when they yield poor returns (e.g., the safest self-driving car that never moves) or violate the constraints (e.g., the record-breaking racer that crashes the car). In this paper, we propose Gradient-adaptive Constrained Policy Optimization (GCPO for short), a novel Pareto optimization method for CRL with two adaptive gradient recalibration techniques. First, to find Pareto-optimal solutions with balanced performance over all targets, we propose gradient rebalancing which forces the agent to improve more on under-optimized objectives at every policy iteration. Second, to guarantee that the cost constraints are satisfied, we propose gradient perturbation that can temporarily sacrifice the returns for costs. Experiments on the SafetyGym benchmarks show that our method consistently outperforms previous CRL methods in reward while satisfying the constraints.
更多查看译文
关键词
constrained reinforcement learning,reinforcement learning,pareto,optimization,gradient-adaptive
AI 理解论文
溯源树
样例
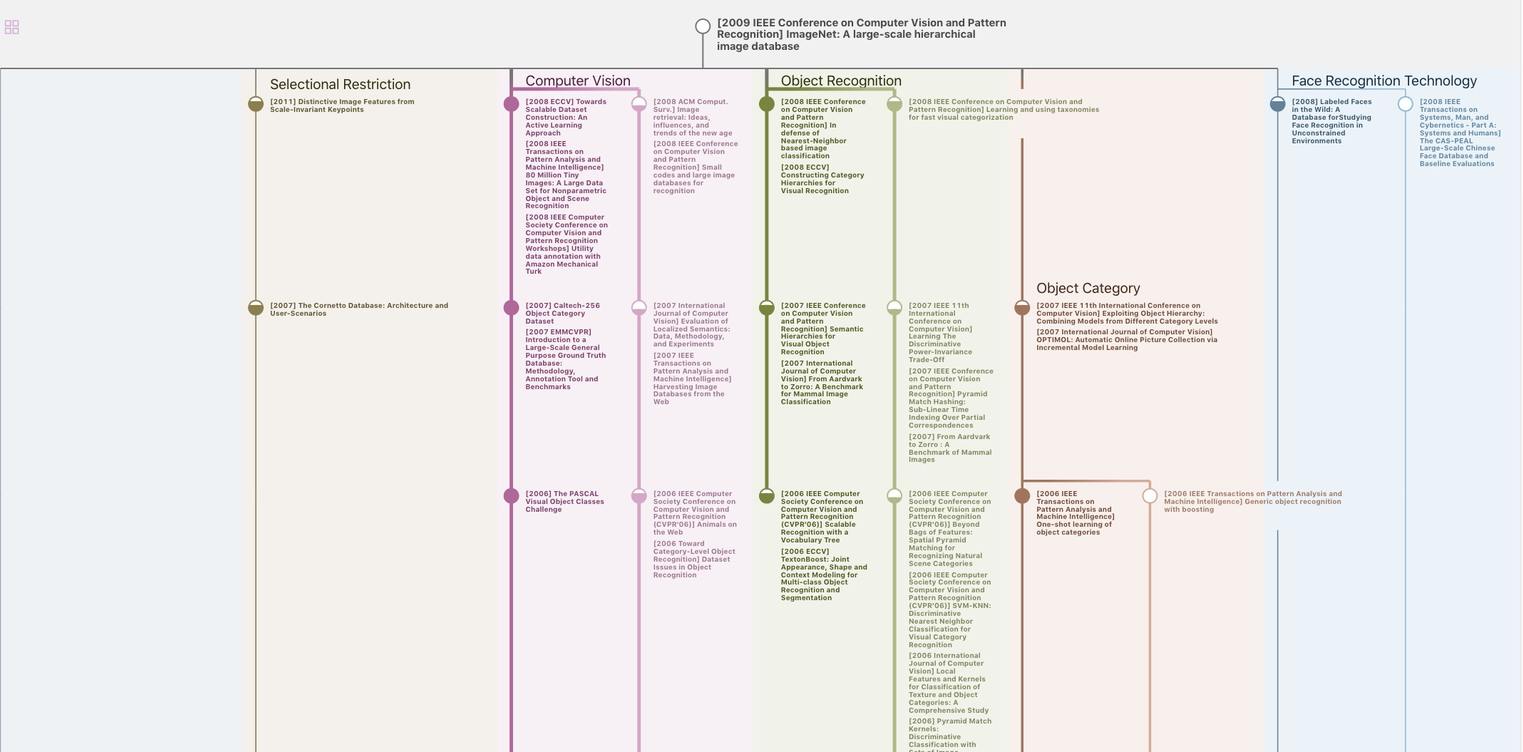
生成溯源树,研究论文发展脉络
Chat Paper
正在生成论文摘要