Out-of-Distribution Generalization by Neural-Symbolic Joint Training.
AAAI(2023)
摘要
This paper develops a novel methodology to simultaneously learn a neural network and extract generalized logic rules. Different from prior neural-symbolic methods that require background knowledge and candidate logical rules to be provided, we aim to induce task semantics with minimal priors. This is achieved by a two-step learning framework that iterates between optimizing neural predictions of task labels and searching for a more accurate representation of the hidden task semantics. Notably, supervision works in both directions: (partially) induced task semantics guide the learning of the neural network and induced neural predictions admit an improved semantic representation. We demonstrate that our proposed framework is capable of achieving superior out-of-distribution generalization performance on two tasks: (i) learning multi-digit addition, where it is trained on short sequences of digits and tested on long sequences of digits; (ii) predicting the optimal action in the Tower of Hanoi, where the model is challenged to discover a policy independent of the number of disks in the puzzle.
更多查看译文
关键词
generalization,joint,out-of-distribution,neural-symbolic
AI 理解论文
溯源树
样例
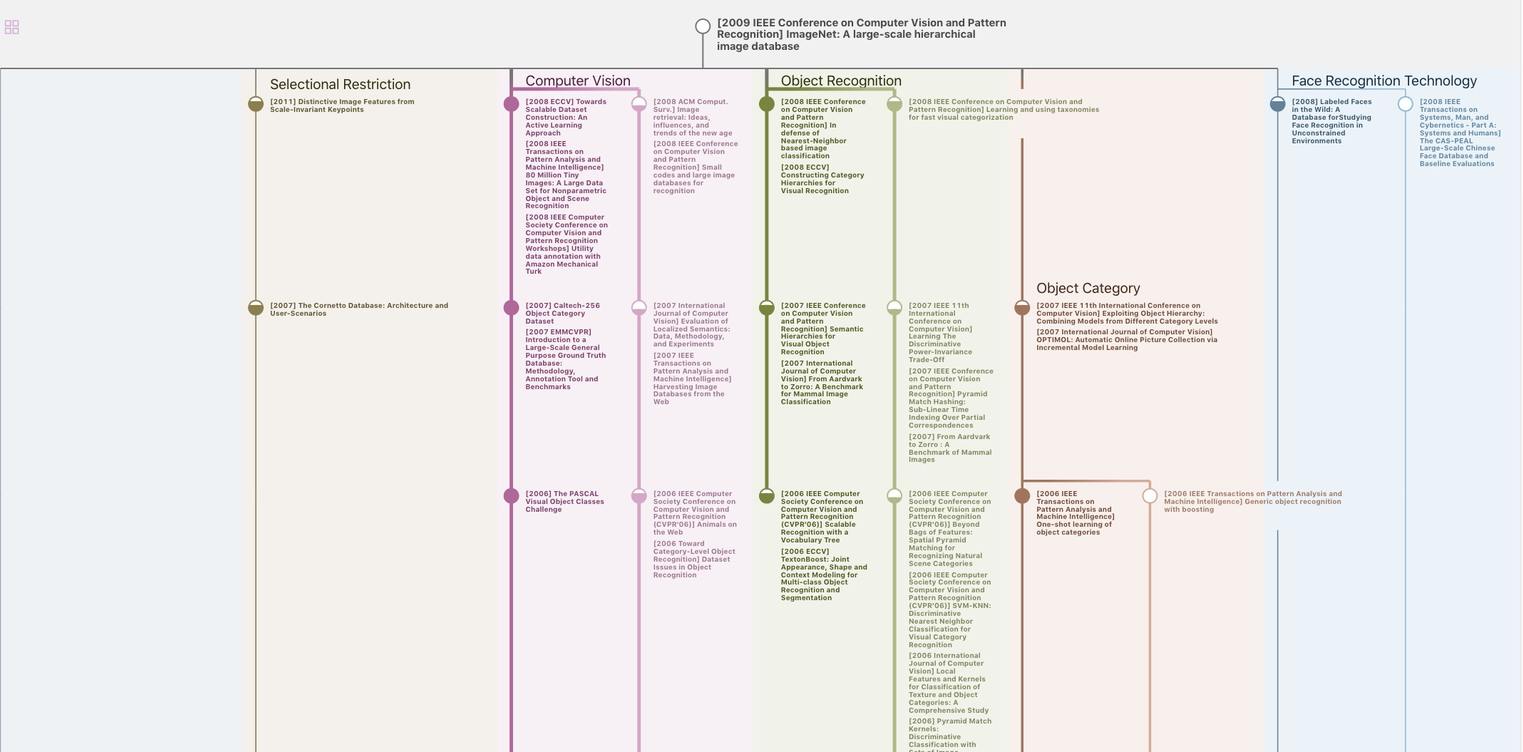
生成溯源树,研究论文发展脉络
Chat Paper
正在生成论文摘要