Priori Anchor Labels Supervised Scalable Multi-View Bipartite Graph Clustering.
AAAI(2023)
摘要
Although multi-view clustering (MVC) has achieved remarkable performance by integrating the complementary information of views, it is inefficient when facing scalable data. Proverbially, anchor strategy can mitigate such a challenge a certain extent. However, the unsupervised dynamic strategy usually cannot obtain the optimal anchors for MVC. The main reasons are that it does not consider the fairness of different views and lacks the priori supervised guidance. To completely solve these problems, we first propose the priori anchor graph regularization (PAGG) for scalable multi-view bipartite graph clustering, dubbed as SMGC method. Specifically, SMGC learns a few representative consensus anchors to simulate the numerous view data well, and constructs a bipartite graph to bridge the affinities between the anchors and original data points. In order to largely improve the quality of anchors, PAGG predefines prior anchor labels to constrain the anchors with discriminative cluster structure and fair view allocation, such that a better bipartite graph can be obtained for fast clustering. Experimentally, abundant of experiments are accomplished on six scalable benchmark datasets, and the experimental results fully demonstrate the effectiveness and efficiency of our SMGC.
更多查看译文
关键词
clustering,multi-view
AI 理解论文
溯源树
样例
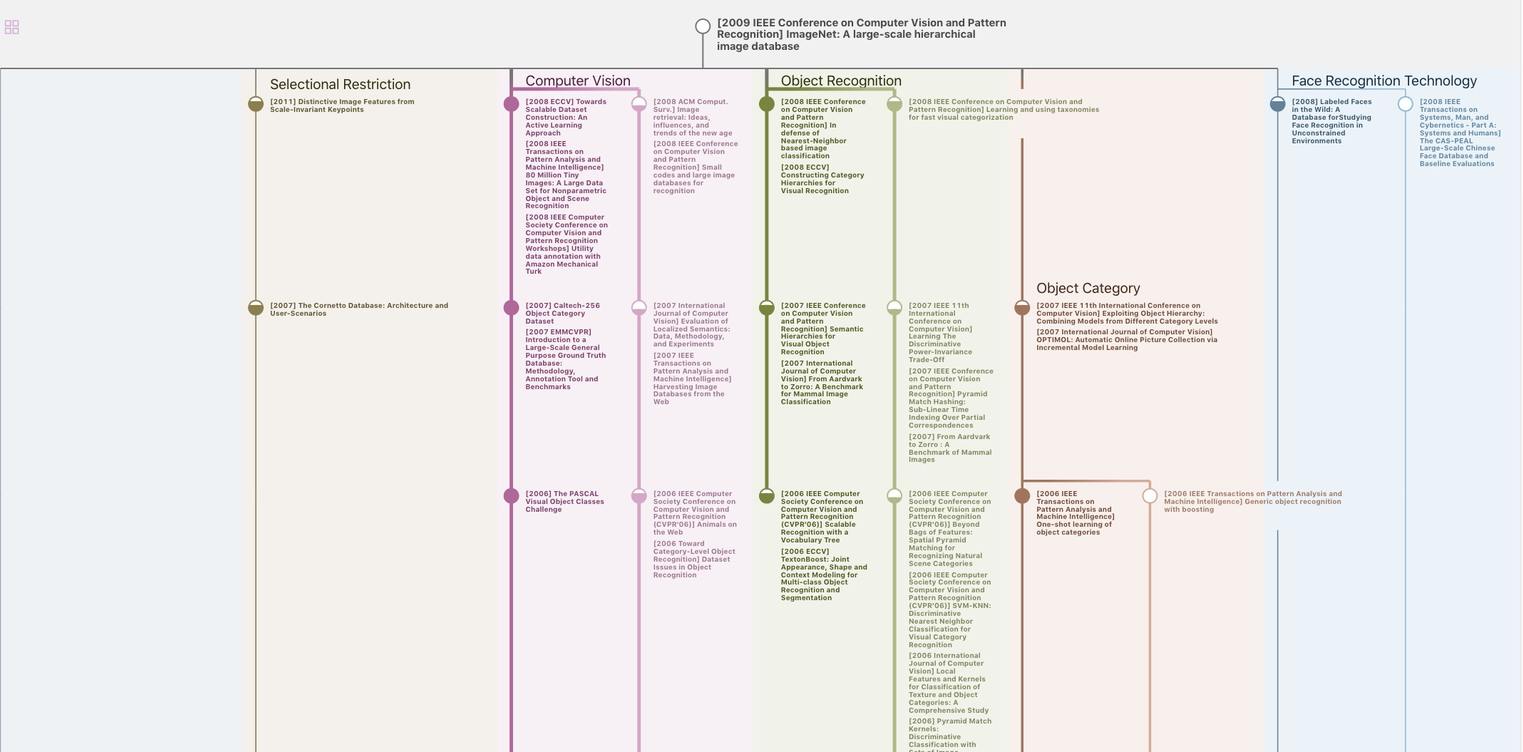
生成溯源树,研究论文发展脉络
Chat Paper
正在生成论文摘要