Multi-View Domain Adaptive Object Detection on Camera Networks.
AAAI(2023)
摘要
In this paper, we study a new domain adaptation setting on camera networks, namely Multi-View Domain Adaptive Object Detection (MVDA-OD), in which labeled source data is unavailable in the target adaptation process and target data is captured from multiple overlapping cameras. In such a challenging context, existing methods including adversarial training and self-training fall short due to multi-domain data shift and the lack of source data. To tackle this problem, we propose a novel training framework consisting of two stages. First, we pre-train the backbone using self-supervised learning, in which a multi-view association is developed to construct an effective pretext task. Second, we fine-tune the detection head using robust self-training, where a tracking-based single-view augmentation is introduced to achieve weak-hard consistency learning. By doing so, an object detection model can take advantage of informative samples generated by multi-view association and single-view augmentation to learn discriminative backbones as well as robust detection classifiers. Experiments on two real-world multicamera datasets demonstrate significant advantages of our approach over the state-of-the-art domain adaptive object detection methods.
更多查看译文
关键词
camera networks,detection,multi-view
AI 理解论文
溯源树
样例
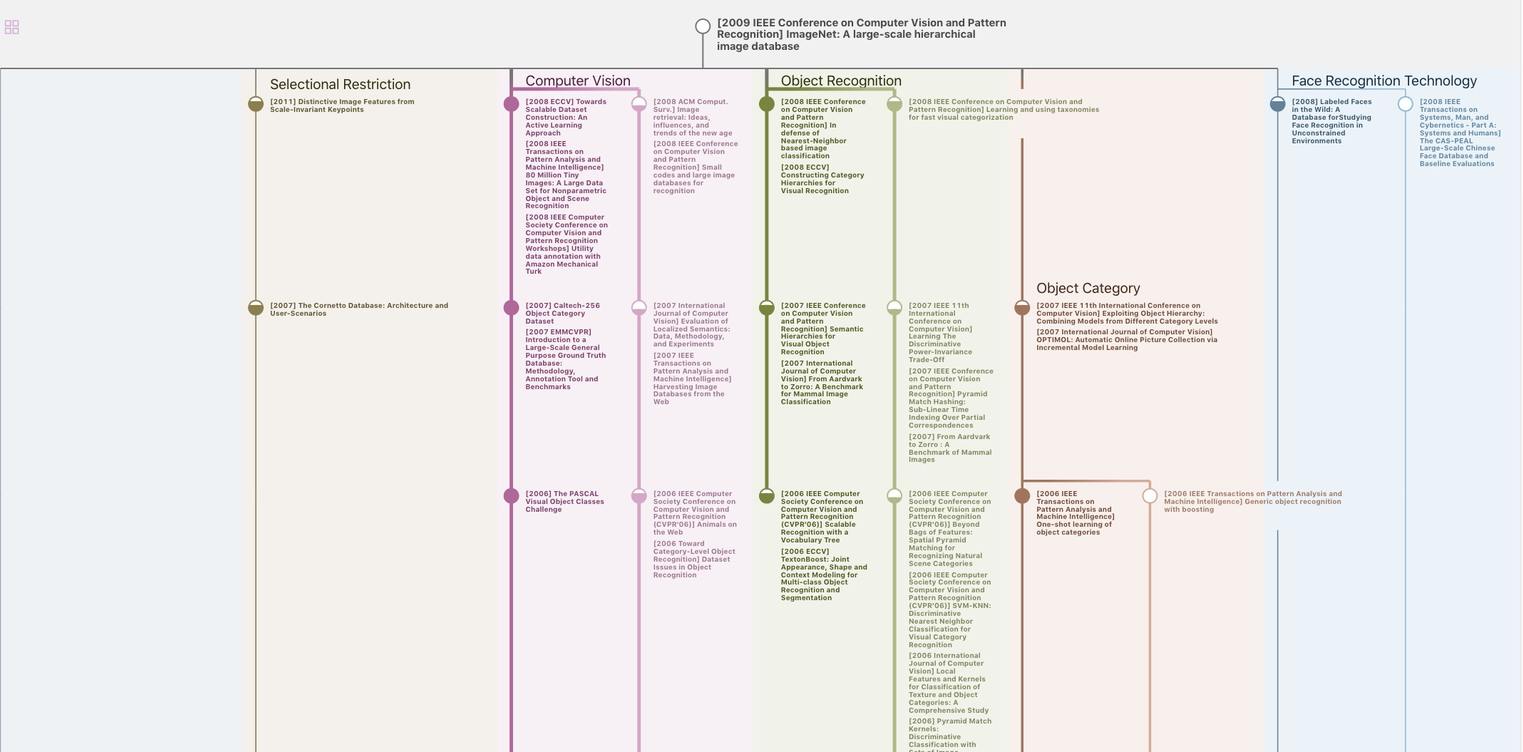
生成溯源树,研究论文发展脉络
Chat Paper
正在生成论文摘要