Let the Data Choose: Flexible and Diverse Anchor Graph Fusion for Scalable Multi-View Clustering.
AAAI(2023)
摘要
In the past few years, numerous multi-view graph clustering algorithms have been proposed to enhance the clustering performance by exploring information from multiple views. Despite the superior performance, the high time and space expenditures limit their scalability. Accordingly, anchor graph learning has been introduced to alleviate the computational complexity. However, existing approaches can be further improved by the following considerations: (i) Existing anchor-based methods share the same number of anchors across views. This strategy violates the diversity and flexibility of multi-view data distribution. (ii) Searching for the optimal anchor number within hyper-parameters takes much extra tuning time, which makes existing methods impractical. (iii) How to flexibly fuse multi-view anchor graphs of diverse sizes has not been well explored in existing literature. To address the above issues, we propose a novel anchor-based method termed Flexible and Diverse Anchor Graph Fusion for Scalable Multi-view Clustering (FDAGF) in this paper. Instead of manually tuning optimal anchor with massive hyper-parameters, we propose to optimize the contribution weights of a group of pre-defined anchor numbers to avoid extra time expenditure among views. Most importantly, we propose a novel hybrid fusion strategy for multi-size anchor graphs with theoretical proof, which allows flexible and diverse anchor graph fusion. Then, an efficient linear optimization algorithm is proposed to solve the resultant problem. Comprehensive experimental results demonstrate the effectiveness and efficiency of our proposed framework.
更多查看译文
关键词
diverse anchor graph fusion,multi-view
AI 理解论文
溯源树
样例
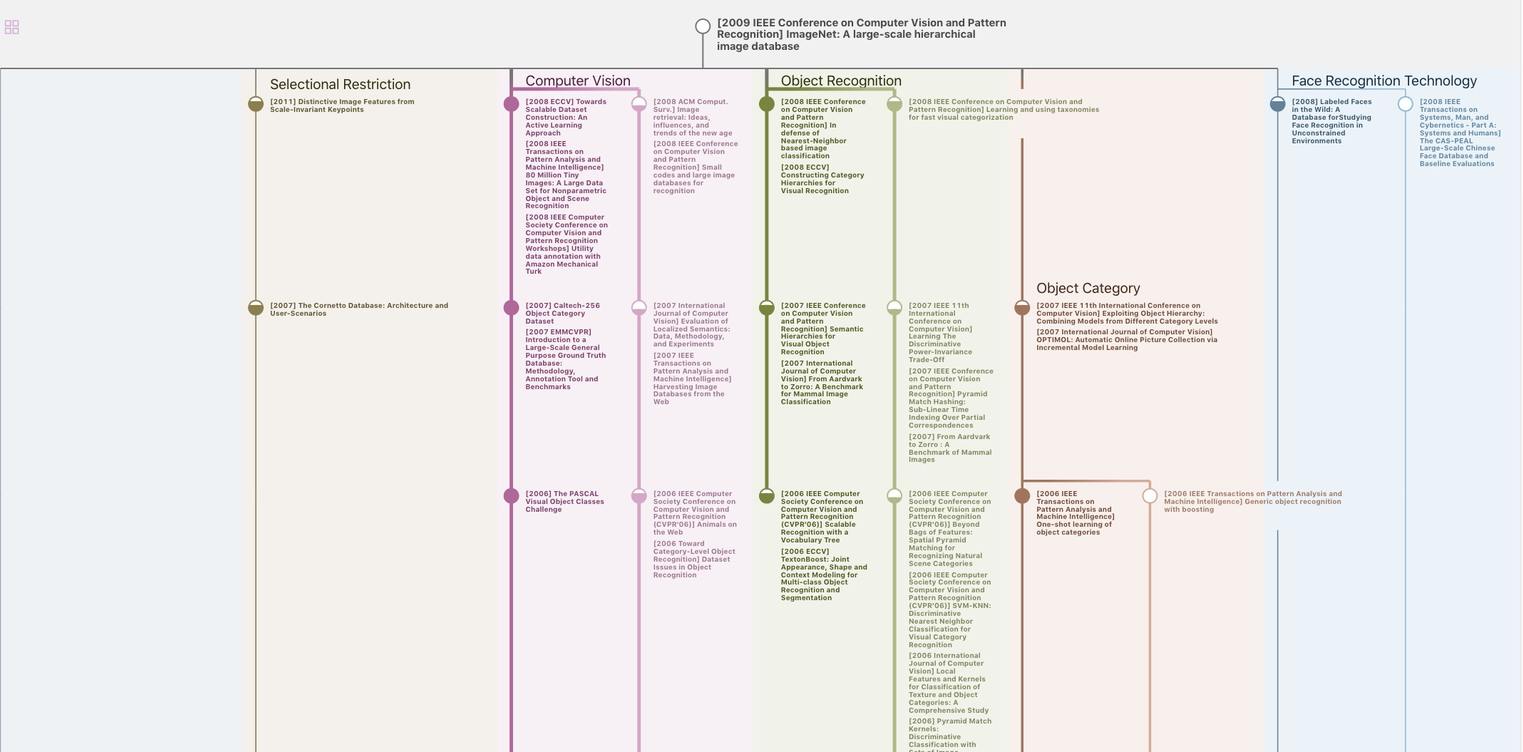
生成溯源树,研究论文发展脉络
Chat Paper
正在生成论文摘要