Faster Fair Machine via Transferring Fairness Constraints to Virtual Samples.
AAAI(2023)
摘要
Fair classification is an emerging and important research topic in machine learning community. Existing methods usually formulate the fairness metrics as additional inequality constraints, and then embed them into the original objective. This makes fair classification problems unable to be effectively tackled by some solvers specific to unconstrained optimization. Although many new tailored algorithms have been designed to attempt to overcome this limitation, they often increase additional computation burden and cannot cope with all types of fairness metrics. To address these challenging issues, in this paper, we propose a novel method for fair classification. Specifically, we theoretically demonstrate that all types of fairness with linear and non-linear covariance functions can be transferred to two virtual samples, which makes the existing state-of-the-art classification solvers be applicable to these cases. Meanwhile, we generalize the proposed method to multiple fairness constraints. We take SVM as an example to show the effectiveness of our new idea. Empirically, we test the proposed method on real-world datasets and all results confirm its excellent performance.
更多查看译文
关键词
fairness constraints,faster fair machine,virtual samples
AI 理解论文
溯源树
样例
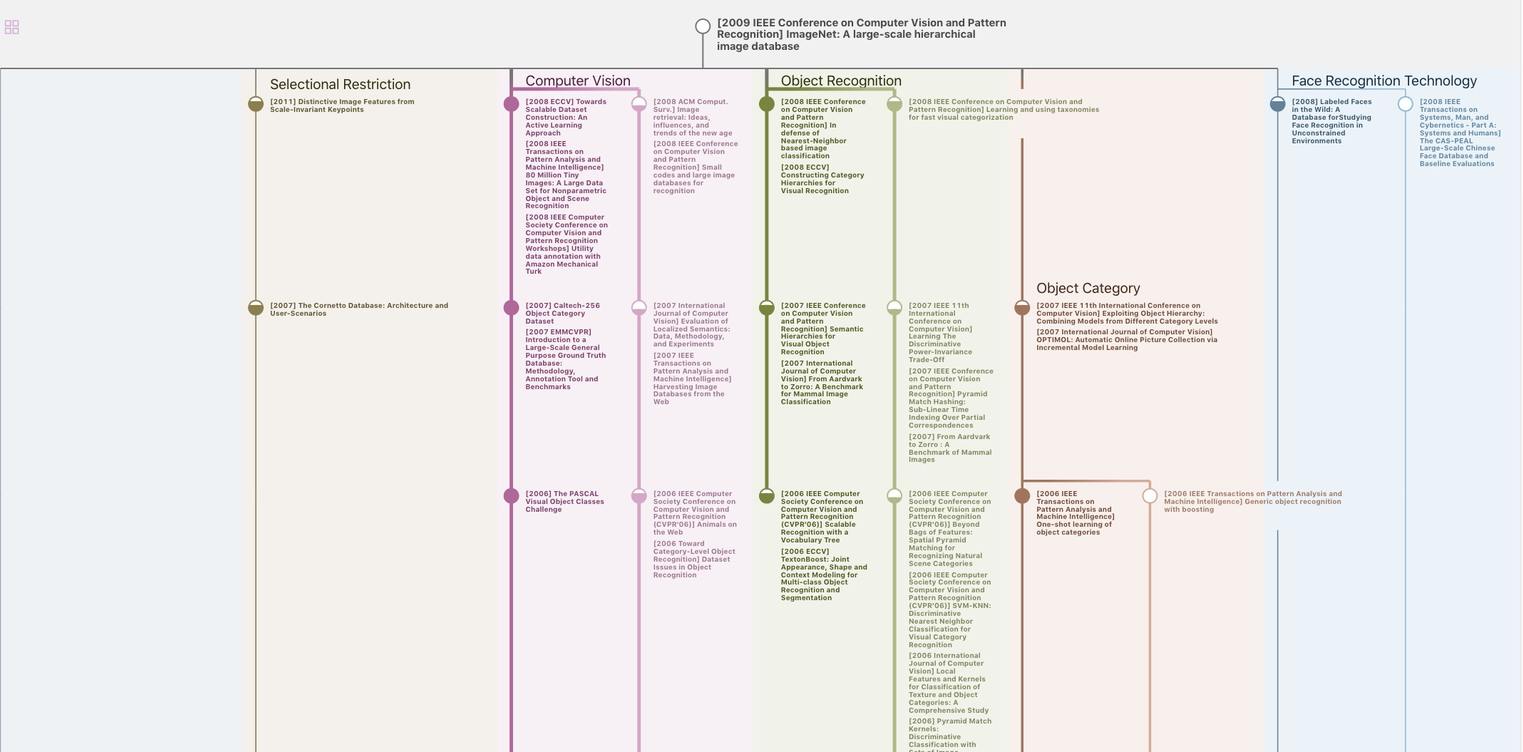
生成溯源树,研究论文发展脉络
Chat Paper
正在生成论文摘要