Learning Generalizable Batch Active Learning Strategies via Deep Q-networks (Student Abstract).
AAAI(2023)
摘要
To handle a large amount of unlabeled data, batch active learning (BAL) queries humans for the labels of a batch of the most valuable data points at every round. Most current BAL strategies are based on human-designed heuristics, such as uncertainty sampling or mutual information maximization. However, there exists a disagreement between these heuristics and the ultimate goal of BAL, i.e., optimizing the model's final performance within the query budgets. This disagreement leads to a limited generality of these heuristics. To this end, we formulate BAL as an MDP and propose a data-driven approach based on deep reinforcement learning. Our method learns the BAL strategy by maximizing the model's final performance. Experiments on the UCI benchmark show that our method can achieve competitive performance compared to existing heuristics-based approaches.
更多查看译文
关键词
active learning,batch,strategies,q-networks
AI 理解论文
溯源树
样例
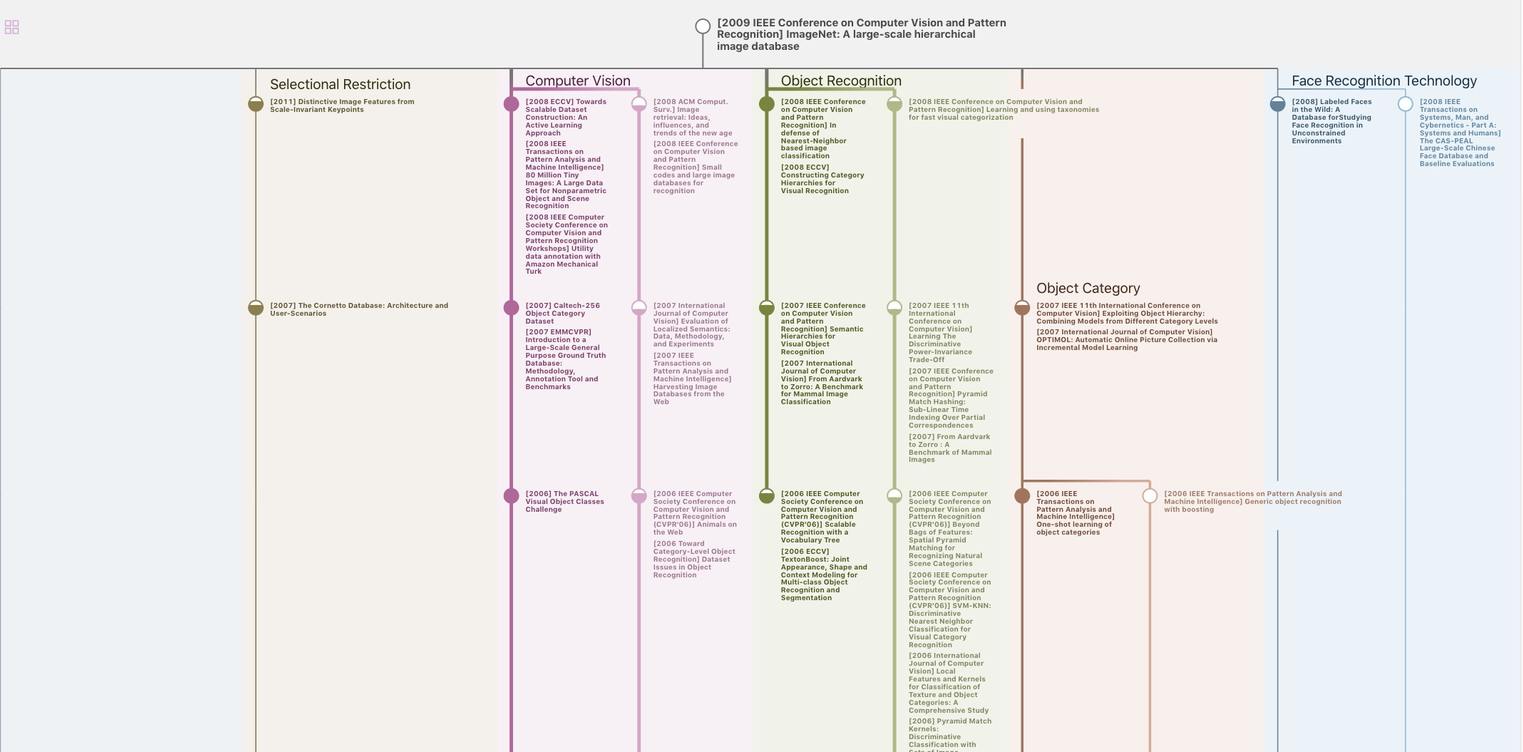
生成溯源树,研究论文发展脉络
Chat Paper
正在生成论文摘要