Iteratively Enhanced Semidefinite Relaxations for Efficient Neural Network Verification.
AAAI(2023)
摘要
We propose an enhanced semidefinite program (SDP) relaxation to enable the tight and efficient verification of neural networks (NNs). The tightness improvement is achieved by introducing a nonlinear constraint to existing SDP relaxations previously proposed for NN verification. The efficiency of the proposal stems from the iterative nature of the proposed algorithm in that it solves the resulting non-convex SDP by recursively solving auxiliary convex layer-based SDP problems. We show formally that the solution generated by our algorithm is tighter than state-of-the-art SDP-based solutions for the problem. We also show that the solution sequence converges to the optimal solution of the non-convex enhanced SDP relaxation. The experimental results on standard benchmarks in the area show that our algorithm achieves the state-of-the-art performance whilst maintaining an acceptable computational cost.
更多查看译文
关键词
efficient neural network verification,semidefinite relaxations
AI 理解论文
溯源树
样例
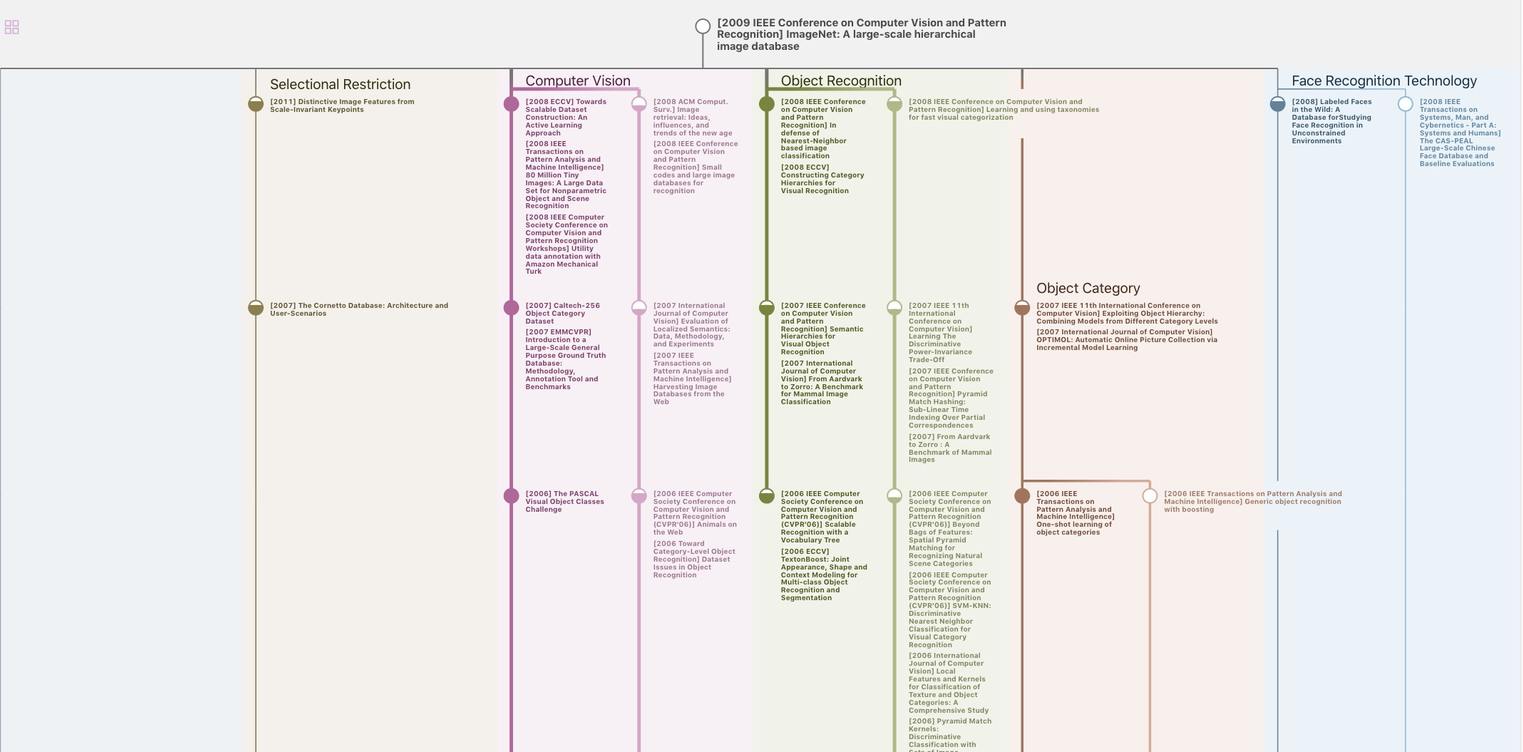
生成溯源树,研究论文发展脉络
Chat Paper
正在生成论文摘要