Self-Paced Learning Based Graph Convolutional Neural Network for Mixed Integer Programming (Student Abstract).
AAAI(2023)
摘要
Graph convolutional neural network (GCN) based methods have achieved noticeable performance in solving mixed integer programming problems (MIPs). However, the generalization of existing work is limited due to the problem structure. This paper proposes a self-paced learning (SPL) based GCN network (SPGCN) with curriculum learning (CL) to make the utmost of samples. SPGCN employs a GCN model to imitate the branching variable selection during the branch and bound process, while the training process is conducted in a self-paced fashion. Specifically, SPGCN contains a loss-based automatic difficulty measurer, where the training loss of the sample represents the difficulty level. In each iteration, a dynamic training dataset is constructed according to the difficulty level for GCN model training. Experiments on four NP-hard datasets verify that CL can lead to generalization improvement and convergence speedup in solving MIPs, where SPL performs better than predefined CL methods.
更多查看译文
关键词
mixed integer programming,graph convolutional neural network,convolutional neural network,student abstract,self-paced
AI 理解论文
溯源树
样例
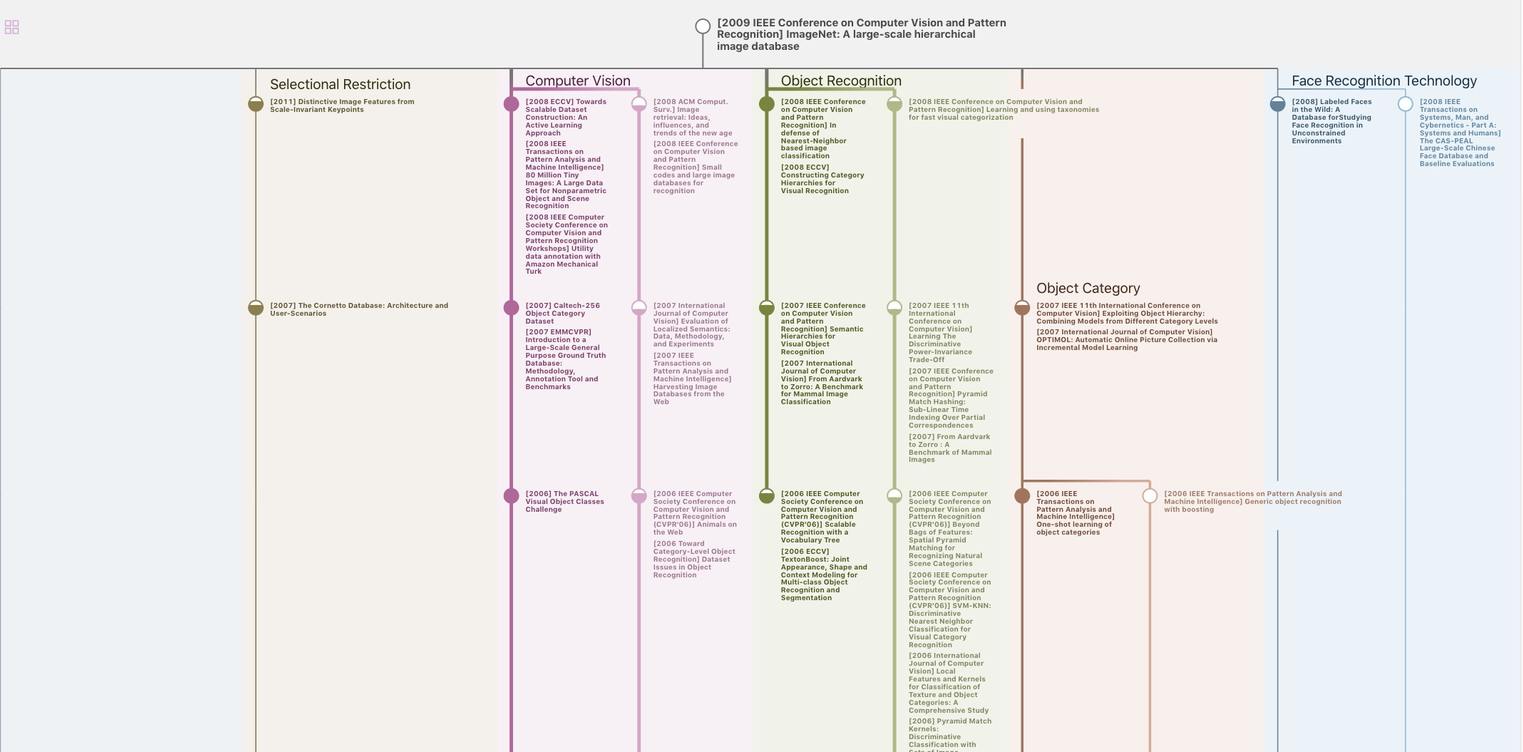
生成溯源树,研究论文发展脉络
Chat Paper
正在生成论文摘要