Spatial–Temporal gated graph attention network for skeleton-based action recognition
Pattern Anal. Appl.(2023)
摘要
Human body skeleton, acting as a spatiotemporal graph, is the key inspiration for researchers to implement a GCN-based method for action recognition. Most recently proposed GCN-based techniques combine convolutions with a self-attention mechanism to extract the most informative joint of a human skeleton and increase the model accuracy. However, the traditional multi-head attention method absorbed all attention heads equally, whereas in most situations, especially for extended skeleton sequences, not all attention heads are expected. Therefore, we present a spatial–temporal gated graph attention network (ST-GGANet) to learn the spatial–temporal patterns of skeleton sequences. The proposed approach uses a lightweight self-attention-based gate layer to pay attention to the important body parts or joints of human skeleton sequences. Experiments show that by introducing an additional gate layer, which controls the significance of each attention head, the proposed model achieved better action classification results while keeping computational cost low. The proposed framework has been evaluated on well-known publicly available large-scale datasets NTU60, NTU120, and Kinetics-400, which notably outperforms state-of-the-art (SOTA) results with an accuracy of 96.5%, 87.3%, and 60.4%, respectively.
更多查看译文
关键词
Action recognition,Graph attention network,Gated self-attention
AI 理解论文
溯源树
样例
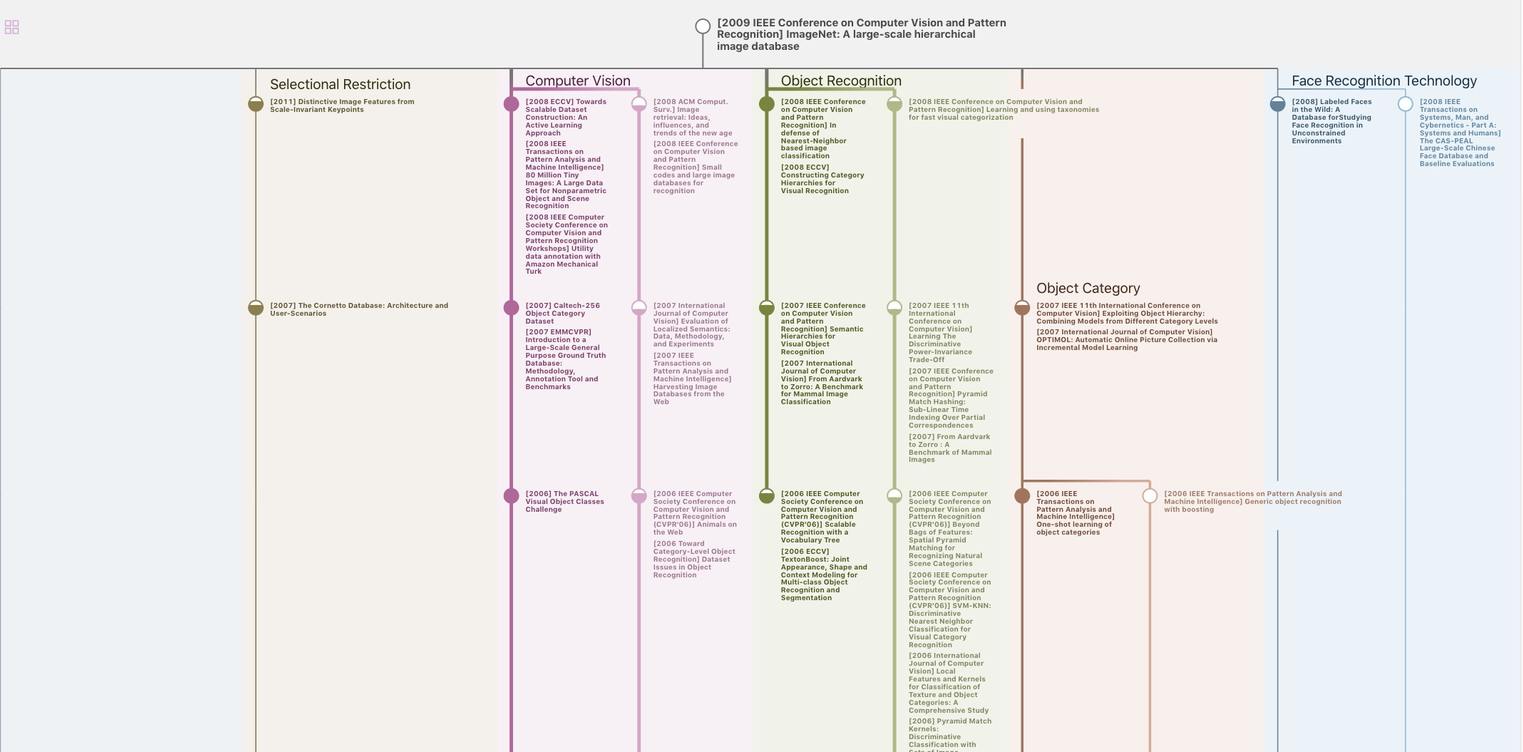
生成溯源树,研究论文发展脉络
Chat Paper
正在生成论文摘要