Stochastic smoothing accelerated gradient method for nonsmooth convex composite optimization
arXiv (Cornell University)(2023)
摘要
We propose a novel stochastic smoothing accelerated gradient (SSAG) method for general constrained nonsmooth convex composite optimization, and analyze the convergence rates. The SSAG method allows various smoothing techniques, and can deal with the nonsmooth term that is not easy to compute its proximal term, or that does not own the linear max structure. To the best of our knowledge, it is the first stochastic approximation type method with solid convergence result to solve the convex composite optimization problem whose nonsmooth term is the maximization of numerous nonlinear convex functions. We prove that the SSAG method achieves the best-known complexity bounds in terms of the stochastic first-order oracle ($\mathcal{SFO}$), using either diminishing smoothing parameters or a fixed smoothing parameter. We give two applications of our results to distributionally robust optimization problems. Numerical results on the two applications demonstrate the effectiveness and efficiency of the proposed SSAG method.
更多查看译文
关键词
stochastic smoothing,gradient method,optimization
AI 理解论文
溯源树
样例
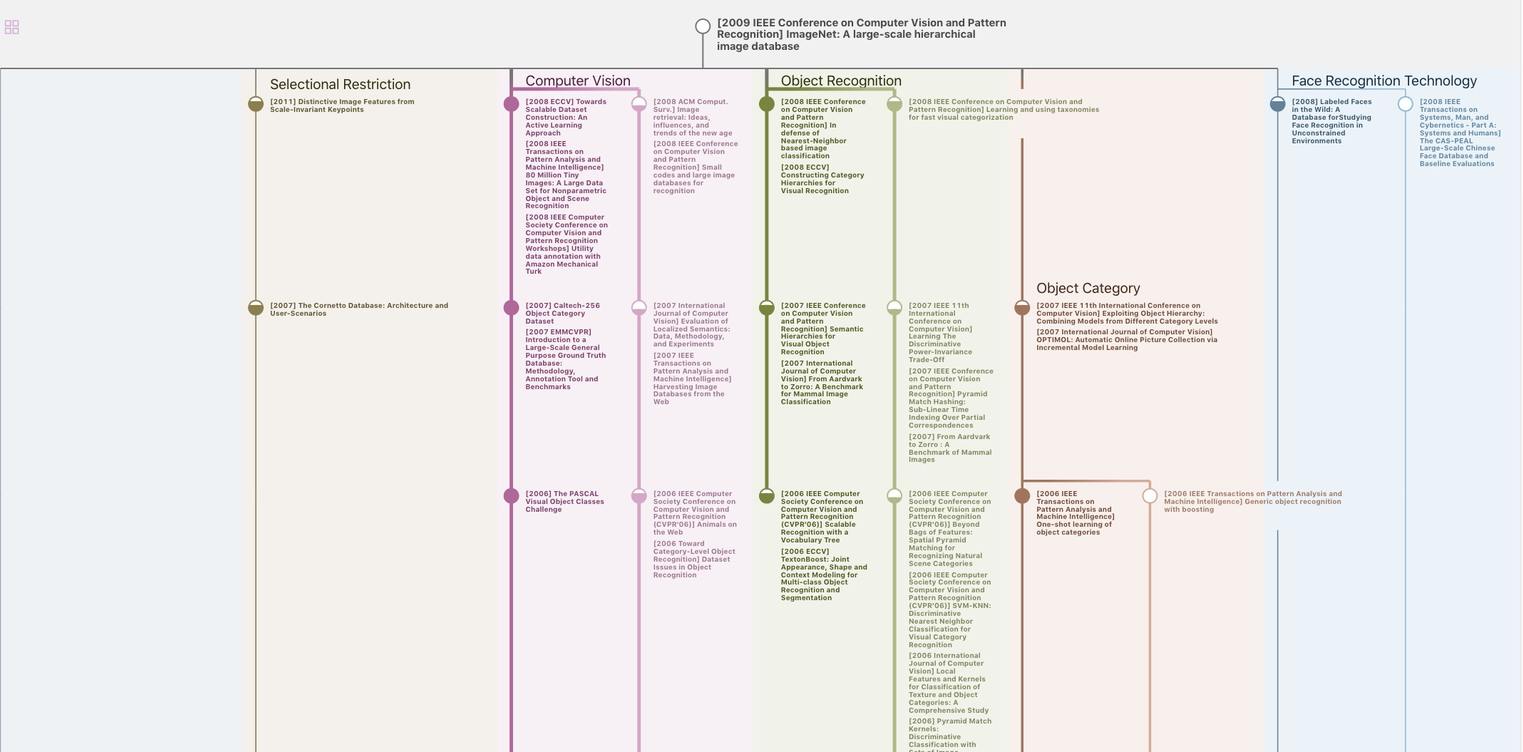
生成溯源树,研究论文发展脉络
Chat Paper
正在生成论文摘要