Unlearning Spurious Correlations in Chest X-ray Classification
CoRR(2023)
摘要
Medical image classification models are frequently trained using training datasets derived from multiple data sources. While leveraging multiple data sources is crucial for achieving model generalization, it is important to acknowledge that the diverse nature of these sources inherently introduces unintended confounders and other challenges that can impact both model accuracy and transparency. A notable confounding factor in medical image classification, particularly in musculoskeletal image classification, is skeletal maturation-induced bone growth observed during adolescence. We train a deep learning model using a Covid-19 chest X-ray dataset and we showcase how this dataset can lead to spurious correlations due to unintended confounding regions. eXplanation Based Learning (XBL) is a deep learning approach that goes beyond interpretability by utilizing model explanations to interactively unlearn spurious correlations. This is achieved by integrating interactive user feedback, specifically feature annotations. In our study, we employed two non-demanding manual feedback mechanisms to implement an XBL-based approach for effectively eliminating these spurious correlations. Our results underscore the promising potential of XBL in constructing robust models even in the presence of confounding factors.
更多查看译文
关键词
spurious correlations,chest,classification,x-ray
AI 理解论文
溯源树
样例
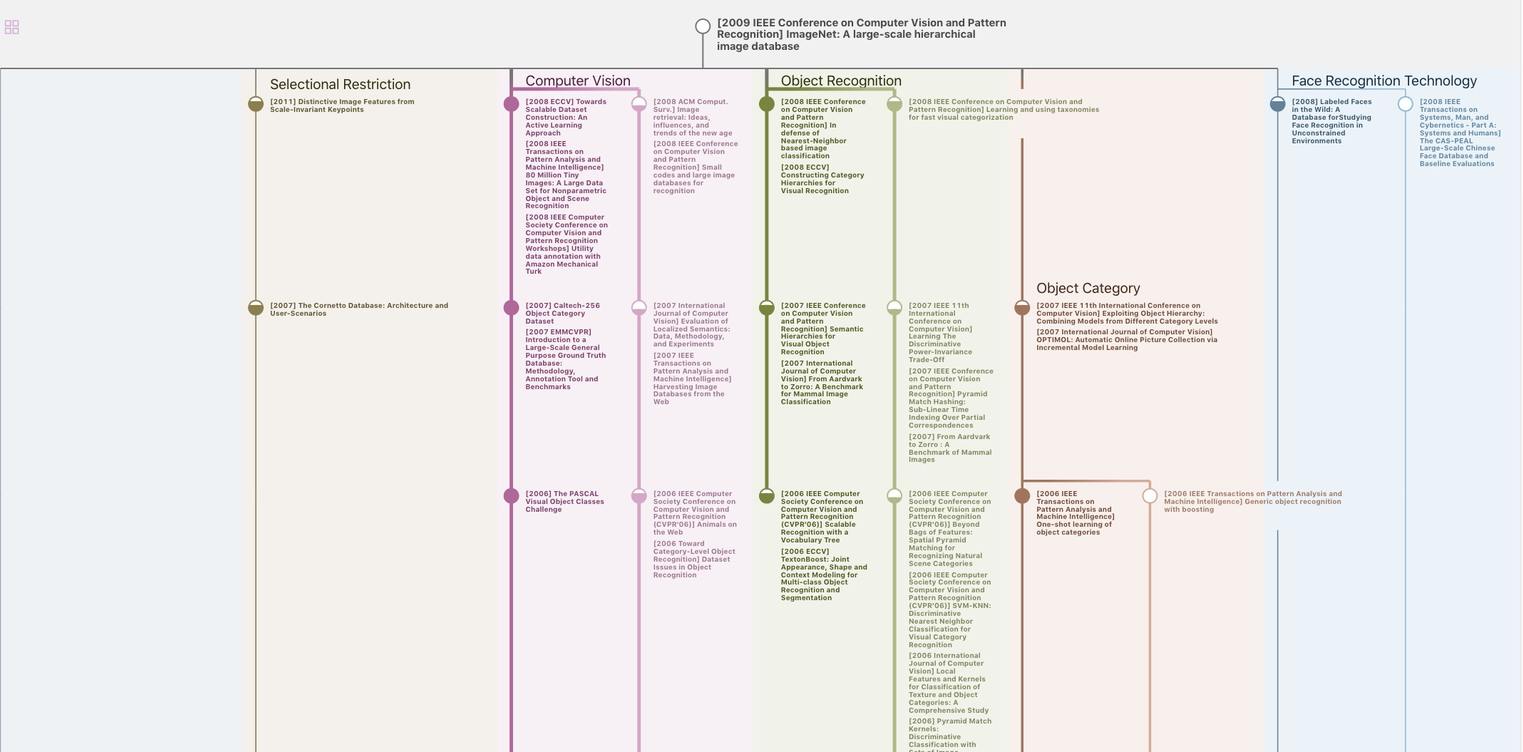
生成溯源树,研究论文发展脉络
Chat Paper
正在生成论文摘要