Causal Inference with Differentially Private (Clustered) Outcomes
CoRR(2023)
摘要
Estimating causal effects from randomized experiments is only feasible if participants agree to reveal their potentially sensitive responses. Of the many ways of ensuring privacy, label differential privacy is a widely used measure of an algorithm's privacy guarantee, which might encourage participants to share responses without running the risk of de-anonymization. Many differentially private mechanisms inject noise into the original data-set to achieve this privacy guarantee, which increases the variance of most statistical estimators and makes the precise measurement of causal effects difficult: there exists a fundamental privacy-variance trade-off to performing causal analyses from differentially private data. With the aim of achieving lower variance for stronger privacy guarantees, we suggest a new differential privacy mechanism, "Cluster-DP", which leverages any given cluster structure of the data while still allowing for the estimation of causal effects. We show that, depending on an intuitive measure of cluster quality, we can improve the variance loss while maintaining our privacy guarantees. We compare its performance, theoretically and empirically, to that of its unclustered version and a more extreme uniform-prior version which does not use any of the original response distribution, both of which are special cases of the "Cluster-DP" algorithm.
更多查看译文
关键词
inference,outcomes,clustered
AI 理解论文
溯源树
样例
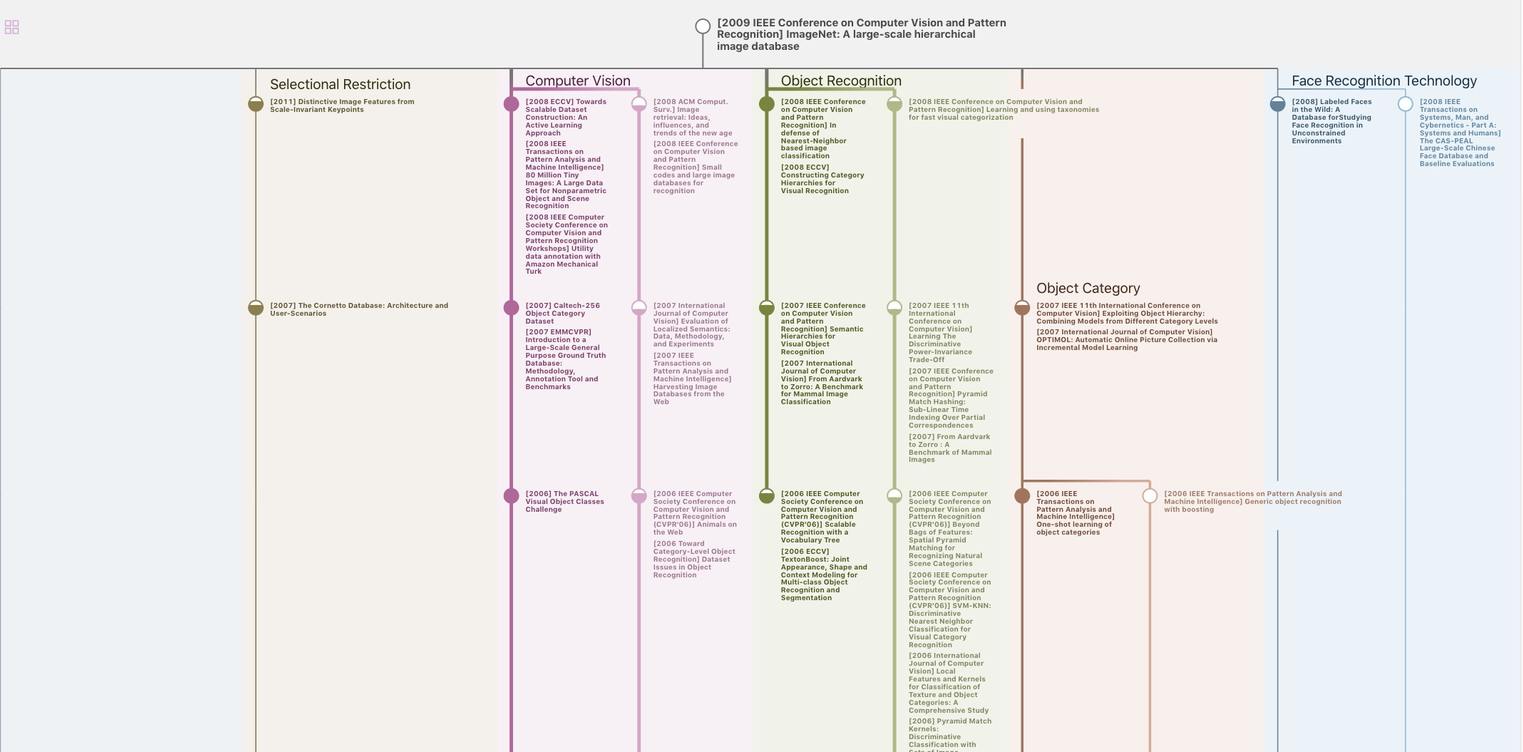
生成溯源树,研究论文发展脉络
Chat Paper
正在生成论文摘要