Machine-Learning-Aided NO2 Discrimination with an Array of Graphene Chemiresistors Covalently Functionalized by Diazonium Chemistry
Chemistry (Weinheim an der Bergstrasse, Germany)(2023)
摘要
Boosted by the emerging need for highly integrated gas sensors in the internet of things (IoT) ecosystems, electronic noses (e-noses) are gaining interest for the detection of specific molecules over a background of interfering gases. The sensing of nitrogen dioxide is particularly relevant for applications in environmental monitoring and precision medicine. Here we present an easy and efficient functionalization procedure to covalently modify graphene layers, taking advantage of diazonium chemistry. Separate graphene layers were functionalized with one of three different aryl rings: 4-nitrophenyl, 4-carboxyphenyl and 4-bromophenyl. The distinct modified graphene layers were assembled with a pristine layer into an e-nose for NO2 discrimination. A remarkable sensitivity to NO2 was demonstrated through exposure to gaseous solutions with NO2 concentrations in the 1-10 ppm range at room temperature. Then, the discrimination capability of the sensor array was tested by carrying out exposure to several interfering gases and analyzing the data through multivariate statistical analysis. This analysis showed that the e-nose can discriminate NO2 among all the interfering gases in a two-dimensional principal component analysis space. Finally, the e-nose was trained to accurately recognize NO2 contributions with a linear discriminant analysis approach, thus providing a metric for discrimination assessment with a prediction accuracy above 95 %.
更多查看译文
关键词
chemiresistors,covalent functionalization of graphene,e-nose,machine learning,nitrogen dioxide
AI 理解论文
溯源树
样例
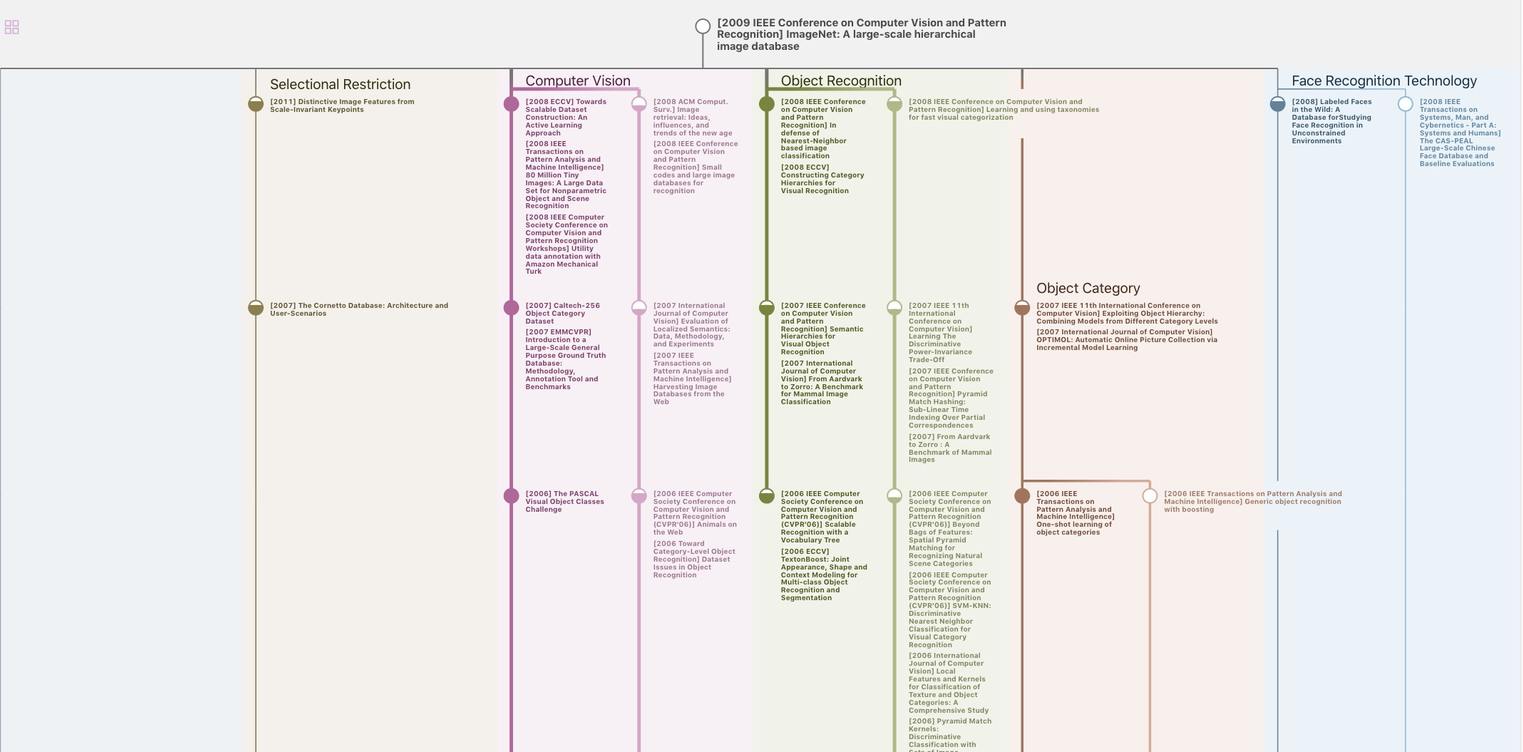
生成溯源树,研究论文发展脉络
Chat Paper
正在生成论文摘要