AN L2-NORMALIZED SPATIAL ATTENTION NETWORK FOR ACCURATE AND FAST CLASSIFICATION OF BRAIN TUMORS IN 2D T1-WEIGHTED CE-MRI IMAGES
2023 IEEE INTERNATIONAL CONFERENCE ON IMAGE PROCESSING, ICIP(2023)
摘要
We propose an accurate and fast classification network for classification of brain tumors in MRI images that outperforms all lightweight methods investigated in terms of accuracy. We test our model on a challenging 2D T1-weighted CE-MRI dataset containing three types of brain tumors: Meningioma, Glioma and Pituitary. We introduce an l(2)-normalized spatial attention mechanism that acts as a regularizer against overfitting during training. We compare our results against the state-of-the-art on this dataset and show that by integrating l(2)-normalized spatial attention into a baseline network we achieve a performance gain of 1.79 percentage points. Even better accuracy can be attained by combining our model in an ensemble with the pretrained VGG16 at the expense of execution speed. Our code is publicly available at https://github.com/juliadietlmeier/MRI_image_classification.
更多查看译文
关键词
Deep learning,MRI image analysis,attention mechanisms,brain tumor classification
AI 理解论文
溯源树
样例
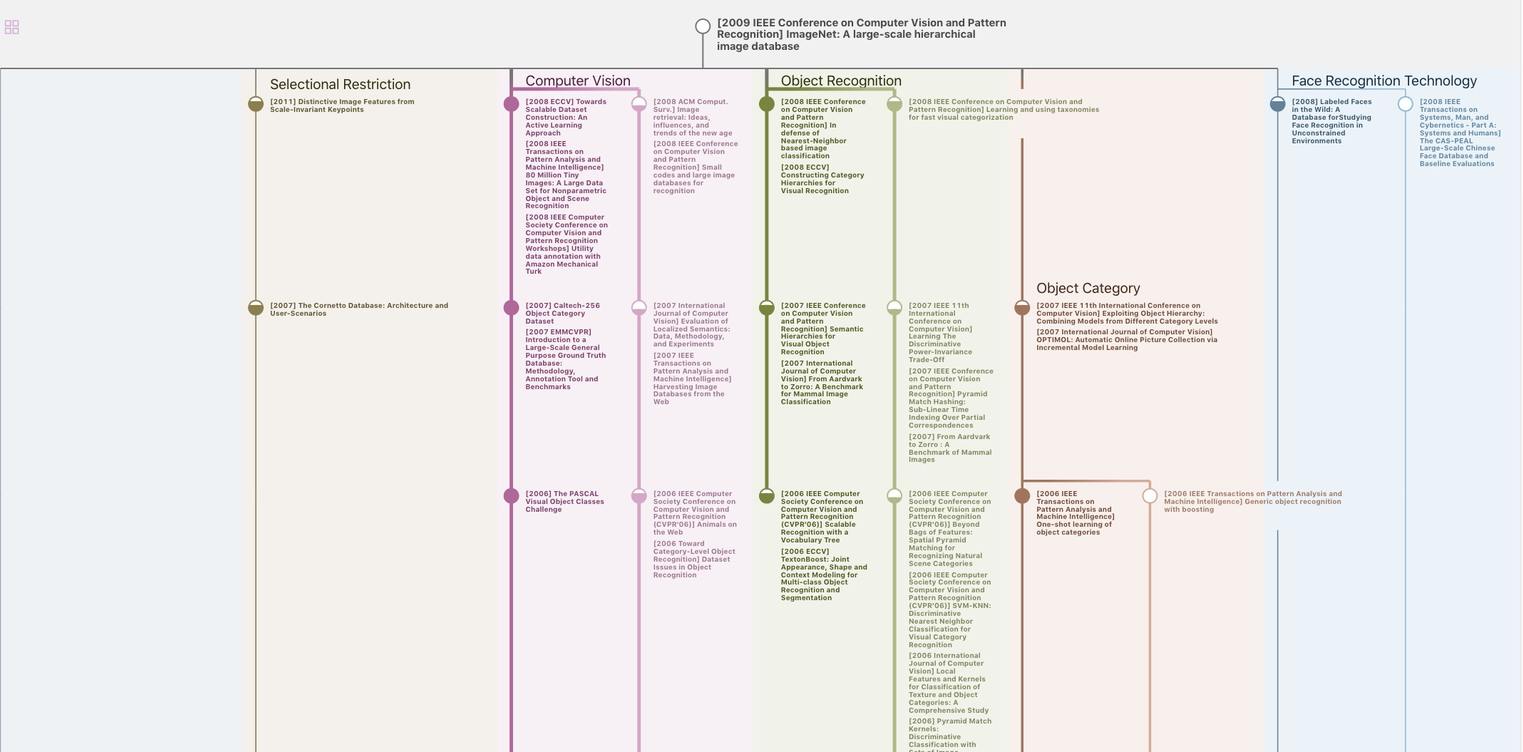
生成溯源树,研究论文发展脉络
Chat Paper
正在生成论文摘要