Space Debris: Are Deep Learning-based Image Enhancements part of the Solution?
CoRR(2023)
摘要
The volume of space debris currently orbiting the Earth is reaching an unsustainable level at an accelerated pace. The detection, tracking, identification, and differentiation between orbit-defined, registered spacecraft, and rogue/inactive space ``objects'', is critical to asset protection. The primary objective of this work is to investigate the validity of Deep Neural Network (DNN) solutions to overcome the limitations and image artefacts most prevalent when captured with monocular cameras in the visible light spectrum. In this work, a hybrid UNet-ResNet34 Deep Learning (DL) architecture pre-trained on the ImageNet dataset, is developed. Image degradations addressed include blurring, exposure issues, poor contrast, and noise. The shortage of space-generated data suitable for supervised DL is also addressed. A visual comparison between the URes34P model developed in this work and the existing state of the art in deep learning image enhancement methods, relevant to images captured in space, is presented. Based upon visual inspection, it is determined that our UNet model is capable of correcting for space-related image degradations and merits further investigation to reduce its computational complexity.
更多查看译文
关键词
image enhancements part,space,learning-based
AI 理解论文
溯源树
样例
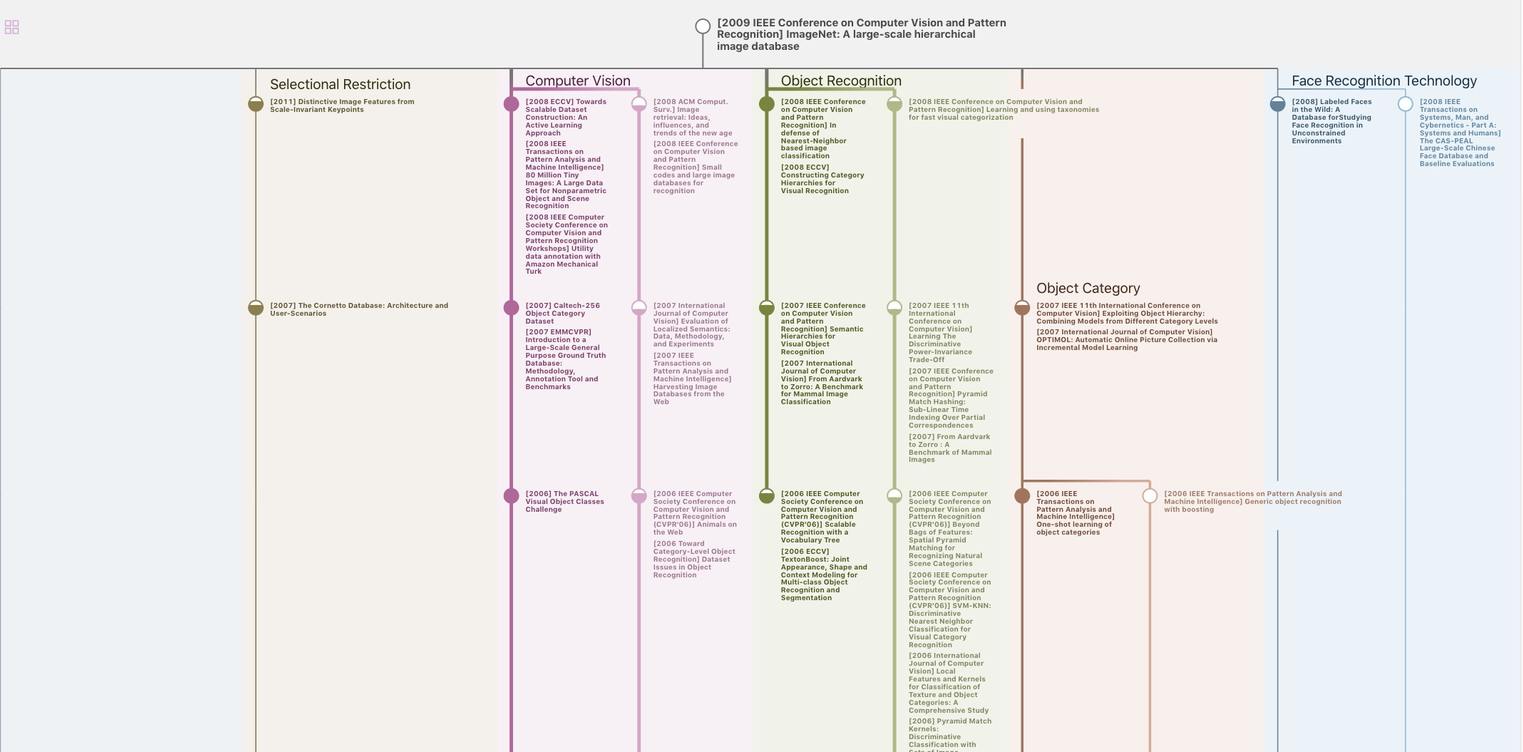
生成溯源树,研究论文发展脉络
Chat Paper
正在生成论文摘要