Pretrained deep models outperform GBDTs in Learning-To-Rank under label scarcity
CoRR(2023)
摘要
On tabular data, a significant body of literature has shown that current deep
learning (DL) models perform at best similarly to Gradient Boosted Decision
Trees (GBDTs), while significantly underperforming them on outlier data. We
identify a natural tabular data setting where DL models can outperform GBDTs:
tabular Learning-to-Rank (LTR) under label scarcity. Tabular LTR applications,
including search and recommendation, often have an abundance of unlabeled data,
and scarce labeled data. We show that DL rankers can utilize unsupervised
pretraining to exploit this unlabeled data. In extensive experiments over both
public and proprietary datasets, we show that pretrained DL rankers
consistently outperform GBDT rankers on ranking metrics – sometimes by as much
as 38% – both overall and on outliers.
更多查看译文
关键词
gbdts,deep models,label
AI 理解论文
溯源树
样例
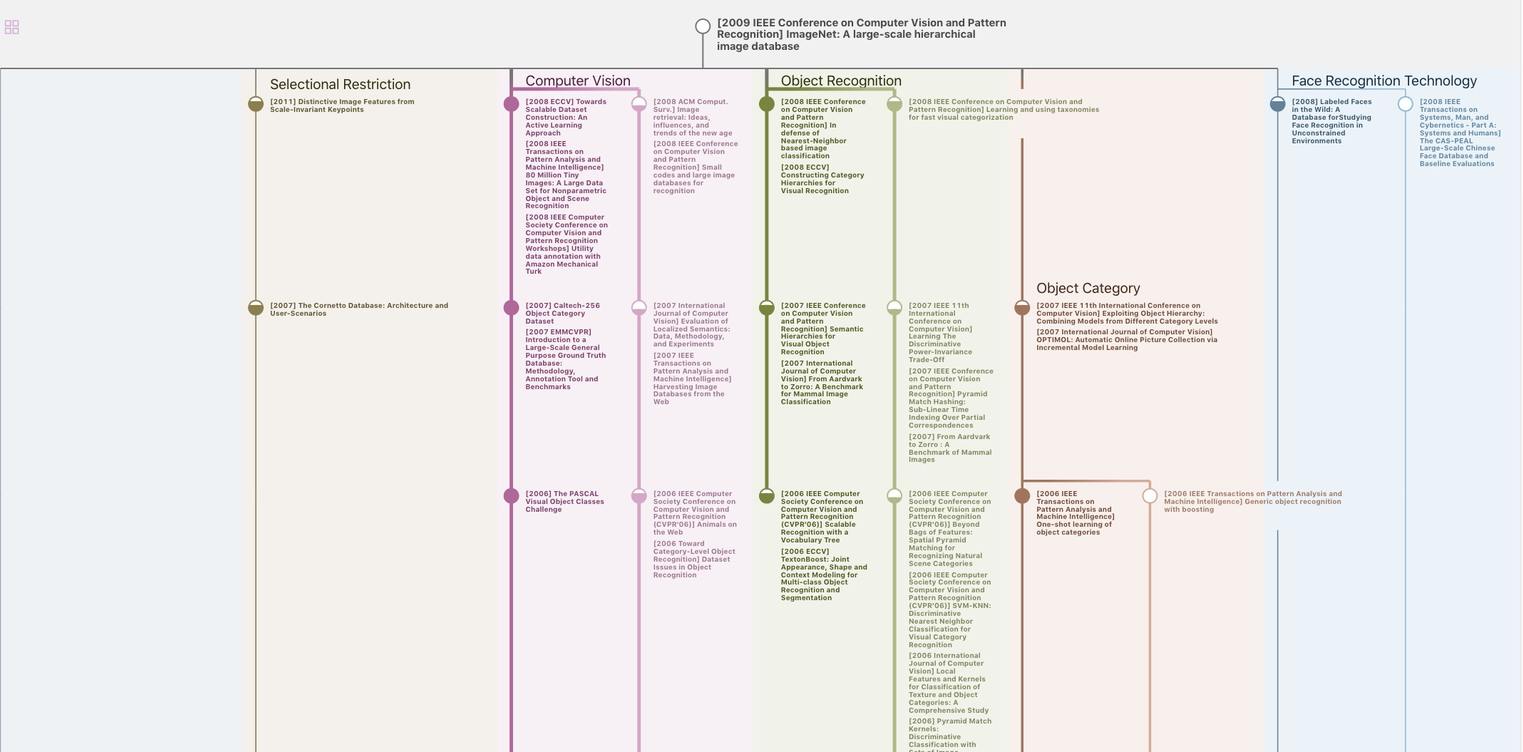
生成溯源树,研究论文发展脉络
Chat Paper
正在生成论文摘要